Modified Yolov3-Tiny Using Dilated Convolution For Driver Distraction Detection
2020 IEEE INTERNATIONAL CONFERENCE ON CONSUMER ELECTRONICS - TAIWAN (ICCE-TAIWAN)(2020)
Abstract
In this paper, the proposed YOLOv3-tiny-dilated network is presented. The well-known YOLO series neural network is famous for its rapidity, and the lightweight version is more suitable for mobile devices or embedded systems. However, the accuracy of one-stage network is slightly lower. Therefore, scale variation problem is investigated in this paper and we replaced the original Feature Pyramid Network (FPN) of the original YOLOv3-tiny network by three dilated convolution branches of different dilation rates to increase the accuracy of the network. As a result, the mean average precision (mAP) was improved by about 2% in the COCO dataset test. Besides, the proposed network was used to identify driving distraction behavior and obtain good accuracy of 95.47%.
MoreTranslated text
Key words
COCO dataset,dilation rate,dilated convolution branches,feature pyramid network,scale variation problem,one-stage network,embedded systems,mobile devices,YOLO series neural network,YOLOv3-tiny-dilated network,driver distraction detection,modified YOLOv3-tiny
AI Read Science
Must-Reading Tree
Example
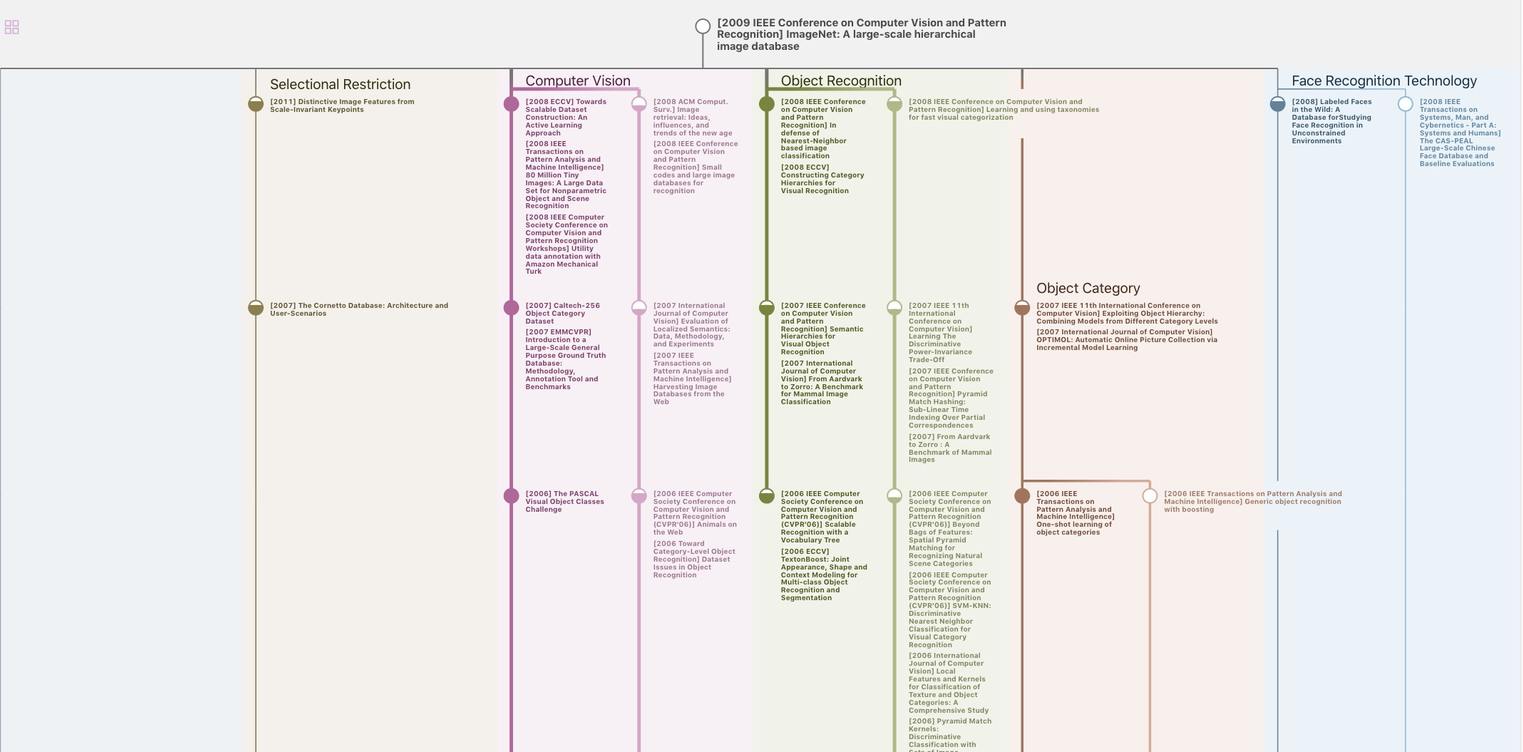
Generate MRT to find the research sequence of this paper
Chat Paper
Summary is being generated by the instructions you defined