Enhancing reinforcement learning-based ramp metering performance at freeway uncertain bottlenecks using curriculum learning
IET INTELLIGENT TRANSPORT SYSTEMS(2024)
Abstract
Most current RM approaches are developed for fixed bottlenecks. However, the number and locations of bottlenecks are usually uncertain and even time-varying due to some unexpected phenomena, such as severe accidents and temporal lane closures. Thus, the RM approach should be able to enhance traffic flow stability by effectively handling the time-delay effect and fluctuations in traffic flow rate caused by uncertain bottlenecks. This study proposed a novel approach called deep reinforcement learning with curriculum learning (DRLCL) to improve ramp metering efficacy under uncertain bottleneck conditions. The curriculum learning method transfers an optimal control policy from a simple on-ramp bottleneck case to more challenging bottleneck tasks, while DRLCL agents explore and learn from the tasks gradually. Four RM control tasks were developed in the modified cell transmission model, including typical on-ramp bottleneck, fixed downstream bottleneck, random-location bottleneck, and multiple bottlenecks. With curriculum learning, the entire training process was reduced by 45.1% to 64.5%, while maintaining a similar maximum reward level compared to DRL-based RM control with full learning from scratch. Specifically, the results also demonstrated that the proposed DRLCL-based RM outperformed the feedback-based RM due to its stronger predictive ability, faster response, and higher action precision. This study proposed a novel approach called deep reinforcement learning with curriculum learning (DRLCL) to improve ramp metering efficacy under uncertain bottleneck conditions. The curriculum learning method transfers an optimal control policy from a simple on-ramp bottleneck case to more challenging bottleneck tasks, while DRLCL agents explore and learn from the tasks gradually. Four RM control tasks were developed in the modified cell transmission model, including typical on-ramp bottleneck, fixed downstream bottleneck, random-location bottleneck, and multiple bottlenecks. image
MoreTranslated text
Key words
artificial intelligence,metering,traffic control
AI Read Science
Must-Reading Tree
Example
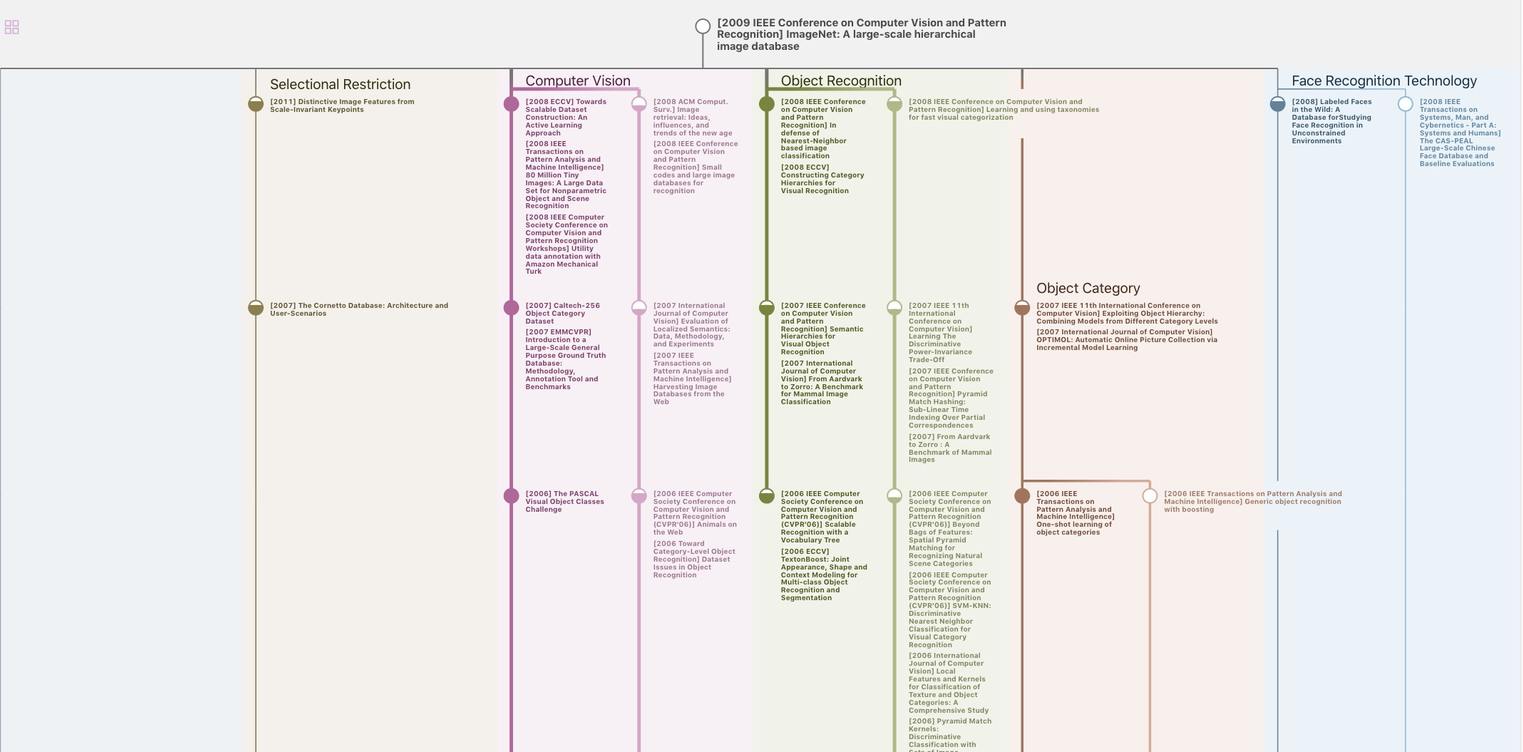
Generate MRT to find the research sequence of this paper
Chat Paper
Summary is being generated by the instructions you defined