Physical informed memory networks for solving PDEs: implementation and applications
COMMUNICATIONS IN THEORETICAL PHYSICS(2024)
摘要
With the advent of physics informed neural networks (PINNs), deep learning has gained interest for solving nonlinear partial differential equations (PDEs) in recent years. In this paper, physics informed memory networks (PIMNs) are proposed as a new approach to solving PDEs by using physical laws and dynamic behavior of PDEs. Unlike the fully connected structure of the PINNs, the PIMNs construct the long-term dependence of the dynamics behavior with the help of the long short-term memory network. Meanwhile, the PDEs residuals are approximated using difference schemes in the form of convolution filter, which avoids information loss at the neighborhood of the sampling points. Finally, the performance of the PIMNs is assessed by solving the KdV equation and the nonlinear Schrodinger equation, and the effects of difference schemes, boundary conditions, network structure and mesh size on the solutions are discussed. Experiments show that the PIMNs are insensitive to boundary conditions and have excellent solution accuracy even with only the initial conditions.
更多查看译文
关键词
nonlinear partial differential equations,physics informed memory networks,physics informed neural networks,numerical solution
AI 理解论文
溯源树
样例
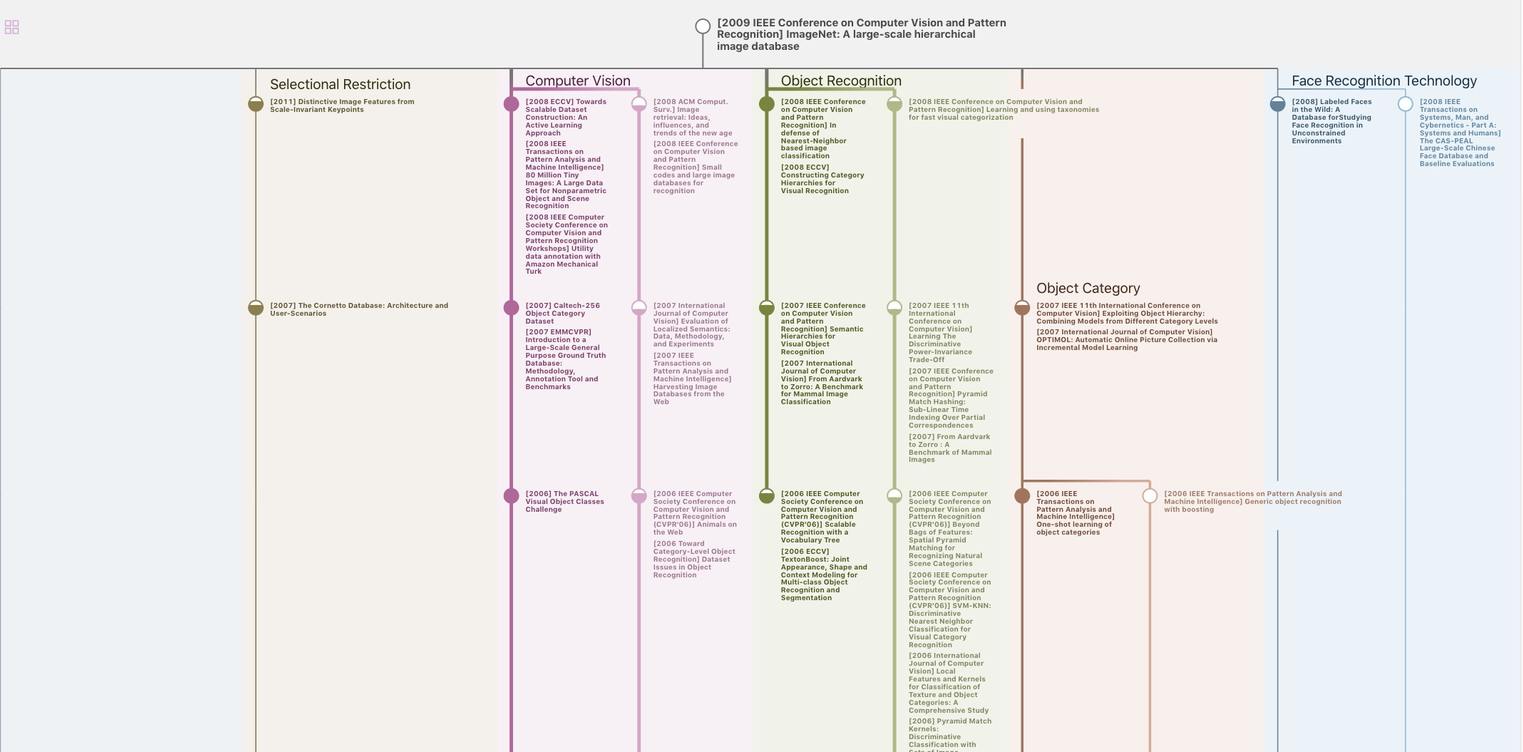
生成溯源树,研究论文发展脉络
Chat Paper
正在生成论文摘要