Wireless Power Assisted Computation Offloading in Mobile Edge Computing: A Deep Reinforcement Learning Approach
HUMAN-CENTRIC COMPUTING AND INFORMATION SCIENCES(2024)
摘要
One of the missions of mobile edge computing (MEC) is to develop innovative applications in closer proximity while preserving latency constraints. Integration of MEC with the emerging wireless power transfer (WPT) approach extends the battery lifetime of end devices. Fading channels with time-varying constraints limit this procedure. We propose a joint deep reinforcement learning based framework that effectively initiates the worthy offloading solution with optimal WPT duration. To solve the joint optimization non-convex problem, we decompose the original problem into two sub-problems including the selection of an efficient offloading solution and allocation of optimal WPT duration. We consider orthogonal frequency division multiple access (OFDMA) as a channel access method. As the hyperparameters of a neural network largely influence its performance, we also simulate and analyze different variations of these parameters for system performance. Furthermore, we also reduce the overhead of task partitioning which usually involves in partial offloading schemes with DNNs. Simulation results show the effectiveness and applicability of the proposed framework in dynamic networks. For example, the proposed framework provides an average execution latency of 372.3 ms per channel in a 30-user network, making the system truly applicable in large scale dynamic networks.
更多查看译文
关键词
Mobile Edge Computing (MEC),Wireless Power Transfer (WPT),Deep Learning,Computation Offloading,Neural Networks,Dynamic Channels
AI 理解论文
溯源树
样例
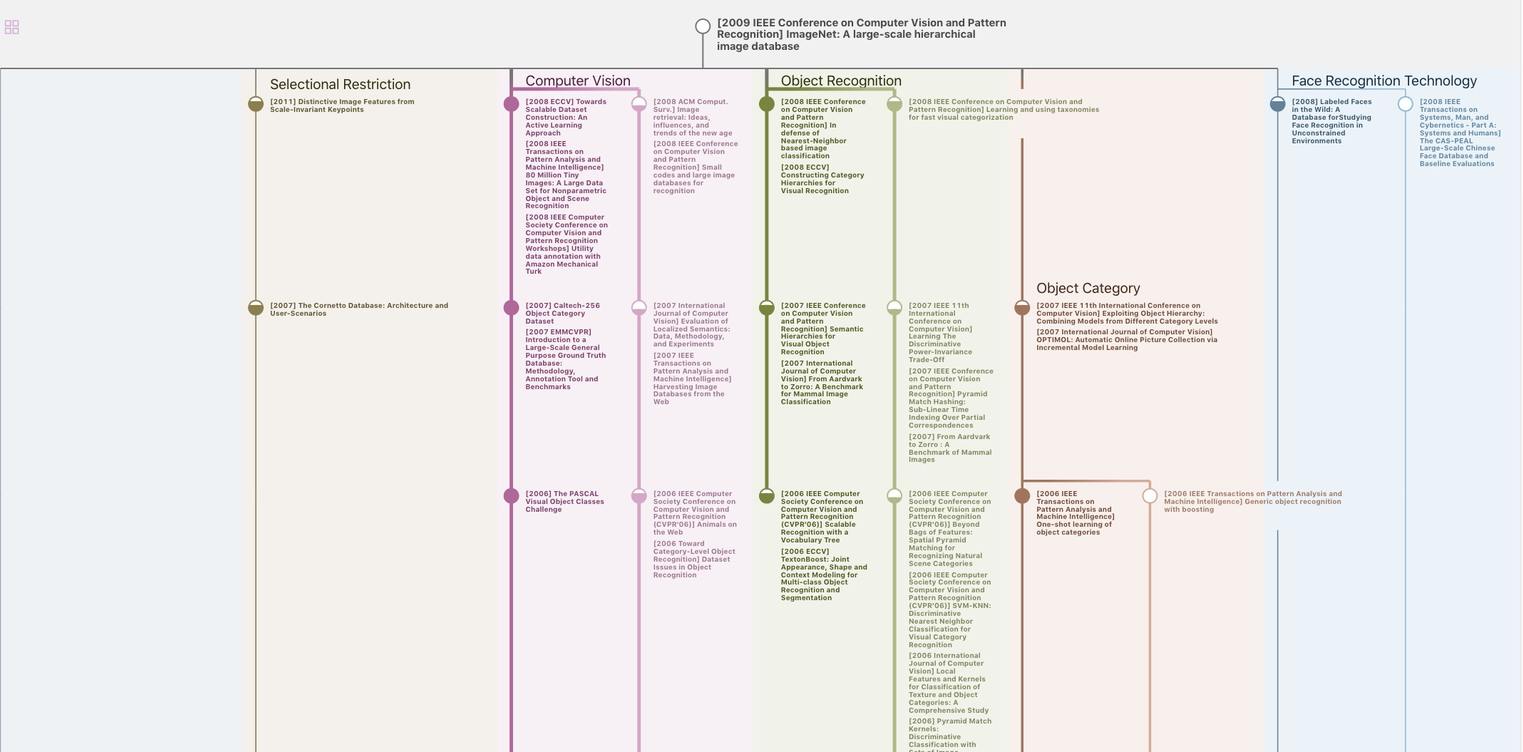
生成溯源树,研究论文发展脉络
Chat Paper
正在生成论文摘要