SLAM-RAMU: 3D LiDAR-IMU lifelong SLAM with relocalization and autonomous map updating for accurate and reliable navigation
INDUSTRIAL ROBOT-THE INTERNATIONAL JOURNAL OF ROBOTICS RESEARCH AND APPLICATION(2024)
摘要
PurposeAutonomous mobile robots (AMRs) play a crucial role in industrial and service fields. The paper aims to build a LiDAR-based simultaneous localization and mapping (SLAM) system used by AMRs to overcome challenges in dynamic and changing environments.Design/methodology/approachThis research introduces SLAM-RAMU, a lifelong SLAM system that addresses these challenges by providing precise and consistent relocalization and autonomous map updating (RAMU). During the mapping process, local odometry is obtained using iterative error state Kalman filtering, while back-end loop detection and global pose graph optimization are used for accurate trajectory correction. In addition, a fast point cloud segmentation module is incorporated to robustly distinguish between floor, walls and roof in the environment. The segmented point clouds are then used to generate a 2.5D grid map, with particular emphasis on floor detection to filter the prior map and eliminate dynamic artifacts. In the positioning process, an initial pose alignment method is designed, which combines 2D branch-and-bound search with 3D iterative closest point registration. This method ensures high accuracy even in scenes with similar characteristics. Subsequently, scan-to-map registration is performed using the segmented point cloud on the prior map. The system also includes a map updating module that takes into account historical point cloud segmentation results. It selectively incorporates or excludes new point cloud data to ensure consistent reflection of the real environment in the map.FindingsThe performance of the SLAM-RAMU system was evaluated in real-world environments and compared against state-of-the-art (SOTA) methods. The results demonstrate that SLAM-RAMU achieves higher mapping quality and relocalization accuracy and exhibits robustness against dynamic obstacles and environmental changes.Originality/valueCompared to other SOTA methods in simulation and real environments, SLAM-RAMU showed higher mapping quality, faster initial aligning speed and higher repeated localization accuracy.
更多查看译文
关键词
Dynamic removal,Lifelong SLAM,Map update,Relocalization
AI 理解论文
溯源树
样例
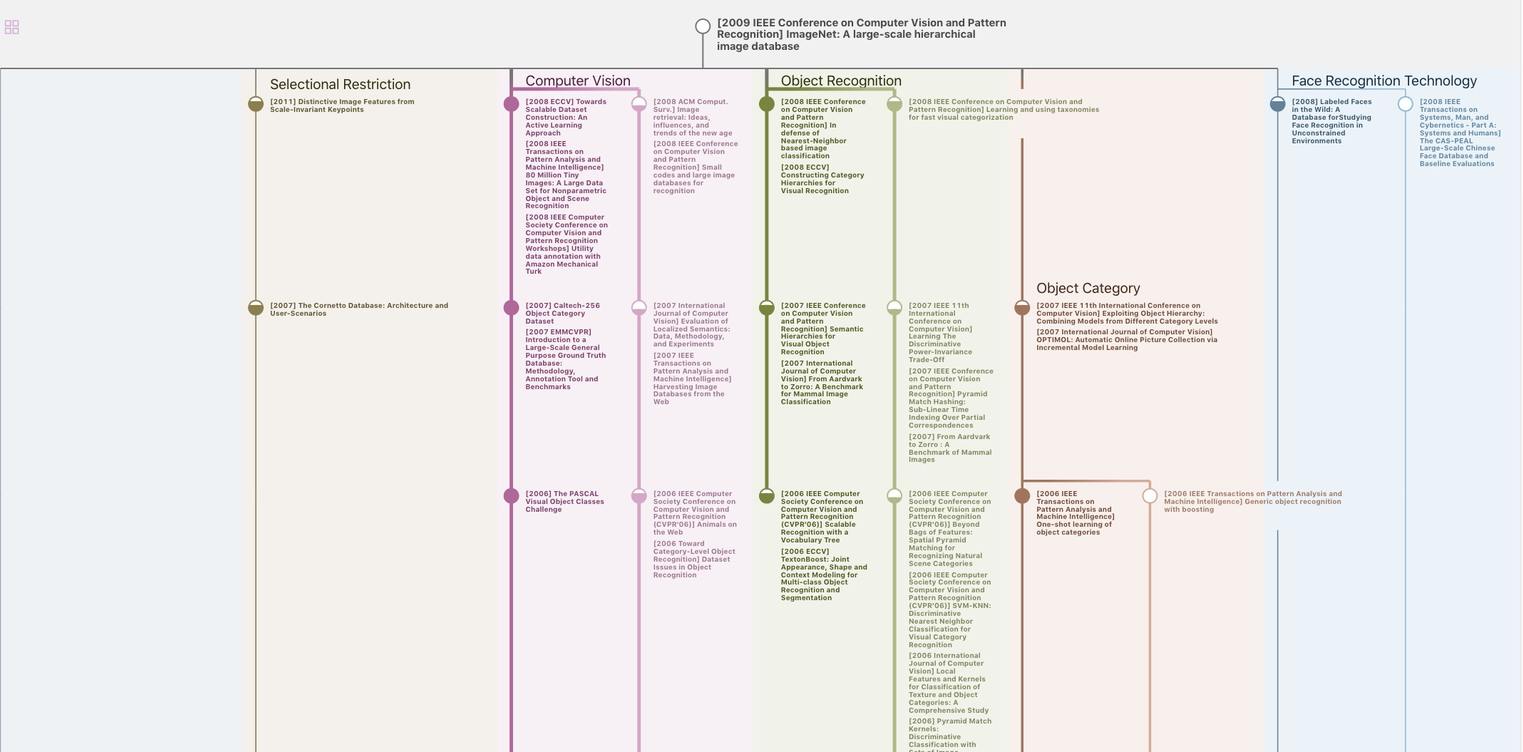
生成溯源树,研究论文发展脉络
Chat Paper
正在生成论文摘要