A multi-label social short text classification method based on contrastive learning and improved ml-KNN
EXPERT SYSTEMS(2024)
摘要
Short texts on social platforms often have the problems of diverse categories and semantic sparsity, making it challenging to identify the diverse intentions of users. To address this issue, this article proposes a multi-label social short text classification method (IML-CL) based on contrastive learning and improved ml-KNN. First, a contrastive learning approach is employed to train a multi-label text classification model. This approach improves semantic sparsity by leveraging the knowledge from the existing samples to enrich the feature representation of short texts. Simultaneously, an improved ml-KNN algorithm is developed to enhance the accuracy of label prediction. This algorithm utilizes a two-layer nearest neighbor rule and introduces a penalty function and weight optimization. Next, the model generates the feature representation for the test sample and predicts its label. Additionally, the improved ml-KNN algorithm retrieves neighbors of the test sample and uses their label information for prediction. Finally, the two predictions are combined to obtain the final prediction, which accurately identifies the user's intention. The experimental results demonstrate that, on the dataset constructed in this article, the IML-CL method effectively boosts the performance of the baseline model.
更多查看译文
关键词
contrastive learning,deep learning,improved ml-KNN,multi-label text classification
AI 理解论文
溯源树
样例
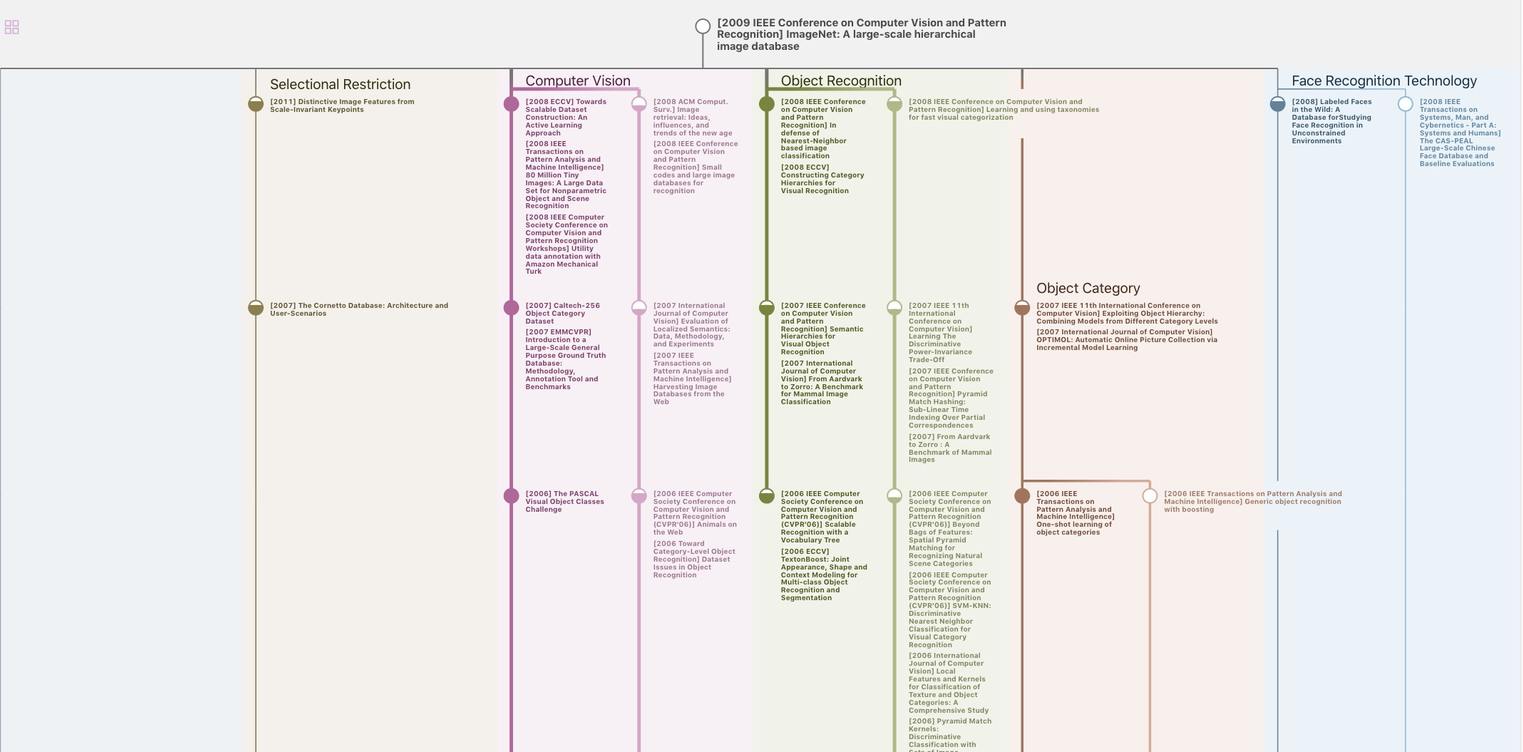
生成溯源树,研究论文发展脉络
Chat Paper
正在生成论文摘要