Data-Driven Compositional Optimization in Misspecified Regimes
OPERATIONS RESEARCH(2024)
关键词
stochastic optimization,compositional optimization,misspecification,risk-averse optimization
AI 理解论文
溯源树
样例
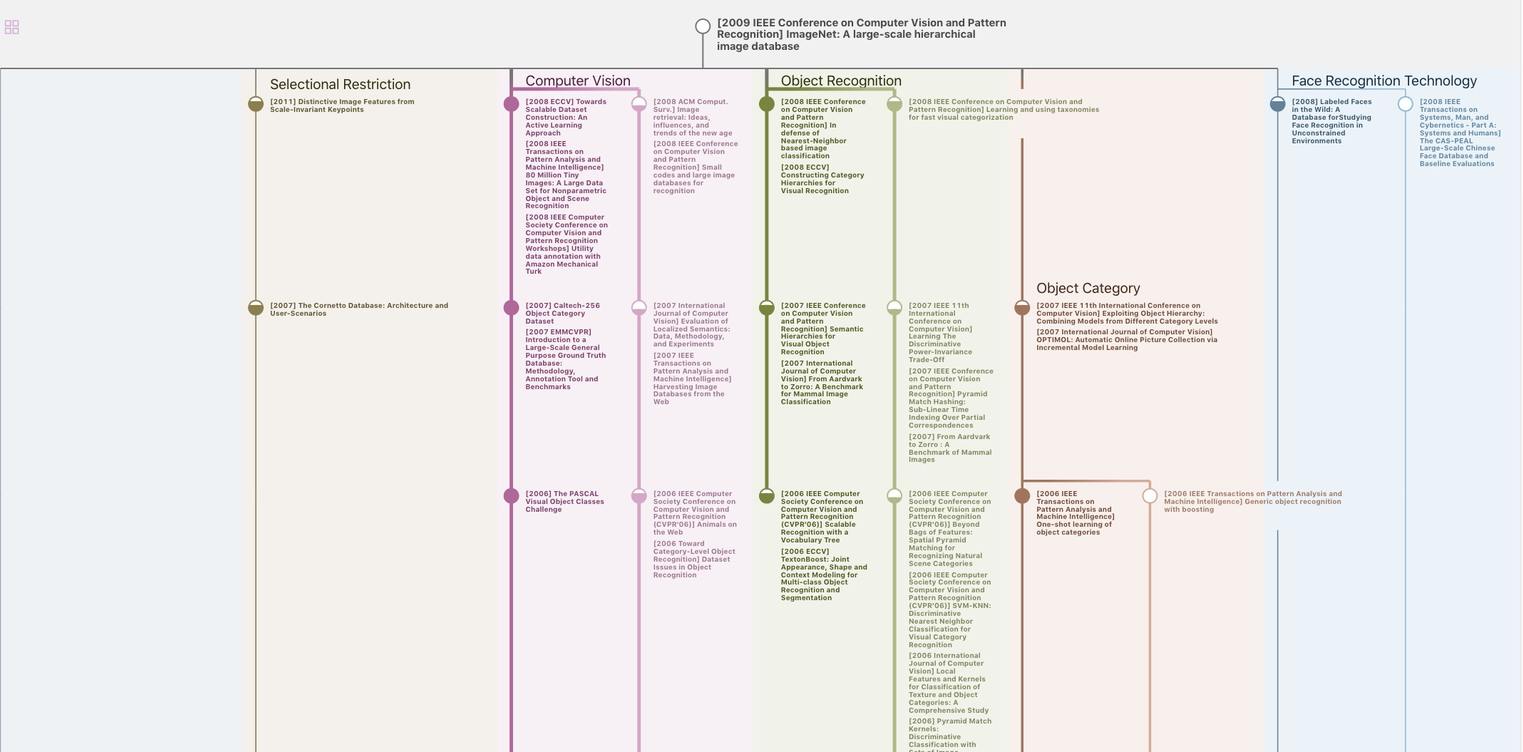
生成溯源树,研究论文发展脉络
Chat Paper
正在生成论文摘要
OPERATIONS RESEARCH(2024)