How predictable are macroscopic traffic states: a perspective of uncertainty quantification
TRANSPORTMETRICA B-TRANSPORT DYNAMICS(2024)
Abstract
Traffic condition forecasting is fundamental for Intelligent Transportation Systems. Besides accuracy, many services require an estimate of uncertainty for each prediction. Uncertainty quantification must consider the inherent randomness in traffic dynamics, the so-called aleatoric uncertainty, and the additional distrust caused by data shortage, the so-called epistemic uncertainty. They together depict how predictable macroscopic traffic is. This study uses deep ensembles of graph neural networks to estimate both types of uncertainty in network-level speed forecasting. Experimental results given by the used model reveal that, although rare congestion patterns arise randomly, the short-term predictability of traffic states is mainly restricted by the irreducible stochasticity in traffic dynamics. The predicted future state bifurcates into congested or free-flowing cases. This study suggests that the potential for improving prediction models through expanding speed and flow data is limited while diversifying data types is crucial.
MoreTranslated text
Key words
Traffic forecasting,uncertainty quantification,traffic dynamics,predictability
AI Read Science
Must-Reading Tree
Example
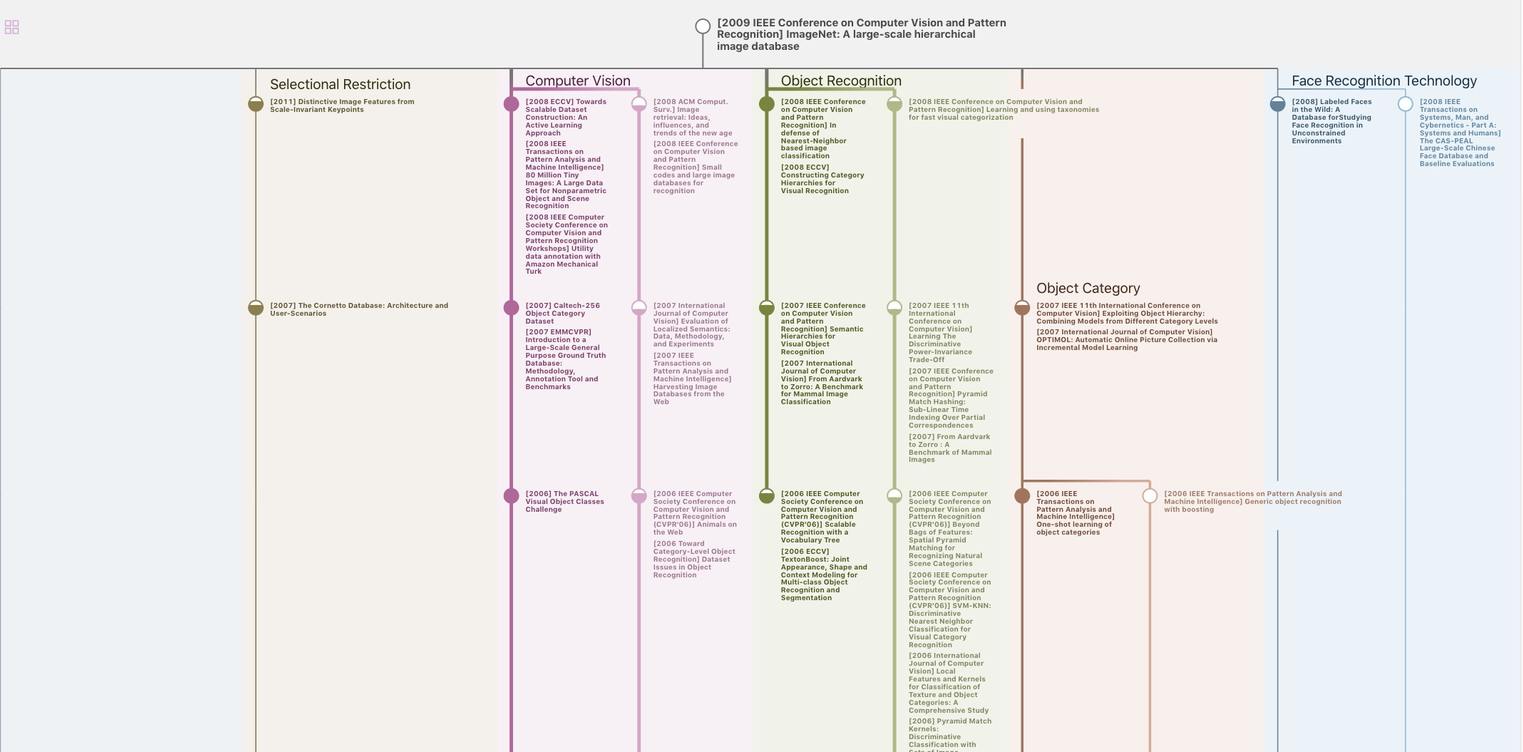
Generate MRT to find the research sequence of this paper
Chat Paper
Summary is being generated by the instructions you defined