Towards adversarial robustness verification of no-reference imageand video-quality metrics
COMPUTER VISION AND IMAGE UNDERSTANDING(2024)
摘要
In this paper, we propose a new method of analysing the stability of modern deep imageand video -quality metrics to different adversarial attacks. The stability analysis of quality metrics is becoming important because nowadays the majority of metrics employ neural networks. Unlike traditional quality metrics based on nature scene statistics or other hand -crafter features, learning -based methods are more vulnerable to adversarial attacks. The usage of such unstable metrics in benchmarks may lead to being exploited by the developers of image and video processing algorithms to achieve higher positions in leaderboards. The majority of known adversarial attacks on images designed for computer vision tasks are not fast enough to be used within realtime video processing algorithms. We propose four fast attacks on metrics suitable for real -life scenarios. The proposed methods are based on creating perturbations that increase metrics scores and can be applied frame -by -frame to attack videos. We analyse the stability of seven widely used no -reference imageand videoquality metrics to proposed attacks. The results showed that only three metrics are stable against our real -life attacks. This research yields insights to further aid in designing stable neural -network -based no -reference quality metrics. Proposed attacks can serve as an additional verification of metrics' reliability.
更多查看译文
关键词
Image Quality Assessment,Blind Image Quality Assessment,Attacks on Image-Quality Metrics
AI 理解论文
溯源树
样例
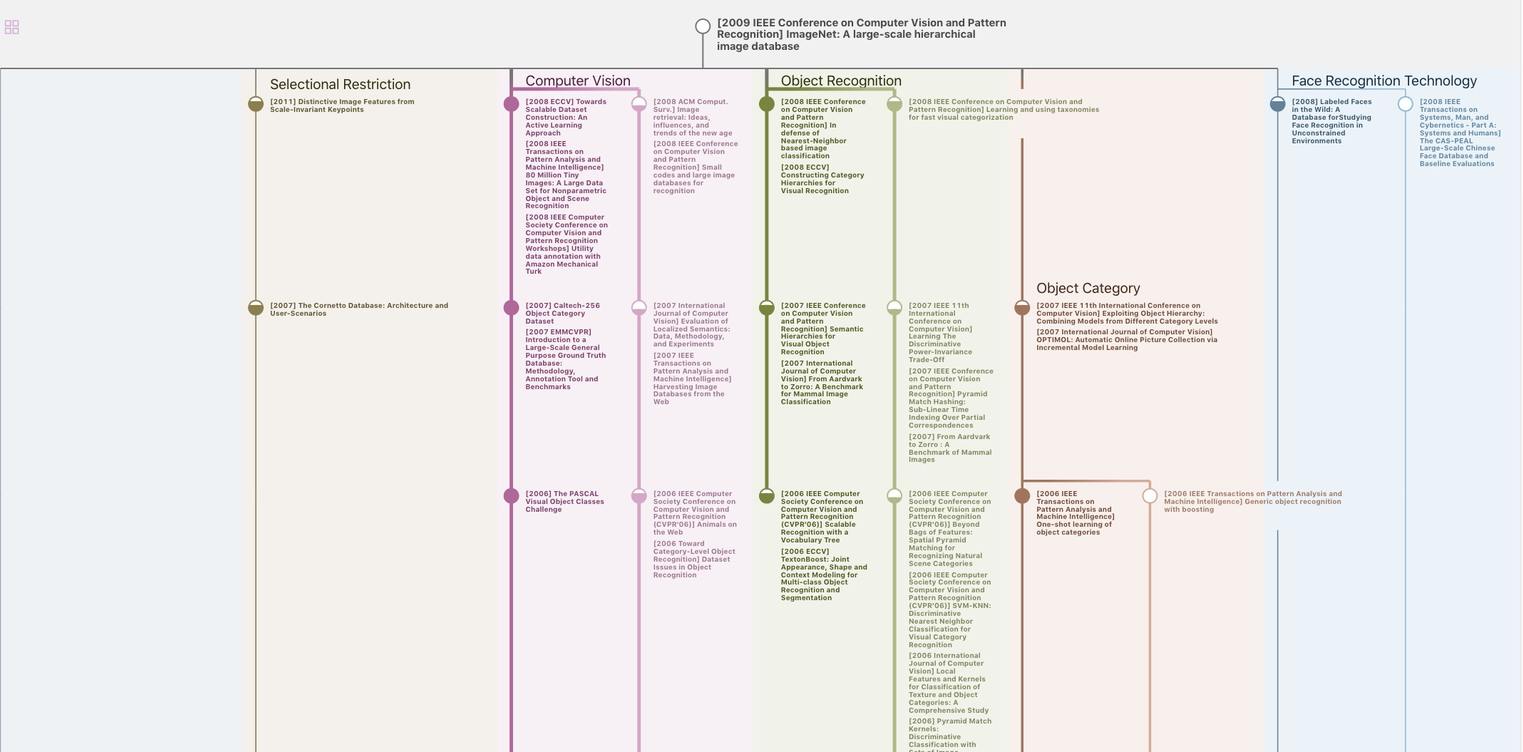
生成溯源树,研究论文发展脉络
Chat Paper
正在生成论文摘要