EAYv3-CFC3: Ensemble Learning With Attention-Based Yv3 Combined With CFC3 Loss for Obscenity Detection
IEEE TRANSACTIONS ON EMERGING TOPICS IN COMPUTATIONAL INTELLIGENCE(2024)
摘要
A substantial amount of pornographic material is unregulated and readily accessible to all, which triggers cybercrime. Hence, the automatic detection and filtering of obscene content have become vital. Due to the sensitive nature of the data, a false-positive misclassification can affect the dignity of a person. So, we choose a detection algorithm that trains the model with annotated obscene images to specifically extract obscene features. We introduced a YOLOv3 ensemble learning method that integrates the sandglass block and convolutional block attention module (CBAM) into feature extraction. A diversified dataset is generated by using Pix-2-Pix GAN-based augmentation followed by extensive manual annotations to train this algorithm. The augmentation technique using illumination shifts and background color variations enables our model to reduce the misclassifications. The proposed design is optimized by using CFC3 loss, a combination of contrastive feature loss, and YOLOv3 default loss. We abbreviated this model as Ensemble learning with Attention-based YOLOv3 combined with CFC3 loss (EAYv3-CF}}C-3). EAYv3-CFC3 achieved an accuracy of 98.85 +/- 1.00%, a precision of 98.70 +/- 1.00%, a JI score of 97.73 +/- 1.00%, an FPR of 0.013 +/- 1.00%, an FM value of 98.85 +/- 1.00%, and MCC value of 97.70 +/- 1.30% with a better performance as compared to the state-of-the-art techniques.
更多查看译文
关键词
Obscene detection,attention learning,feature loss,YOLOv3,ensemble learning,deep learning
AI 理解论文
溯源树
样例
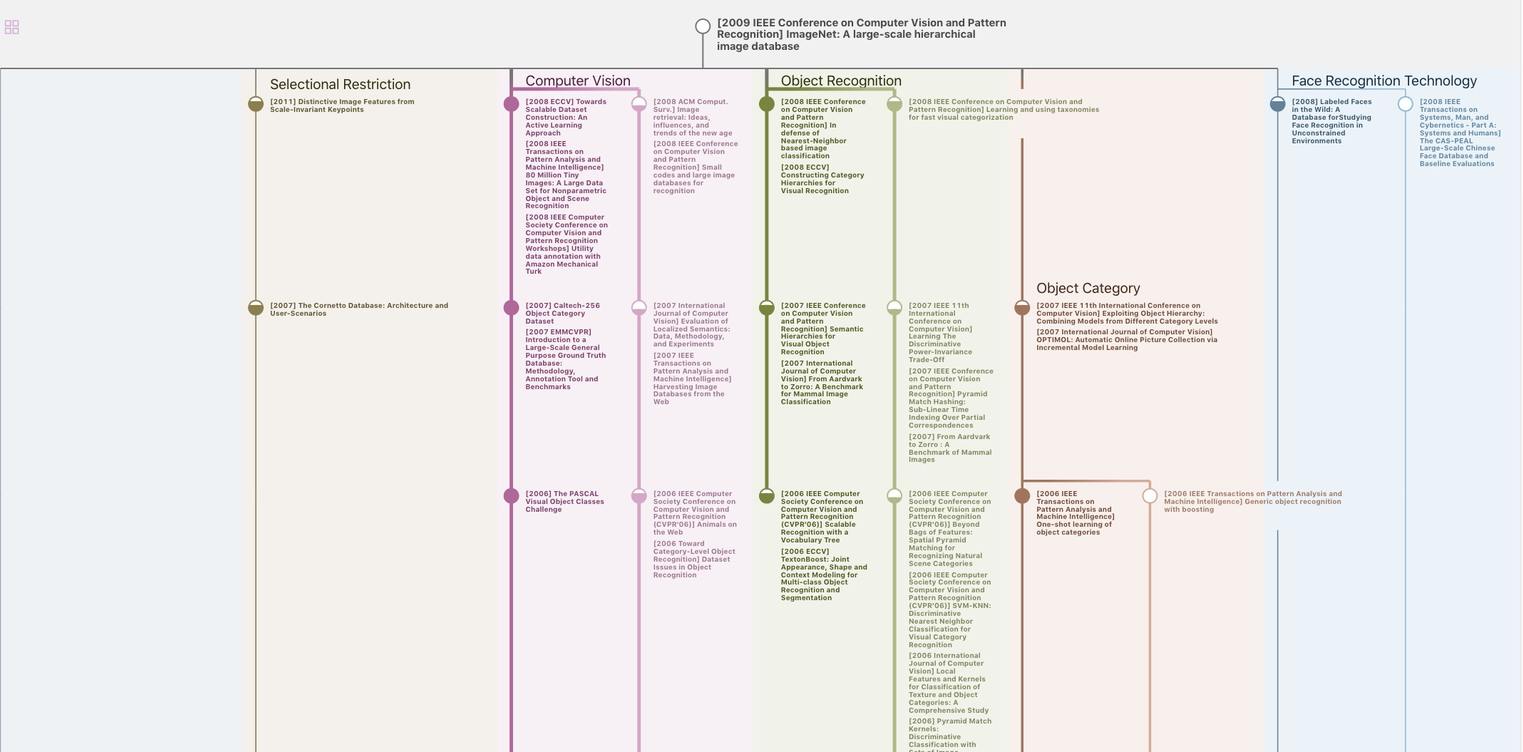
生成溯源树,研究论文发展脉络
Chat Paper
正在生成论文摘要