RewardTLG: Learning to Temporal Language Grounding from Flexible Reward
PROCEEDINGS OF THE 46TH INTERNATIONAL ACM SIGIR CONFERENCE ON RESEARCH AND DEVELOPMENT IN INFORMATION RETRIEVAL, SIGIR 2023(2023)
Abstract
Given a textual sentence provided by a user, the Temporal Language Grounding (TLG) task is defined as the process of finding a semantically relevant video moment or clip from an untrimmed video. In recent years, localization-based TLG methods have been explored, which adopt reinforcement learning to locate a clip from the video. However, these methods are not stable enough due to the stochastic exploration mechanism of reinforcement learning, which is sensitive to the reward. Therefore, providing a more flexible and reasonable reward has become a focus of attention for both academia and industry. Inspired by the training process of chatGPT, we innovatively adopt a vision-language pre-training (VLP) model as a reward model, which provides flexible rewards to help the localization-based TLG task converge. Specifically, a reinforcement learning-based localization module is introduced to predict the start and end timestamps in multi-modal scenarios. Thereafter, we fine-tune a reward model based on a VLP model, even introducing some human feedback, which provides a flexible reward score for the localization module. In this way, our model is able to capture subtle differences of the untrimmed video. Extensive experiments on two datasets have well verified the effectiveness of our proposed solution.
MoreTranslated text
Key words
Temporal Language Grounding,Cross-Modal Moment Retrieval
AI Read Science
Must-Reading Tree
Example
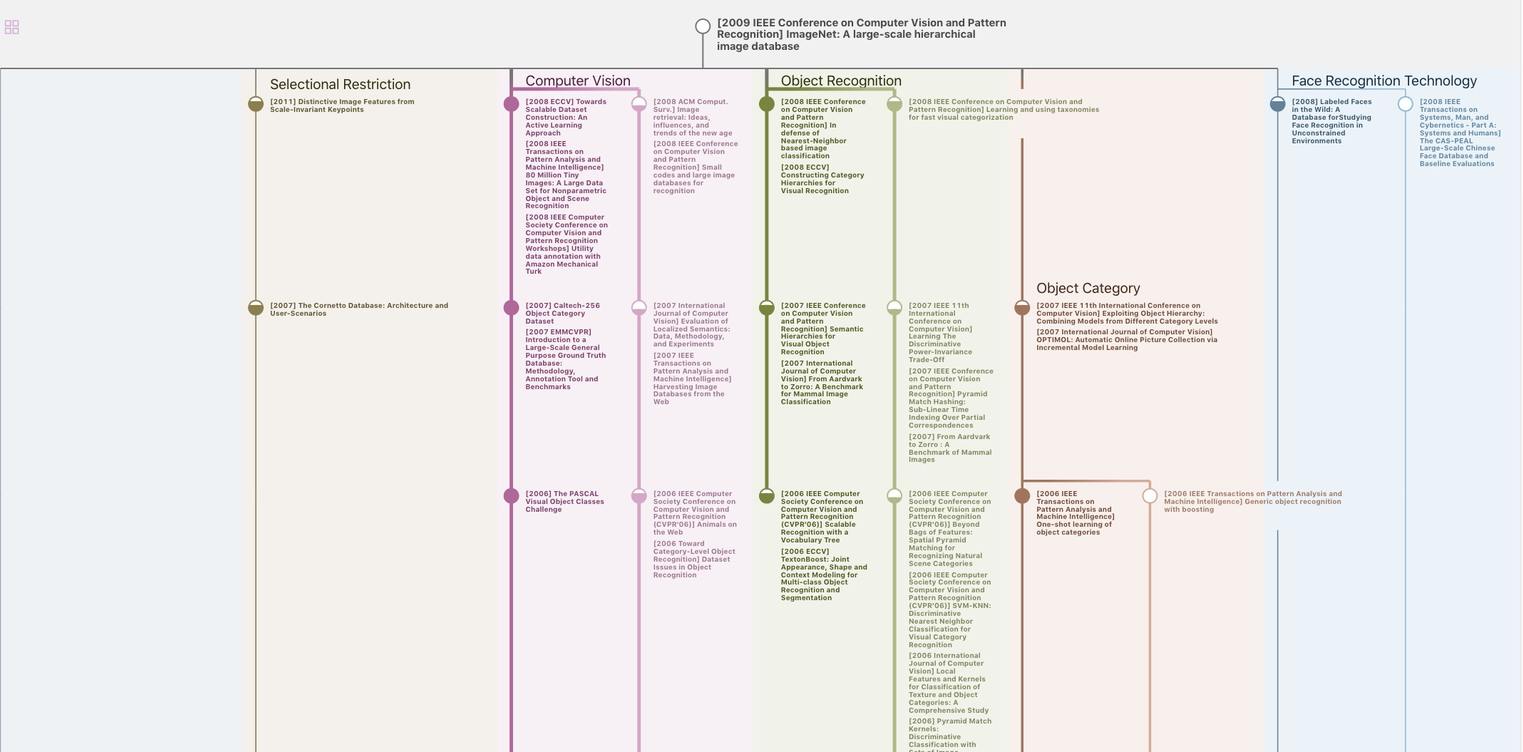
Generate MRT to find the research sequence of this paper
Chat Paper
Summary is being generated by the instructions you defined