Empirical Forecasting Analysis of Bitcoin Prices: A Comparison of Machine Learning, Deep Learning, and Ensemble Learning Models
INTERNATIONAL JOURNAL OF ELECTRICAL AND COMPUTER ENGINEERING SYSTEMS(2024)
摘要
Bitcoin has drawn a lot of interest recently as a possible high-earning investment. There are significant financial risks associated with its erratic price volatility. Therefore, investors and decision-makers place great significance on being able to precisely foresee and capture shifting patterns in the Bitcoin market. However, empirical studies on the systems that support Bitcoin trading and forecasting are still in their infancy. The suggested method will predict the prices of all key cryptocurrencies with accuracy. A number of factors are going to be taken into account in order to precisely predict the pricing. By leveraging encryption technology, cryptocurrencies may serve as an online accounting framework and a medium of exchange. The main goal of this work is to predict Bitcoin price. To address the drawbacks of traditional forecasting techniques, we use a variety of machine learning, deep learning, and ensemble learning algorithms. We conduct a performance analysis of Auto-Regressive Integrated Moving Averages (ARIMA), Long-Short-Term Memory (LSTM), FB-Prophet, XGBoost, and a pair of hybrid formulations, LSTM-GRU and LSTM-1D_CNN. Utilizing historical Bitcoin data from 2012 to 2020, we compared the models with their Mean Absolute Error (MAE) and Root Mean Squared Error (RMSE). The hybrid LSTM-GRU model outperforms the rest with a Mean Absolute Error (MAE) of 0.464 and a Root Mean Squared Error (RMSE) of 0.323. The finding has significant ramifications for market analysts and investors in digital currencies.
更多查看译文
关键词
Bitcoin,Cryptocurrency,Arima,LSTM,Prophet,XGBOOST,Hybrid model,Prediction
AI 理解论文
溯源树
样例
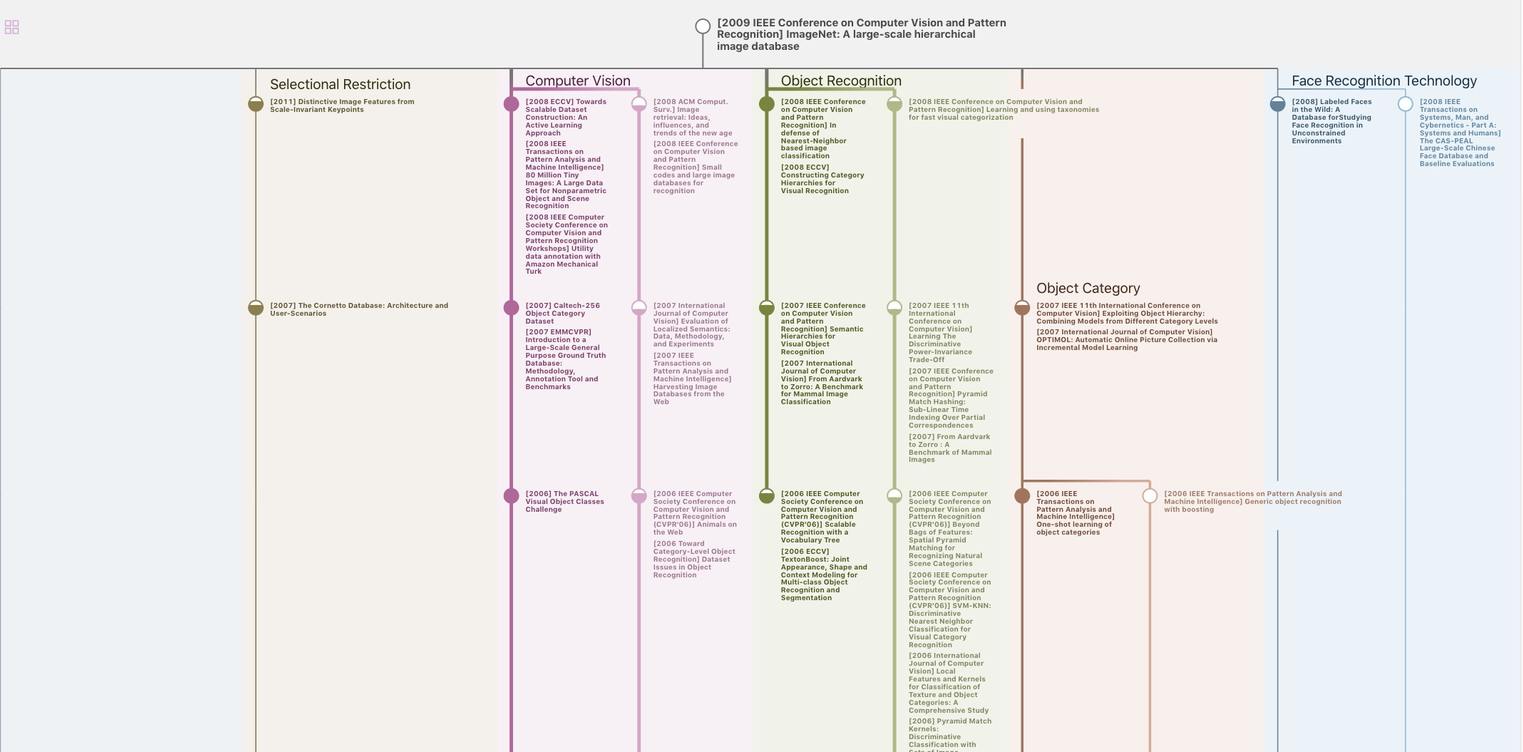
生成溯源树,研究论文发展脉络
Chat Paper
正在生成论文摘要