Dual-input ultralight multi-head self-attention learning network for hyperspectral image classification
INTERNATIONAL JOURNAL OF REMOTE SENSING(2024)
摘要
In the hyperspectral image (HSI) classification tasks, various deep learning models have achieved remarkable success. However, most deep learning models are compute-intensive, requiring significant computing power, time, and other resources. It becomes a challenge to pursue better results while saving computational resources. Therefore, a novel dual-input ultralight multi-head self-attention learning network (DUMS-LN) is proposed for HSI classification. The proposed DUMS-LN consists of three main core modules, namely the high-dimensional reduced module (HDRM), lightweight multi-head self-attention (LMHSA) module, and linearized hierarchical conversion module (LHCM). HDRM is used as a pre-processing module with efficient data compression and combines spatial and spectral information extraction from the raw data to provide cleaner and more comprehensive feature data for subsequent processing. In addition, the core computational module of DUMS-LN is the LMHSA module, which is lightweight but possesses better data processing capability than the traditional multi-head self-attention module. Finally, the LHCM divides the model into two phases, reducing the dimensionality of the feature data phase by phase so that the LMHSA module can perform feature extraction at different levels. Experiments on four benchmark HSI datasets show that the proposed DUMS-LN outperforms the comparison HSI classification algorithms regarding speed and classification accuracy.
更多查看译文
关键词
Remote sensing,hyperspectral image classification,multi-head self-attention,deep learning
AI 理解论文
溯源树
样例
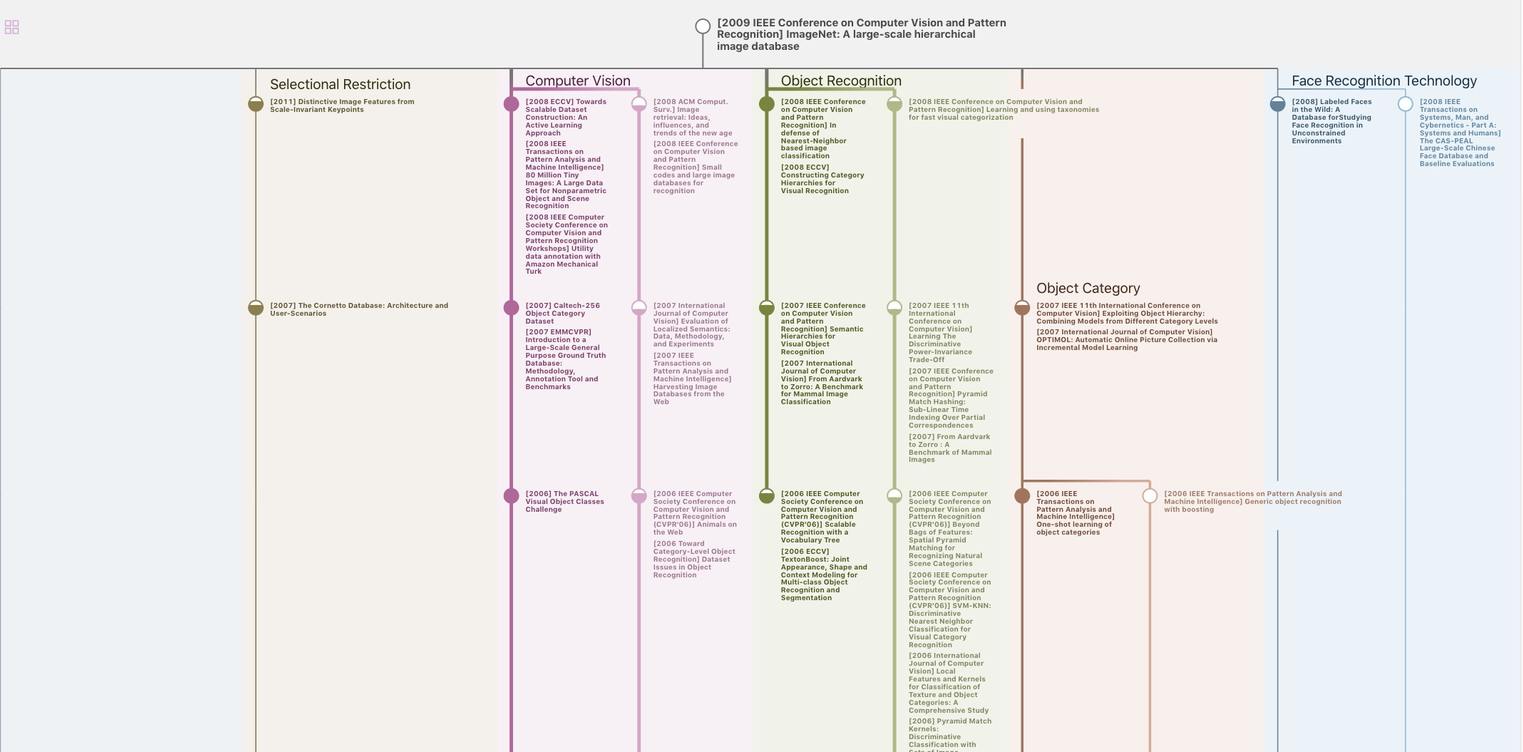
生成溯源树,研究论文发展脉络
Chat Paper
正在生成论文摘要