CE2: A Copula Entropic Mutual Information Estimator for Enhancing Adversarial Robustness
PATTERN RECOGNITION AND COMPUTER VISION, PRCV 2023, PT IV(2024)
摘要
Deep neural networks are vulnerable to adversarial examples, which exploit imperceptible perturbations to mislead classifiers. To improve adversarial robustness, recent methods have focused on estimating mutual information (MI). However, existing MI estimators struggle to provide stable and reliable estimates in high-dimensional data. To this end, we propose a Copula EntropicMI Estimator (CE2) to address these limitations. CE2 leverages copula entropy to estimating MI in high dimensions, allowing target models to harness information from both clean and adversarial examples to withstand attacks. Our empirical experiments demonstrate that CE2 achieves a trade-off between variance and bias in MI estimates, resulting in stable and reliable estimates. Furthermore, the defense algorithm based on CE2 significantly enhances adversarial robustness against multiple attacks. The experimental results underscore the effectiveness of CE2 and its potential for improving adversarial robustness.
更多查看译文
关键词
Adversarial robustness,Copula entropy,Mutual information estimation
AI 理解论文
溯源树
样例
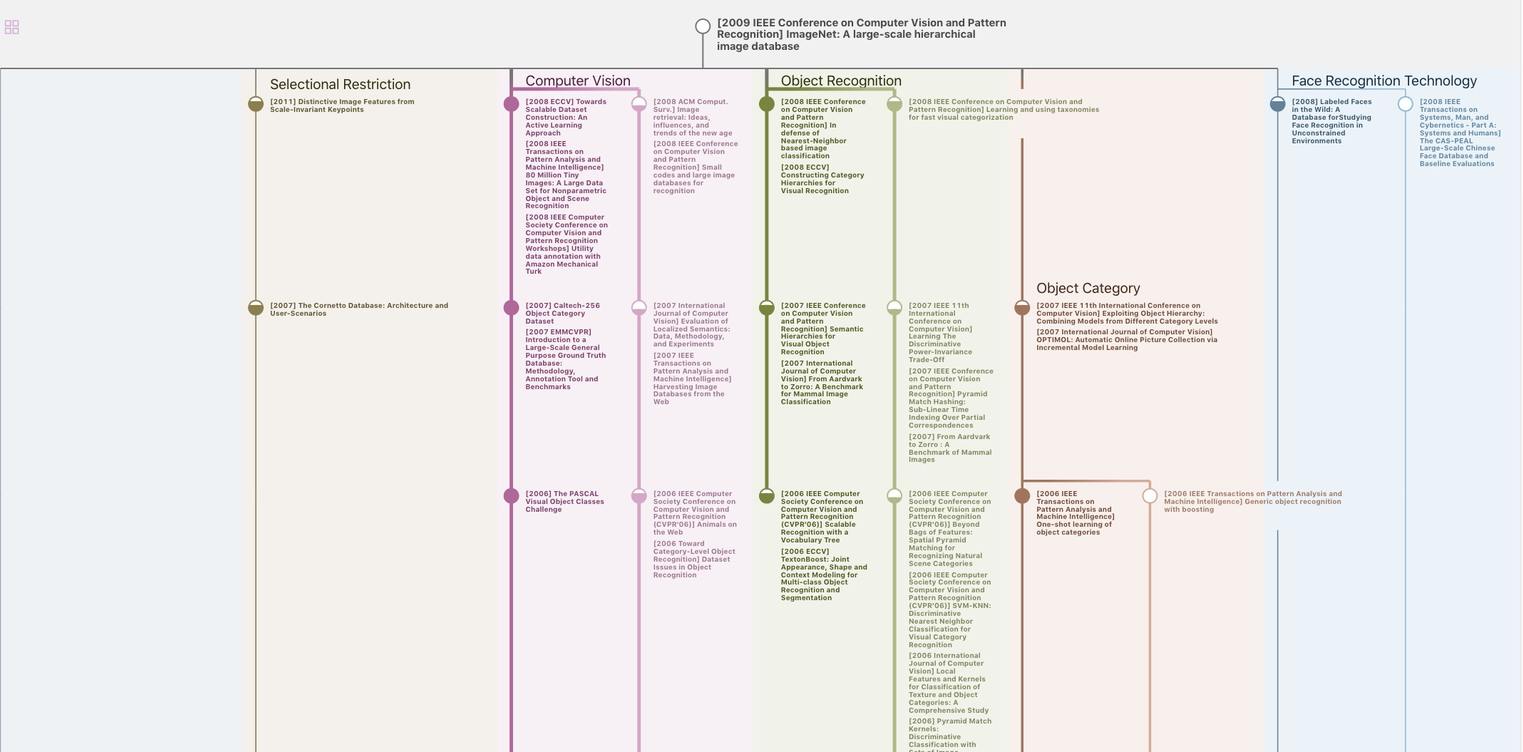
生成溯源树,研究论文发展脉络
Chat Paper
正在生成论文摘要