Exploring Multiscale Spatial Interactions: Multiscale Geographically Weighted Negative Binomial Regression
ANNALS OF THE AMERICAN ASSOCIATION OF GEOGRAPHERS(2024)
摘要
In this article, I develop and implement the multiscale geographically weighted negative binomial (MGWNB) model, extending the spatially weighted interaction models by integrating a multiscale framework. This model effectively tackles the multiscale nonstationarity and overdispersion issues found in spatial interaction models. By comparing it with multiscale geographically weighted Poisson regression using simulated data, I demonstrate its superior performance in several aspects, including its capability to estimate the scale of processes, its effectiveness in capturing the spatial heterogeneity, and its ability to produce a better goodness of fit. The application of MGWNB in interprovincial population migration in China, using 2020 Chinese census data, also demonstrates its effectiveness and efficiency, revealing strong multiscale spatial heterogeneity in the migration patterns.
更多查看译文
关键词
multiscale geographically weighted regression,negative binomial,spatial heterogeneity,spatial interaction
AI 理解论文
溯源树
样例
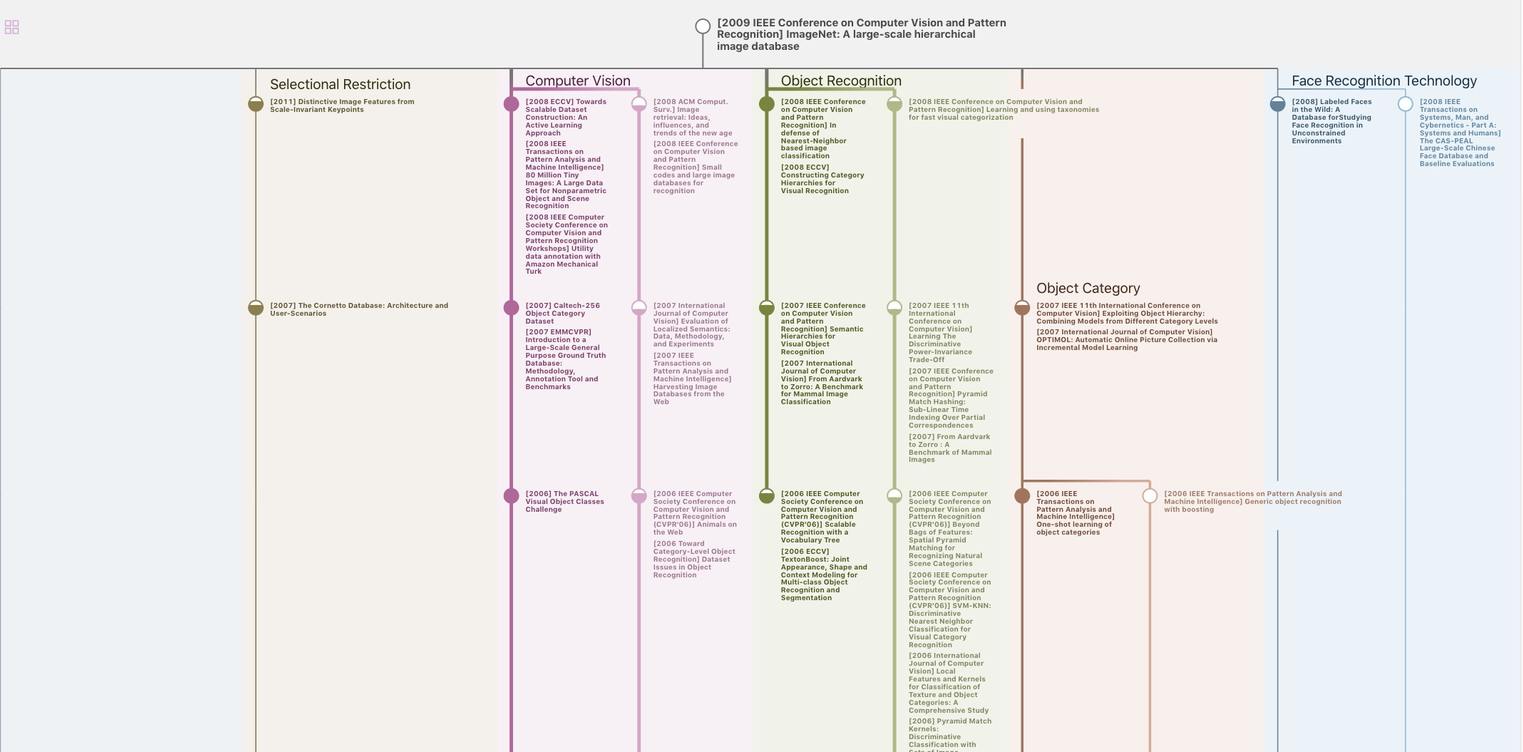
生成溯源树,研究论文发展脉络
Chat Paper
正在生成论文摘要