Value-Aware Active Learning
HHAI 2023: AUGMENTING HUMAN INTELLECT(2023)
Abstract
In many practical applications, machine learning models are embedded into a pipeline involving a human actor that decides whether to trust the machine prediction or take a default route (e.g., classify the example herself). Selective classifiers have the option to abstain from making a prediction on an example they do not feel confident about. Recently, the notion of the value of a machine learning model has been introduced as a way to jointly consider the benefit of a correct prediction, the cost of an error, and that of abstaining. In this paper, we study how active learning of selective classifiers is affected by the focus on value. We show that the performance of the state-of-the-art active learning strategies drops significantly when we evaluate them based on value rather than accuracy. Finally, we propose a novel value-aware active learning strategy that outperforms the state-of-the-art ones when the cost of incorrect predictions substantially outweighs that of abstaining.
MoreTranslated text
Key words
active learning,value-based learning,cost-sensitive learning,selective classifier
AI Read Science
Must-Reading Tree
Example
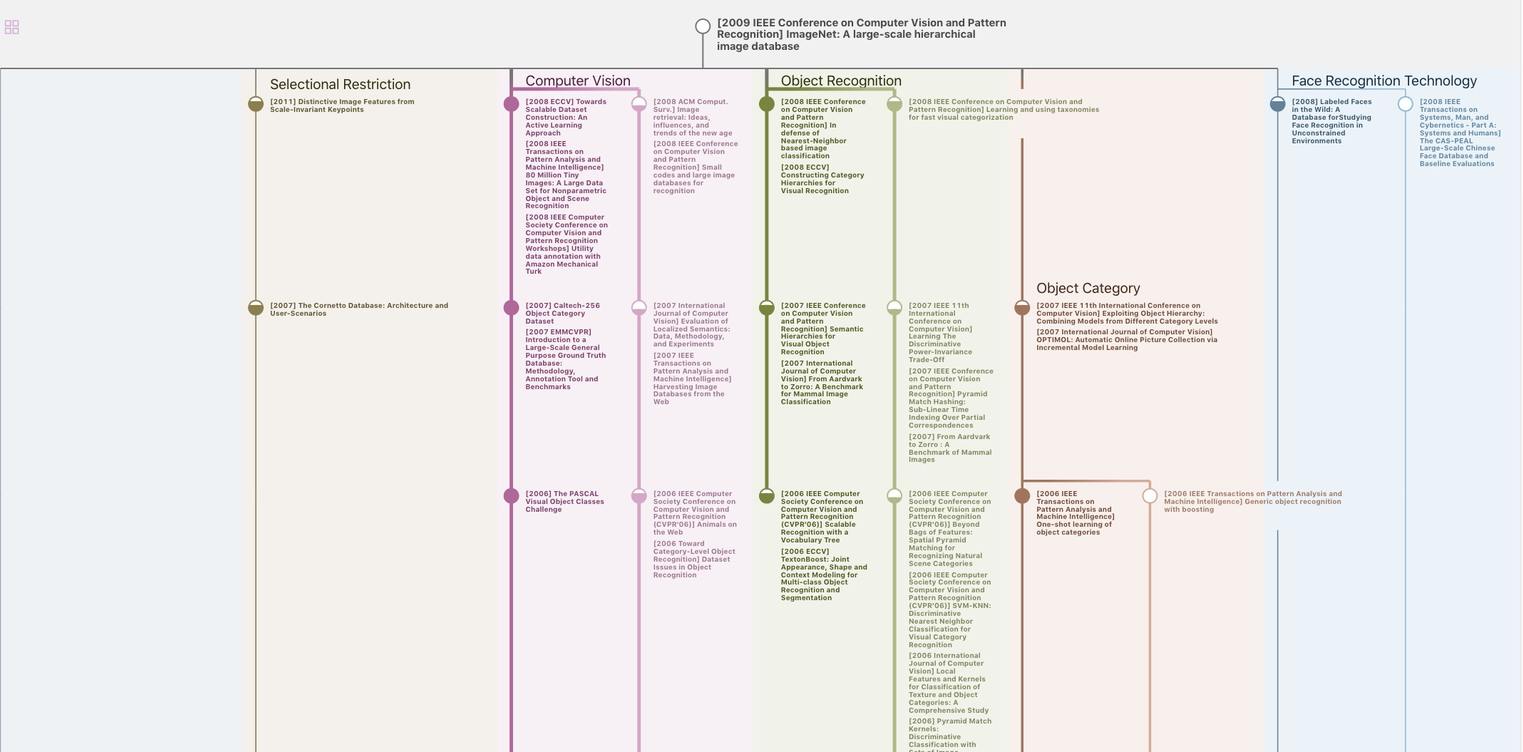
Generate MRT to find the research sequence of this paper
Chat Paper
Summary is being generated by the instructions you defined