Reinforcement Learning Under Partial Observability Guided by Learned Environment Models
INTEGRATED FORMAL METHODS, IFM 2023(2024)
摘要
Reinforcement learning and planning under partial observability is notoriously difficult. In this setting, decision-making agents need to perform a sequence of actions with incomplete information about the underlying state of the system. As such, methods that can act in the presence of incomplete state information are of special interest to machine learning, planning, and control communities. In the scope of this paper, we consider environments that behave like a partially observable Markov decision process (POMDP) with known discrete actions, while assuming no knowledge about its structure or transition probabilities. We propose an approach for reinforcement learning (RL) in such partially observable environments. Our approach combines Q-learning with IoAlergia, an automata learning method that can learn Markov decision processes (MDPs). By learning MDP models of the environment from the experiences of the RL agent, we enable RL in partially observable domains without explicit, additional memory to track previous interactions for dealing with ambiguities stemming from partial observability. We instead provide the RL agent with additional observations in the form of abstract environment states. By simulating new experiences on a learned model we extend the agent's internal state representation, which in turn enables better decision-making in the presence of partial observability. In our evaluation we report on the validity of our approach and its promising performance in comparison to six state-of-the-art deep RL techniques with recurrent neural networks and fixed memory.
更多查看译文
关键词
Reinforcement Learning,Automata Learning,Partially Observable Markov Decision Processes,Markov Decision Processes
AI 理解论文
溯源树
样例
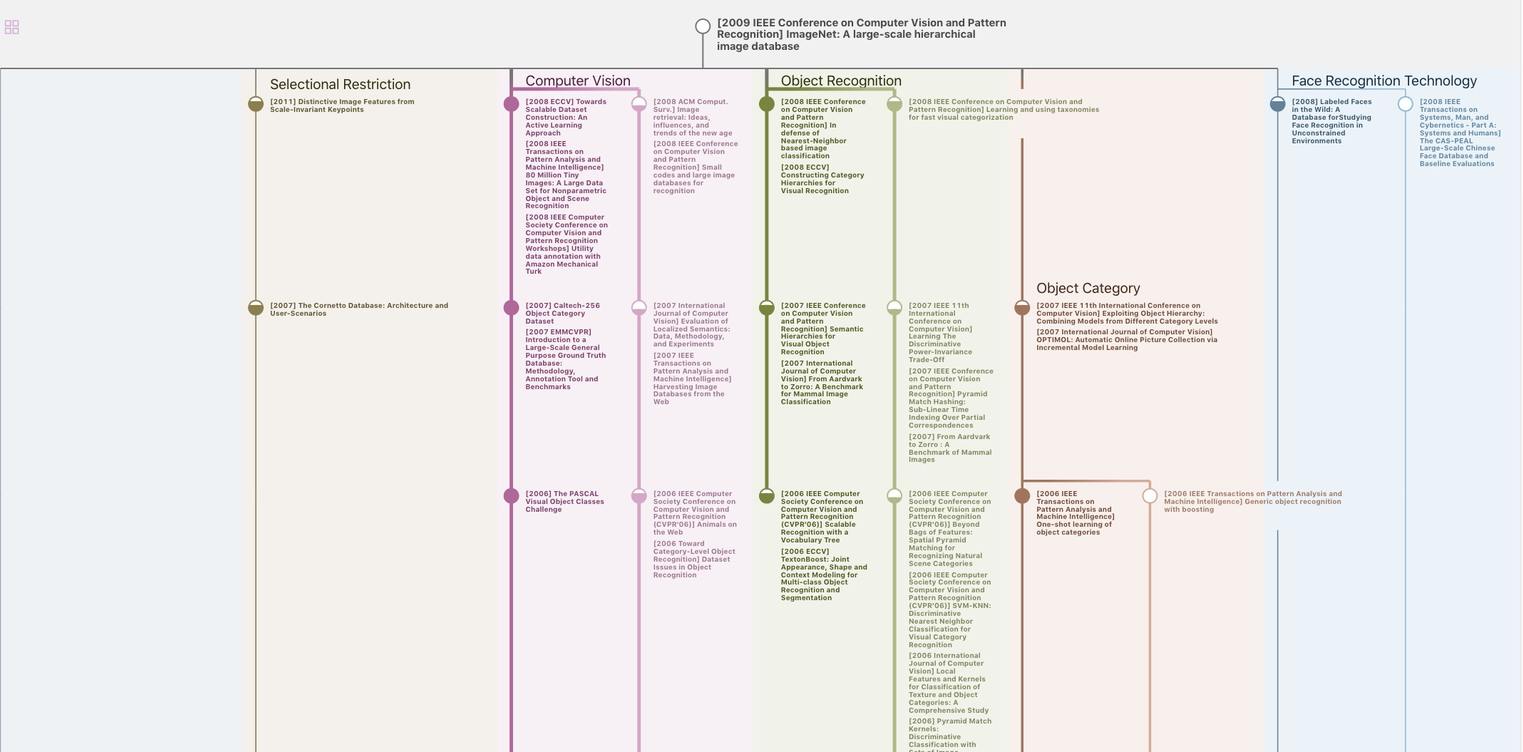
生成溯源树,研究论文发展脉络
Chat Paper
正在生成论文摘要