Seasonal time-series imputation of gap missing algorithm (stigma)
KYBERNETIKA(2023)
摘要
This work presents a new approach for the imputation of missing data in weather timeseries from a seasonal pattern; the seasonal time-series imputation of gap missing algorithm (STIGMA). The algorithm takes advantage from a seasonal pattern for the imputation of unknown data by averaging available data. We test the algorithm using data measured every 10 minutes over a period of 365 days during the year 2010; the variables include global irradiance, diffuse irradiance, ultraviolet irradiance, and temperature, arranged in a matrix of dimensions 52,560 rows for data points over time and 4 columns for weather variables. The particularity of this work is that the algorithm is well-suited for the imputation of values when the missing data are presented continuously and in seasonal patterns. The algorithm employs a date-time index to collect available data for the imputation of missing data, repeating the process until all missing values are calculated. The tests are performed by removing 5%, 10%, 15%, 20%, 25%, and 30% of the available data, and the results are compared to autoregressive models. The proposed algorithm has been successfully tested with a maximum of 2, 736 contiguous missing values that account for 19 consecutive days of a single month; this dataset is a portion of all the missing values when the time-series lacks 30% of all data. The metrics to measure the performance of the algorithms are root-mean-square error (RMSE) and the coefficient of determination (R2). The results indicate that the proposed algorithm outperforms autoregressive models while preserving the seasonal behavior of the time-series. The STIGMA is also tested with non-weather time-series of beer sales and number of air passengers per month, which also have a cyclical pattern, and the results show the precise imputation of data.
更多查看译文
关键词
contiguous missing values,seasonal patterns,time-series
AI 理解论文
溯源树
样例
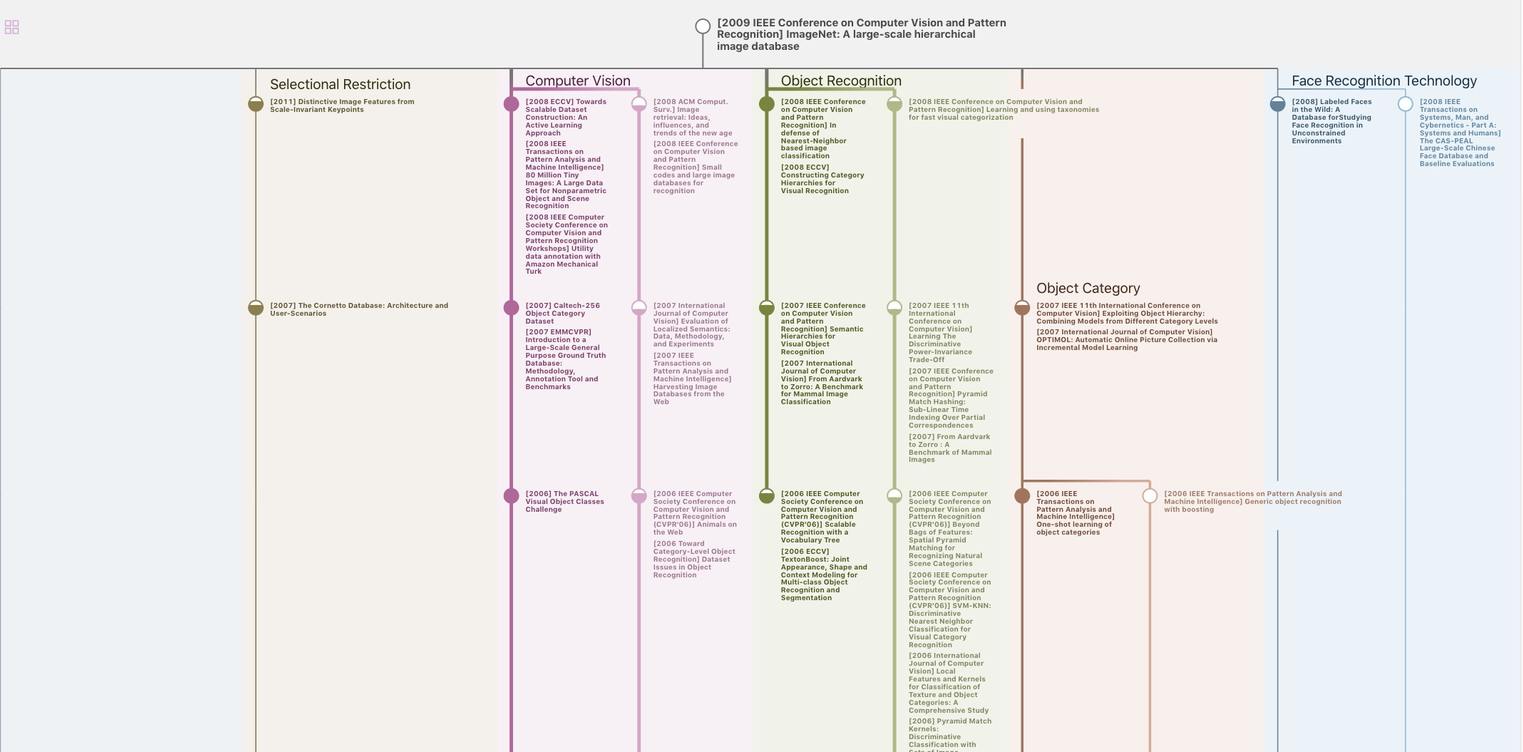
生成溯源树,研究论文发展脉络
Chat Paper
正在生成论文摘要