Activating high-frequency information nodes for super-resolution magnetic resonance imaging
BIOMEDICAL SIGNAL PROCESSING AND CONTROL(2024)
摘要
Objective: To recover the missing high-frequency components in low-resolution images by activating nodes in the high-frequency region of k-space. In addition, combining the information features in the k-space domain with the structural features in the image domain to generate high-quality super-resolution images. Methods: We propose a novel Dual-Domain cascaded Super -Resolution Network (DDSRNet), which shifts the starting point of the network to k-space. DDSRNet combines the ideas of GraphSAGE, Swin-Unet, and Hybrid Attention Transformer, to achieve complementary advantages of the k-space domain and the image domain. The k-space domain network activates zero-filled nodes in high-frequency regions and generates multi-order information features. The image domain network performs shallow, deep and gradient feature extraction to obtain the high-level representation of dual-domain hybrid features. In addition, to better utilize the features of both domains, we construct a domain interaction pool to facilitate cross-domain feature transfer and improve the efficiency of feature fusion. Results: Exhaustive experiments are conducted on both public dataset and real scanning dataset. Compared with state-of-the-art algorithms, DDSRNet has the best numerical evaluation results (the average PSNR and SSIM improvements are more than 0.1 dB and 0.0078) and visual perception. In addition, DDSRNet enables highquality 2x SR MRI with a reduced number of excitations, leading to increased low-field imaging speed. Conclusion: DDSRNet shows excellent performance in highand low-field SR tasks and has the potential to be a powerful tool for clinical applications. Significance: The proposed method is of great practical significance for achieving fast and high-quality magnetic resonance imaging.
更多查看译文
关键词
MRI,Super-resolution,K-space,Dual-domain,Deep learning
AI 理解论文
溯源树
样例
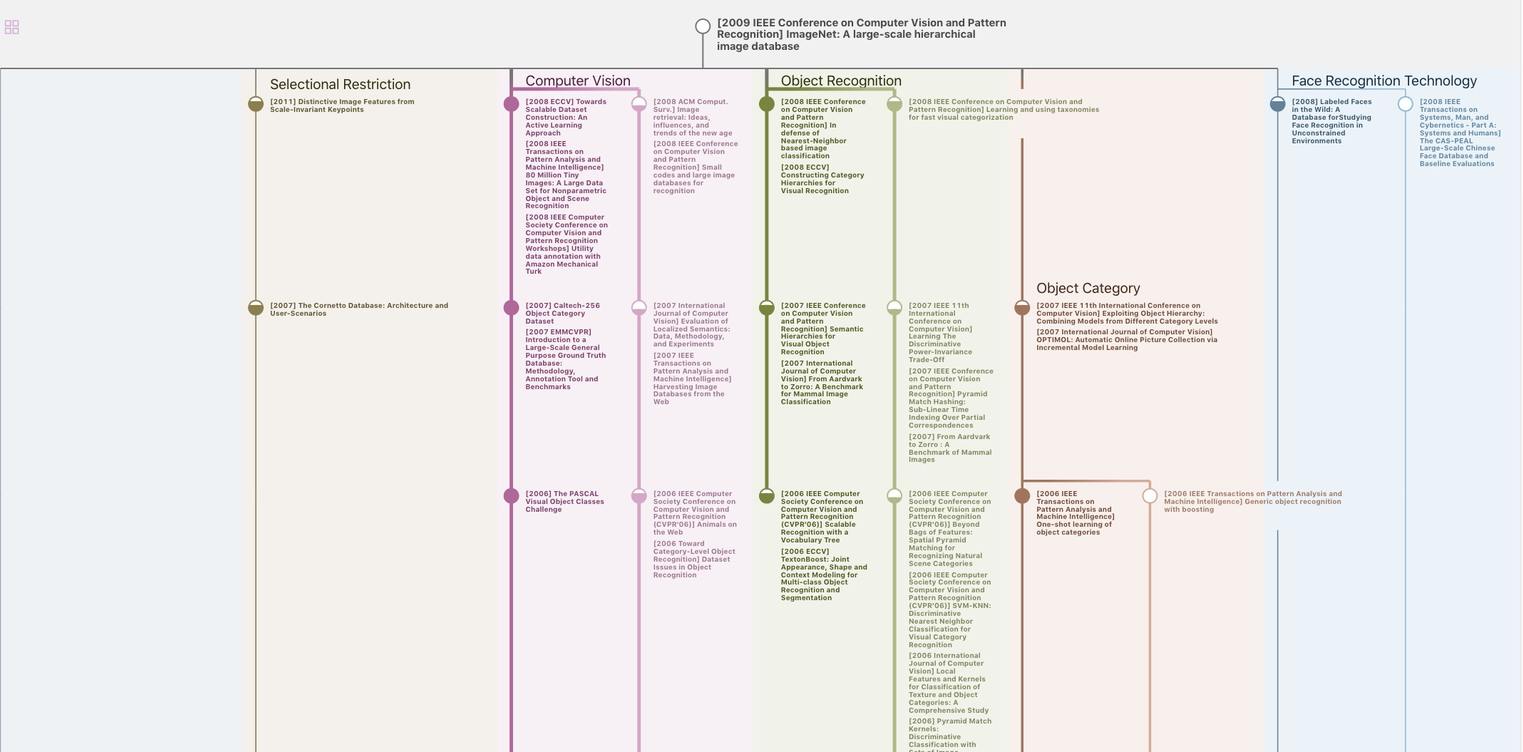
生成溯源树,研究论文发展脉络
Chat Paper
正在生成论文摘要