Comparative Analysis Between Bi-LSTM and Uni-LSTM Algorithms for Wind Speed Estimation
2023 7th International Conference on Computer Applications in Electrical Engineering-Recent Advances (CERA)(2023)
摘要
The integration of sustainable energy sources into the conventional power grid depends on accurate wind speed estimates since it affects grid operations, stability, and overall planning. Owing to its advantages for the environment and ability to lessen dependency on fossil fuels, wind power is highly valued. Accurate wind speed prediction enables grid managers to estimate energy generation and make in-the-moment changes to ensure a steady and dependable power supply. It is becoming increasingly important to comprehend and utilize wind power’s potential as the globe moves toward a more sustainable energy future. The use of deep learning algorithms like Unilateral Long Short-Term Memory (Uni-LSTM), Bilateral Long Short-Term Memory (Bi-LSTM), and Gated Recurrent Unit (GRU) networks is one of the novel ways being investigated in research to improve wind speed forecasts. These algorithms examine past wind speed data and forecast future wind speeds by utilizing their innate memory and flexibility. This study focuses on the Jaisalmer area in Rajasthan, India, where the energy mix may be considerably influenced by wind power. To ensure the data is properly scaled for input into the deep learning models, a preprocessing step is conducted using the Min-Max scaler approach on a dataset downloaded from the NASA website. The analysis shows that the GRU model outperforms the Uni-LSTM and Bi-LSTM models. The “RELU” activation function is used in these models. The model’s performance is analyzed by comparing MAE and RMSE error values with $R^{2}$ accuracy values. The GRU model gives rich $R^{2}$ value performances better than other models.
更多查看译文
关键词
Uni-LSTM,Bi-LSTM,GRU,Estimation,Wind speed,Deep learning models
AI 理解论文
溯源树
样例
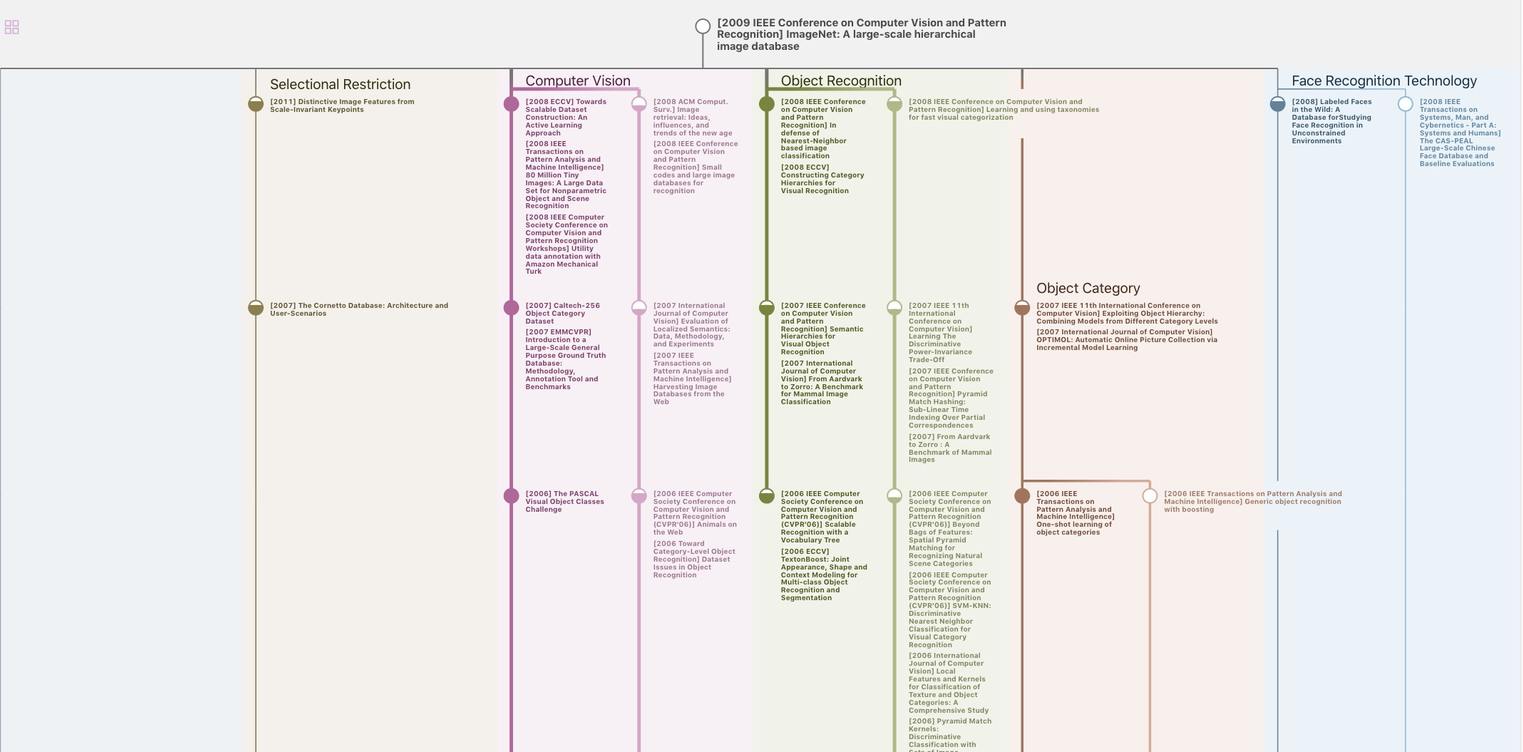
生成溯源树,研究论文发展脉络
Chat Paper
正在生成论文摘要