Identification and validation of an explainable prediction model of acute kidney injury with prognostic implications in ill children: a multicenter cohort
ECLINICALMEDICINE(2024)
摘要
Background Acute kidney injury (AKI) is a common and serious organ dysfunction in critically ill children. Early identification and prediction of AKI are of great significance. However, current AKI criteria are insufficiently sensitive and specific, and AKI heterogeneity limits the clinical value of AKI biomarkers. This study aimed to establish and validate an explainable prediction model based on the machine learning (ML) approach for AKI, and assess its prognostic implications in children admitted to the pediatric intensive care unit (PICU). Methods This multicenter prospective study in China was conducted on critically ill children for the derivation and validation of the prediction model. The derivation cohort, consisting of 957 children admitted to four independent PICUs from September 2020 to January 2021, was separated for training and internal validation, and an external data set of 866 children admitted from February 2021 to February 2022 was employed for external validation. AKI was defined based on serum creatinine and urine output using the Kidney Disease: Improving Global Outcome (KDIGO) criteria. With 33 medical characteristics easily obtained or evaluated during the first 24 h after PICU admission, 11 ML algorithms were used to construct prediction models. Several evaluation indexes, including the area under the receiver -operating -characteristic curve (AUC), were used to compare the predictive performance. The SHapley Additive exPlanation method was used to rank the feature importance and explain the final model. A probability threshold for the final model was identified for AKI prediction and subgrouping. Clinical outcomes were evaluated in various subgroups determined by a combination of the final model and KDIGO criteria. Findings The random forest (RF) model performed best in discriminative ability among the 11 ML models. After reducing features according to feature importance rank, an explainable final RF model was established with 8 features. The final model could accurately predict AKI in both internal (AUC = 0.929) and external (AUC = 0.910) validations, and has been translated into a convenient tool to facilitate its utility in clinical settings. Critically ill children with a probability exceeding or equal to the threshold in the final model had a higher risk of death and multiple organ dysfunctions, regardless of whether they met the KDIGO criteria for AKI. Interpretation Our explainable ML model was not only successfully developed to accurately predict AKI but was also highly relevant to adverse outcomes in individual children at an early stage of PICU admission, and it mitigated the concern of the "black -box" issue with an undirect interpretation of the ML technique Copyright (c) 2023 The Authors. Published by Elsevier Ltd. This is an open access article under the CC BY -NC -ND license (http://creativecommons.org/licenses/by-nc-nd/4.0/).
更多查看译文
AI 理解论文
溯源树
样例
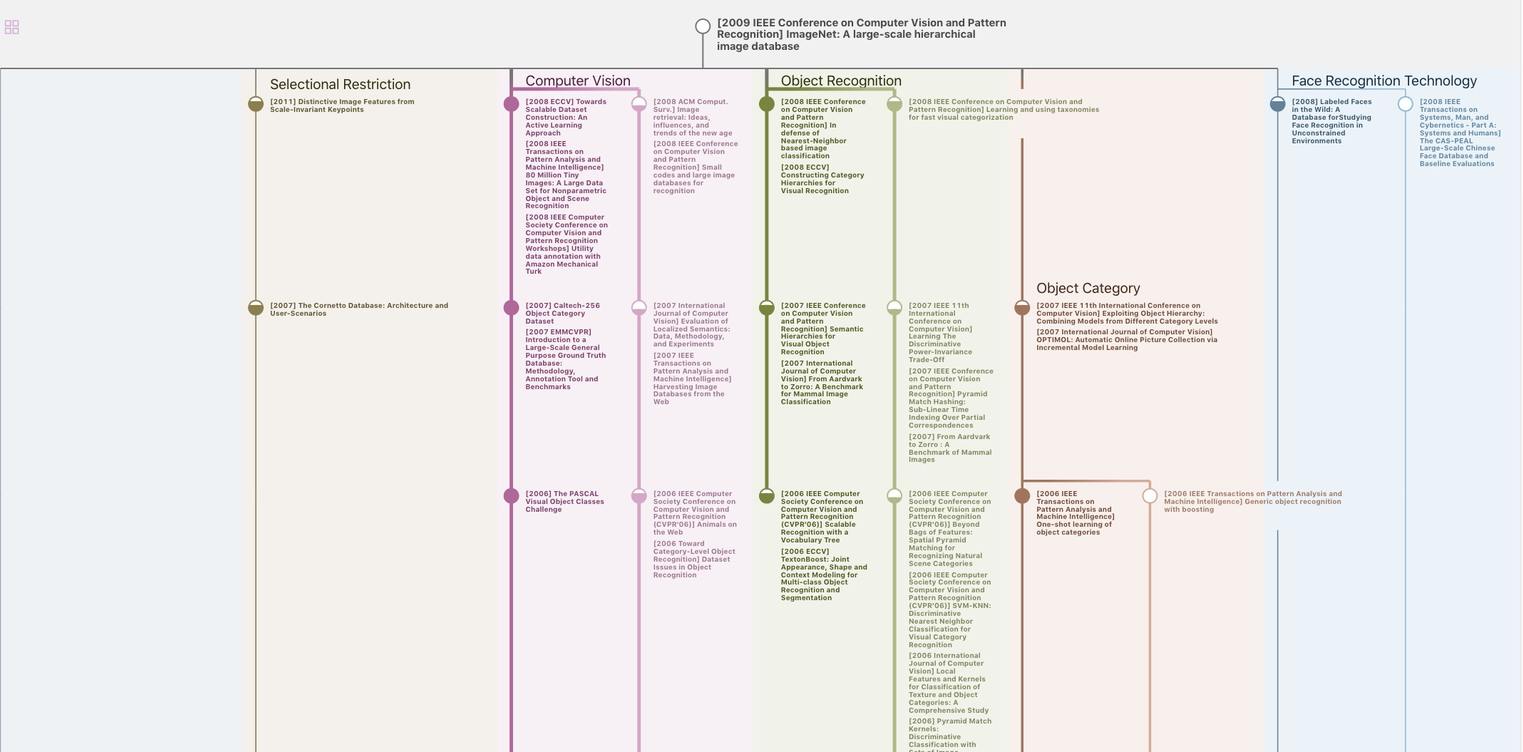
生成溯源树,研究论文发展脉络
Chat Paper
正在生成论文摘要