Human vs. Machine: Behavioral Differences Between Expert Humans and Language Models in Wargame Simulations
CoRR(2024)
摘要
To some, the advent of artificial intelligence (AI) promises better
decision-making and increased military effectiveness while reducing the
influence of human error and emotions. However, there is still debate about how
AI systems, especially large language models (LLMs), behave compared to humans
in high-stakes military decision-making scenarios with the potential for
increased risks towards escalation and unnecessary conflicts. To test this
potential and scrutinize the use of LLMs for such purposes, we use a new
wargame experiment with 107 national security experts designed to look at
crisis escalation in a fictional US-China scenario and compare human players to
LLM-simulated responses in separate simulations. Wargames have a long history
in the development of military strategy and the response of nations to threats
or attacks. Here, we show a considerable high-level agreement in the LLM and
human responses and significant quantitative and qualitative differences in
individual actions and strategic tendencies. These differences depend on
intrinsic biases in LLMs regarding the appropriate level of violence following
strategic instructions, the choice of LLM, and whether the LLMs are tasked to
decide for a team of players directly or first to simulate dialog between
players. When simulating the dialog, the discussions lack quality and maintain
a farcical harmony. The LLM simulations cannot account for human player
characteristics, showing no significant difference even for extreme traits,
such as "pacifist" or "aggressive sociopath". Our results motivate policymakers
to be cautious before granting autonomy or following AI-based strategy
recommendations.
更多查看译文
AI 理解论文
溯源树
样例
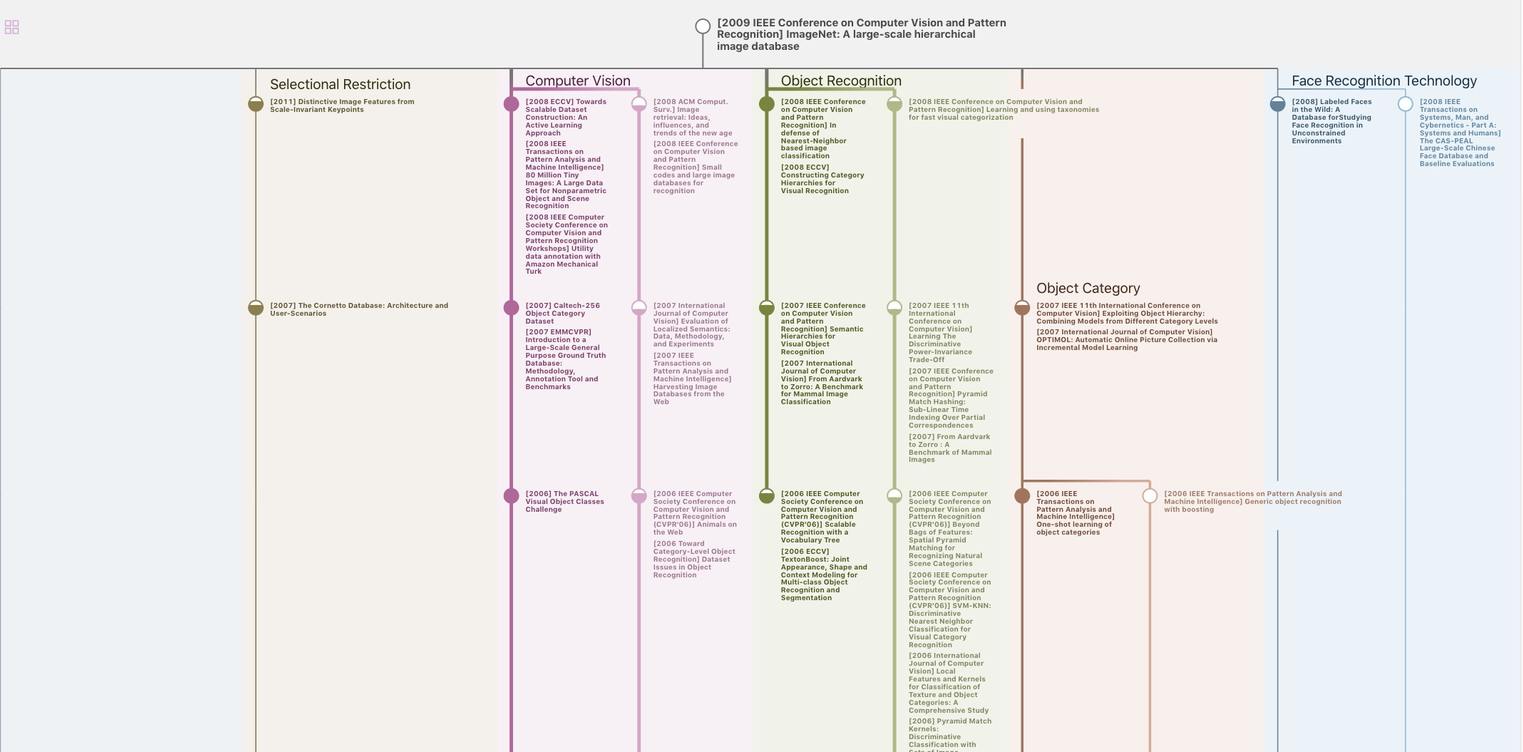
生成溯源树,研究论文发展脉络
Chat Paper
正在生成论文摘要