Optimizing Photodegradation Rate Prediction of Organic Contaminants: Models with Fine-Tuned Hyperparameters and SHAP Feature Analysis for Informed Decision Making
ACS ES&T WATER(2023)
摘要
Titanium dioxide (TiO2) is commonly used as a photocatalyst for treating water contaminants. However, experimentally determining the photodegradation kinetics is both expensive and time-consuming. In this study, the authors developed gradient booster regressor (GBR) and extreme gradient boosted (XGBoost) decision tree models using the BayesSearchCV and hyperopt libraries, respectively, to fine-tune the hyperparameters. The goal was to estimate photodegradation rate constants for organic contaminants and investigate the relationship between input variables and their characteristics to enhance model performance. To assess the models' predictive capabilities, the researchers employed quantitative approaches such as the coefficient of determination (R-2), mean absolute error (MAE), and root mean squared error (RMSE). Additionally, they utilized the SHAP technique, which applies cooperative game theory to estimate photodegradation rates and rank the importance of features, to provide interpretability to the results. The study identified enhanced models that outperformed other models in the literature, with the XGBoost model combined with the TPE algorithm performing the best, achieving an R-2 of 0.891, an MAE of 0.100, and an RMSE of 0.157. Furthermore, the SHAP technique provided insights into the features predicting photodegradation rates and their correlations.
更多查看译文
关键词
photodegradation of water contaminants,gradient boosterregressor,XGBoost,BayesSearchCV,hyperopt,molecular fingerprint,SHAP interpretation
AI 理解论文
溯源树
样例
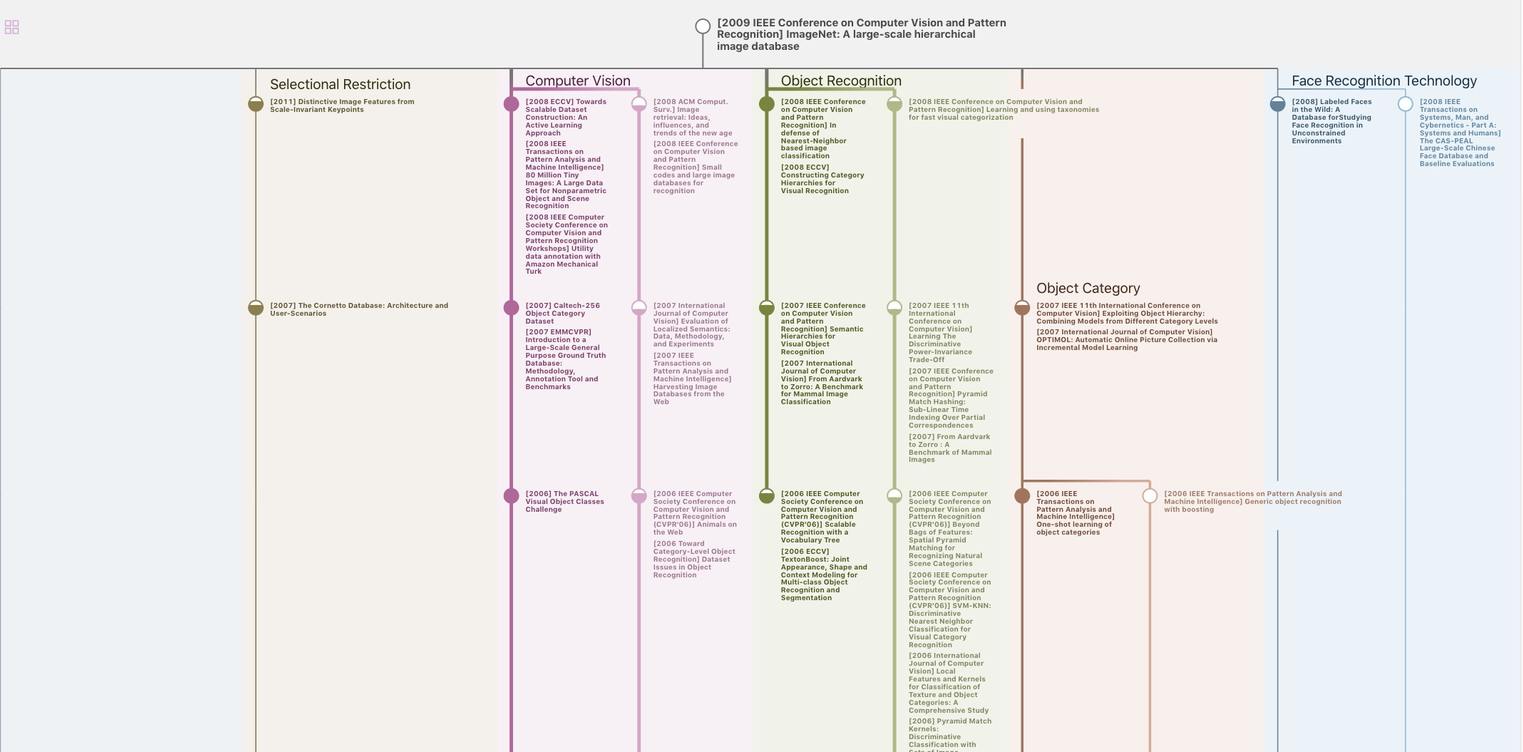
生成溯源树,研究论文发展脉络
Chat Paper
正在生成论文摘要