Learning to Match Features with Geometry-Aware Pooling
NEURAL INFORMATION PROCESSING, ICONIP 2023, PT VI(2024)
摘要
and crucial step for many computer vision tasks, such as 3D-reconstruction and virtual reality. However, previous studies still struggle in challenging cases, including large view changes, repetitive pattern and textureless regions, due to the neglect of geometric constraint in the process of feature encoding. Accordingly, we propose a novel GPMatcher, which is designed to introduce geometric constraints and guidance in the feature encoding process. To achieve this goal, we compute camera poses with the corresponding features in each attention layer and adopt a geometry-aware pooling to reduce the redundant information in the next layer. By these means, an iterative geometry-aware pooing and pose estimation pipeline is constructed, which avoids the updating of redundant features and reduces the impact of noise. Experiments conducted on a range of evaluation benchmarks demonstrate that our method improves the matching accuracy and achieves the state-of-the-art performance.
更多查看译文
关键词
Image matching,Pose estimation,Transformer
AI 理解论文
溯源树
样例
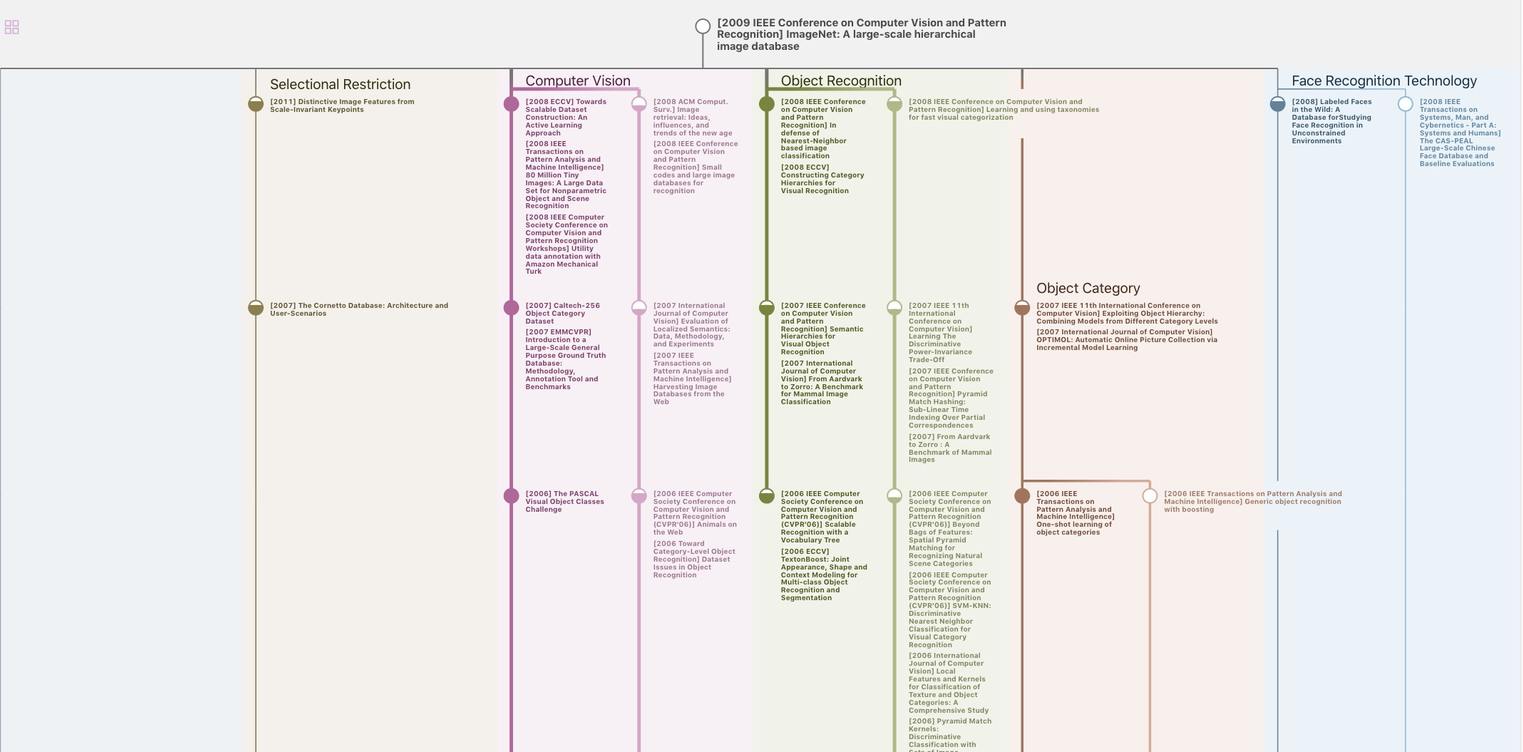
生成溯源树,研究论文发展脉络
Chat Paper
正在生成论文摘要