Statistical downscaling of high-resolution precipitation in India using convolutional long short-term memory networks
JOURNAL OF WATER AND CLIMATE CHANGE(2024)
摘要
Statistical downscaling of the General Circulation Model (GCM) simulations are widely used for accessing climate changes in the future at different spatiotemporal scales. This study proposes a novel Statistical Downscaling (SD) model established on the Convolutional Long Short-Term Memory (ConvLSTM) Network. The methodology is applied to obtain future projection of rainfall at 0.25(degrees )spatial resolution over the Indian sub-continental region. The traditional multisite downscaling models typically perform downscaling on a single homogeneous rainfall zone, predicting rainfall at only one grid point in a single model run. The proposed model captures spatiotemporal dependencies in multisite local rainfall and predicts rainfall for the entire zone in a single model run. The study proposes a Shared ConvLSTM model providing a single end-to-end supervised model for predicting the future precipitation for entire India. The model captures the regional variability in rainfall better than a region-wise trained model. The projected future rainfall for different scenarios of climate change reveals an overall increase in the rainfall mean and spatially non-uniform changes in future rainfall extremes over India. The results highlight the importance of conducting in-depth hydrologic studies for different river basins of the country for future water availability assessment and making water resource policies.
更多查看译文
关键词
climate projections,Convolutional Long Short-Term Memory (ConvLSTM) Network,India,Indian Summer Monsoon,statistical downscaling
AI 理解论文
溯源树
样例
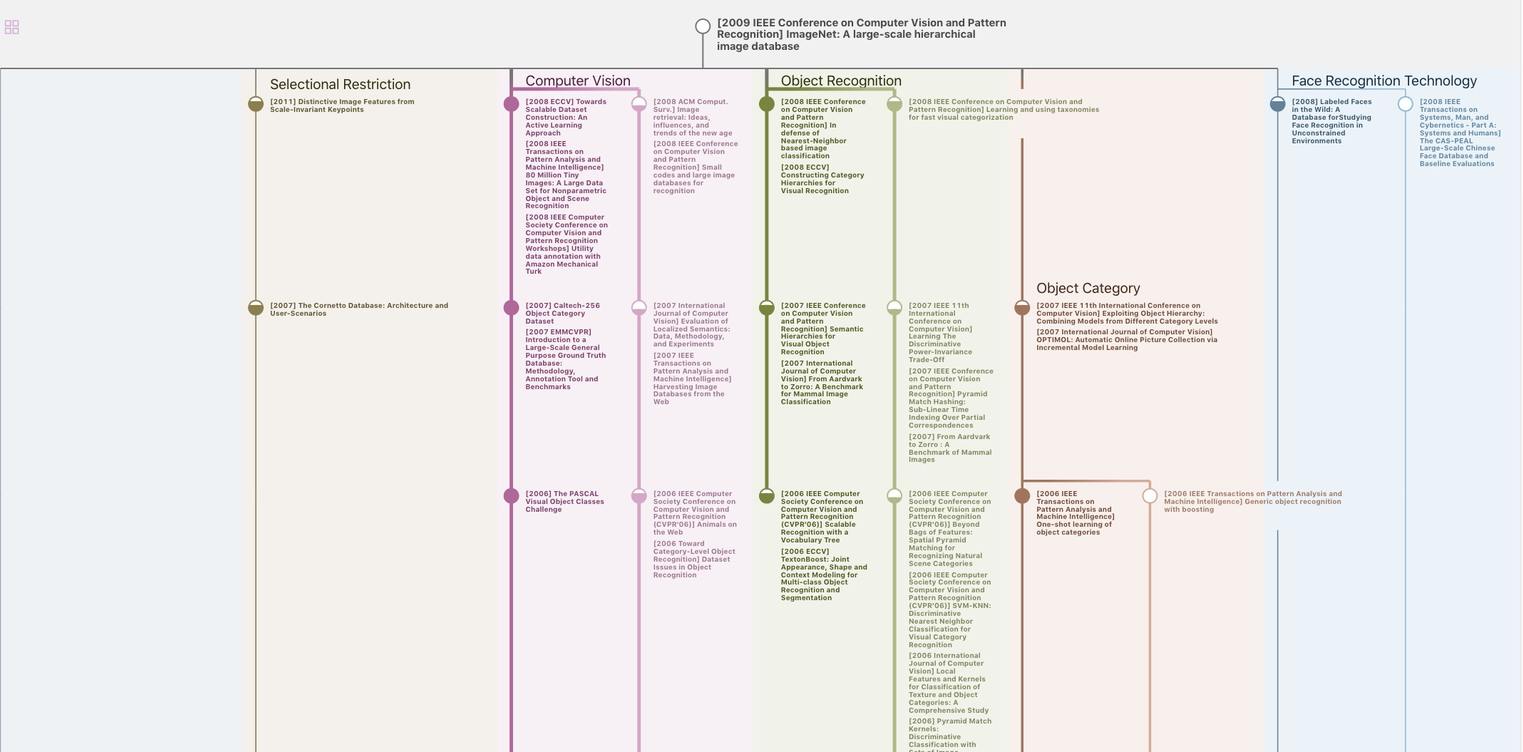
生成溯源树,研究论文发展脉络
Chat Paper
正在生成论文摘要