Predicting multifaceted risks using machine learning in atrial fibrillation: insights from GLORIA-AF study
EUROPEAN HEART JOURNAL - DIGITAL HEALTH(2024)
Abstract
Aims Patients with atrial fibrillation (AF) have a higher risk of ischaemic stroke and death. While anticoagulants are effective at reducing these risks, they increase the risk of bleeding. Current clinical risk scores only perform modestly in predicting adverse outcomes, especially for the outcome of death. We aimed to test the multi-label gradient boosting decision tree (ML-GBDT) model in predicting risks for adverse outcomes in a prospective global AF registry.Methods and results We studied patients from phase II/III of the Global Registry on Long-Term Oral Anti-Thrombotic Treatment in Patients with Atrial Fibrillation registry between 2011 and 2020. The outcomes were all-cause death, ischaemic stroke, and major bleeding within 1 year following the AF. We trained the ML-GBDT model and compared its discrimination with the clinical scores in predicting patient outcomes. A total of 25 656 patients were included [mean age 70.3 years (SD 10.3); 44.8% female]. Within 1 year after AF, ischaemic stroke occurred in 215 (0.8%), major bleeding in 405 (1.6%), and death in 897 (3.5%) patients. Our model achieved an optimized area under the curve in predicting death (0.785, 95% CI: 0.757-0.813) compared with the Charlson Comorbidity Index (0.747, P = 0.007), ischaemic stroke (0.691, 0.626-0.756) compared with CHA2DS2-VASc (0.613, P = 0.028), and major bleeding (0.698, 0.651-0.745) as opposed to HAS-BLED (0.607, P = 0.002), with improvement in net reclassification index (10.0, 12.5, and 23.6%, respectively).Conclusion The ML-GBDT model outperformed clinical risk scores in predicting the risks in patients with AF. This approach could be used as a single multifaceted holistic tool to optimize patient risk assessment and mitigate adverse outcomes when managing AF. Graphical Abstract Currently, clinical risk scores, derived from a specific set of weighted risk factors, are utilized to predict risks of all-cause mortality, ischaemic stroke, and major bleeding for patients diagnosed with AF. Consider a patient as an example: a patient might score 5 on the CCI, 4 on the CHA2DS2-VASc, and 3 on the HAS-BLED. Based on these scores, clinicians use predefined cut-offs to determine the suitable treatments. We aim to build a machine learning model, which integrates additional data (both input and output information) to improve risk prediction. Clinicians could leverage the developed model to enhance the overall outcomes for AF patients, benefiting from its superior predictive capabilities and the clarity provided by its feature importance ranking. AF, atrial fibrillation; CCI, Charlson Comorbidity Index; CHA2DS2-VASc includes congestive heart failure, hypertension, age, diabetes mellitus, stroke or transient ischaemic attack, vascular disease, and sex (female); HAS-BLED includes hypertension, abnormal renal or liver function, stroke, bleeding, international normalized ratio, elderly (age over 65 years), and drugs or alcohol usage; ML-GBDT, multi-label gradient boosting decision tree.
MoreTranslated text
Key words
Atrial fibrillation,Death,Ischaemic stroke,Major bleeding,Machine learning,Risk
AI Read Science
Must-Reading Tree
Example
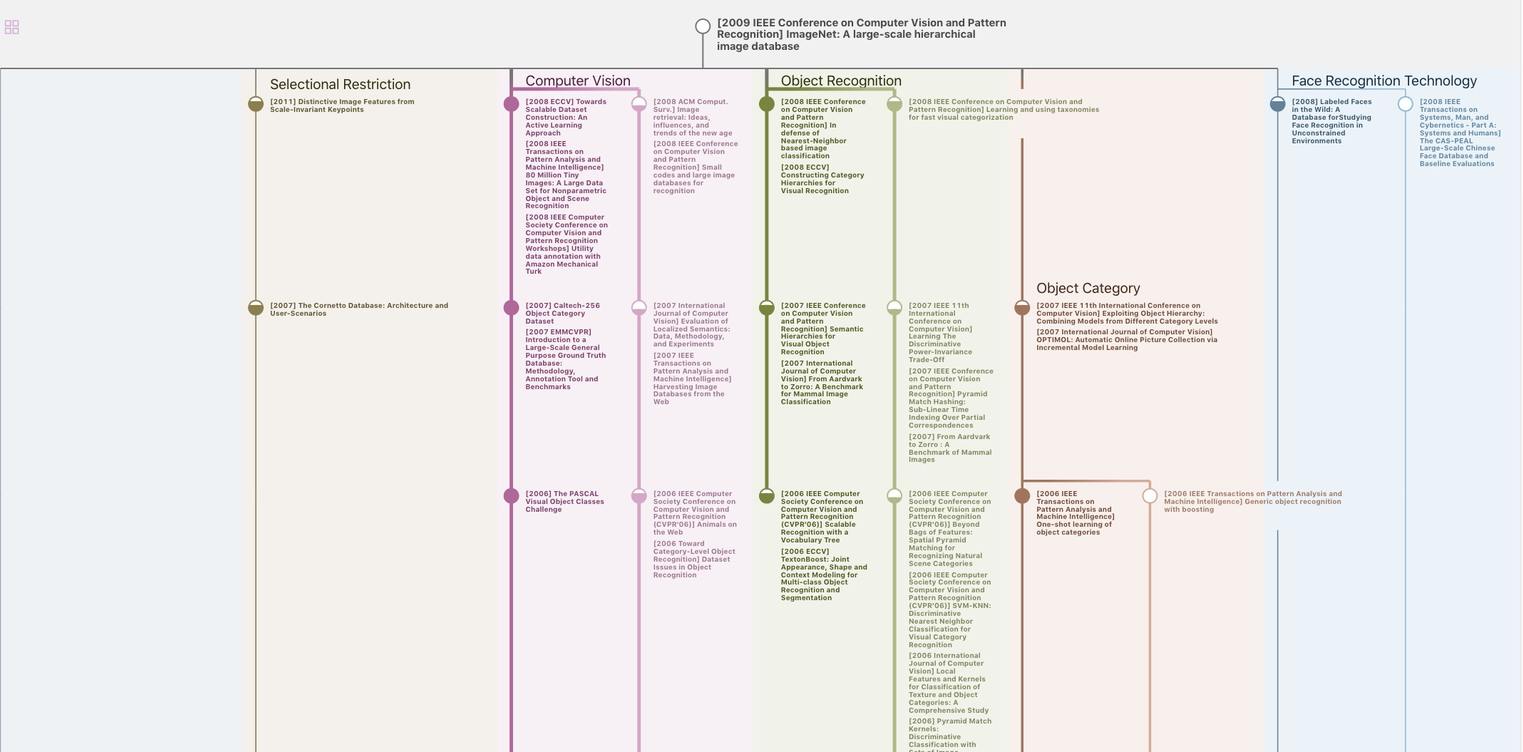
Generate MRT to find the research sequence of this paper
Chat Paper
Summary is being generated by the instructions you defined