Simulating human mobility with a trajectory generation framework based on diffusion model
INTERNATIONAL JOURNAL OF GEOGRAPHICAL INFORMATION SCIENCE(2024)
摘要
Most mobility modeling methods are designed to solve specific tasks, leading to questions regarding their deficiency in generalizability. Inspired by the bloom of foundation models, we proposed a Trajectory Generation framework based on the Diffusion Model (TrajGDM) to capture the universal mobility pattern in a trajectory dataset by learning the trajectory generation process. The process is modeled as a step-by-step uncertainty-reducing process, in which a deep learning network with a novel training method is proposed to learn from the process. We compared the proposed trajectory generation method with six baselines on two public trajectory datasets. The results showed that the similarity between the generated and real trajectory movements measured by the Jensen-Shannon Divergence improved significantly on both datasets. Moreover, we applied zero-shot inferences on two basic trajectory tasks: trajectory prediction and trajectory reconstruction. The accuracy improved by a maximum of 25.6% on two tasks. The universal mobility pattern that is suitable for solving multiple trajectory tasks is verified, inferring the strong generalizability of our model. Finally, the study provides insights into artificial intelligence's understanding of human mobility by exploring the way the model maps the trajectory in the latent space into reality.
更多查看译文
关键词
Human mobility,trajectory generation,diffusion model,geo-foundation model
AI 理解论文
溯源树
样例
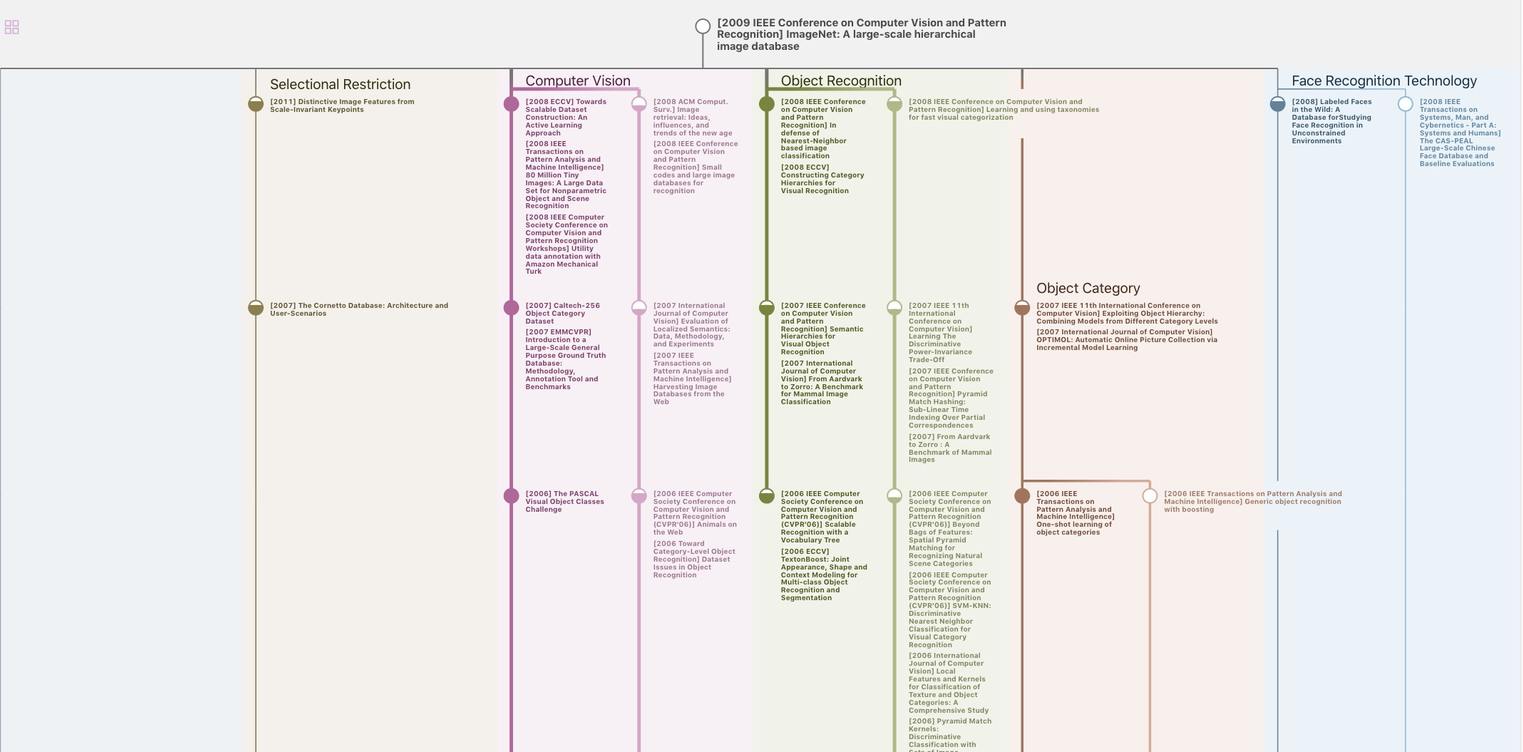
生成溯源树,研究论文发展脉络
Chat Paper
正在生成论文摘要