Spatial Decomposition and Aggregation for Attention in Convolutional Neural Networks
INTERNATIONAL JOURNAL OF PATTERN RECOGNITION AND ARTIFICIAL INTELLIGENCE(2024)
摘要
Channel attention has been shown to improve the performance of deep convolutional neural networks efficiently. Channel attention adaptively recalibrates the importance of each channel, determining what to attend to. However, channel attention only encodes inter-channel information but neglects the importance of positional information. Positional information is crucial in determining where to attend to. To address this issue, we propose a novel channel-spatial attention method named Spatial-Decomposition-Aggregation Attention (SDAA) method. First, a high-axis spatial direction is decomposed into multiple low-axis spatial directions. Then, a shared transformation sub-unit establishes attention in each low-axis space direction. Next, all the low-axis attention masks are aggregated into a high-axis attention mask. Finally, the generated high-axis attention mask is fused into the input features, thus enhancing the input features. Essentially, our method is a divide-and-conquer process. Experimental results demonstrate that our SDAA method outperforms the existing channel-spatial attention methods.
更多查看译文
关键词
Convolutional neural networks,channel-spatial attention,spatial decomposition,spatial aggregation
AI 理解论文
溯源树
样例
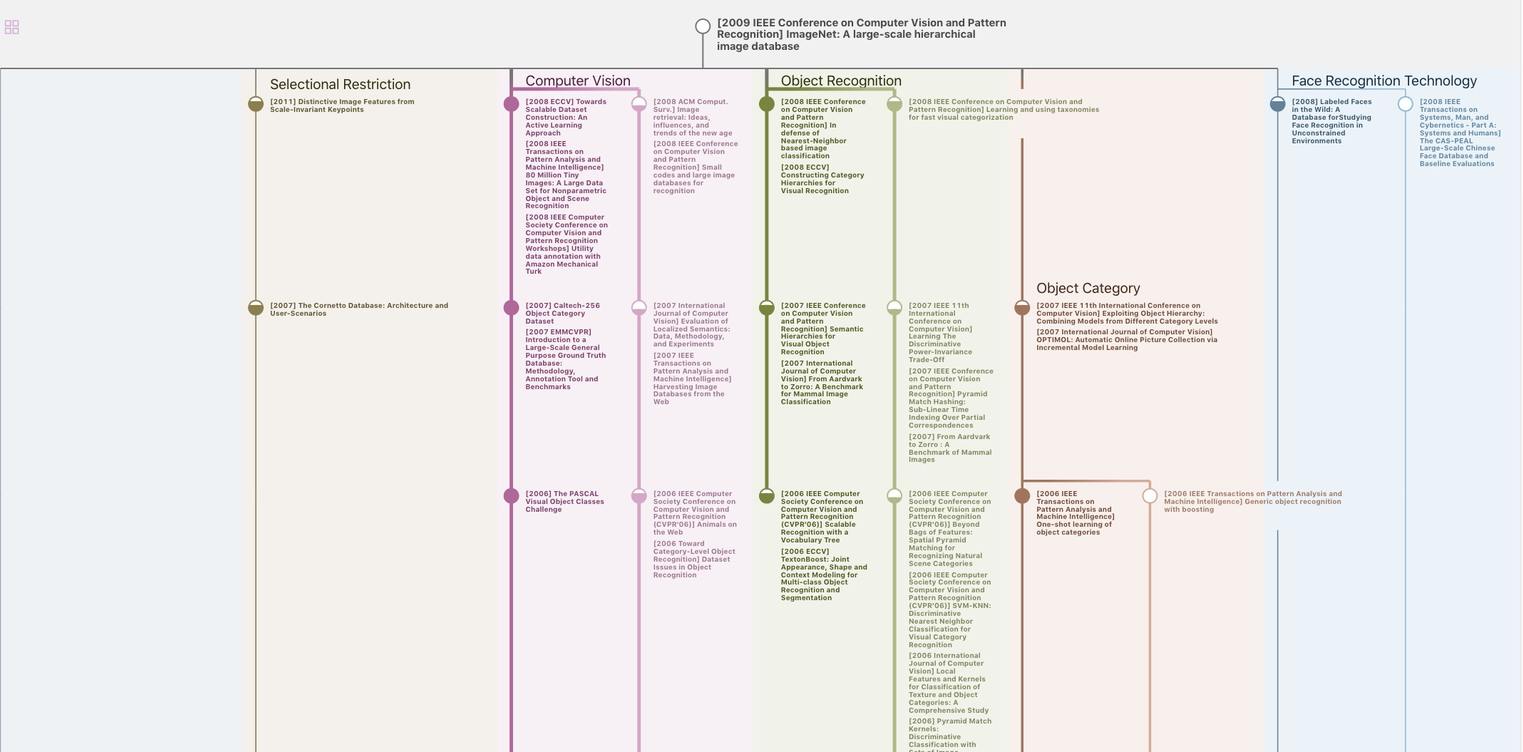
生成溯源树,研究论文发展脉络
Chat Paper
正在生成论文摘要