Renyi Divergence Deep Mutual Learning
MACHINE LEARNING AND KNOWLEDGE DISCOVERY IN DATABASES: RESEARCH TRACK, ECML PKDD 2023, PT II(2023)
摘要
This paper revisits Deep Mutual Learning (DML), a simple yet effective computing paradigm. We propose using Renyi divergence instead of the Kullback-Leibler divergence, which is more flexible and tunable, to improve vanilla DML. This modification is able to consistently improve performance over vanilla DML with limited additional complexity. The convergence properties of the proposed paradigm are analyzed theoretically, and Stochastic Gradient Descent with a constant learning rate is shown to converge with O(1)-bias in the worst case scenario for nonconvex optimization tasks. That is, learning will reach nearby local optima but continue searching within a bounded scope, which may help mitigate overfitting. Finally, our extensive empirical results demonstrate the advantage of combining DML and the Renyi divergence, leading to further improvement in model generalization.
更多查看译文
AI 理解论文
溯源树
样例
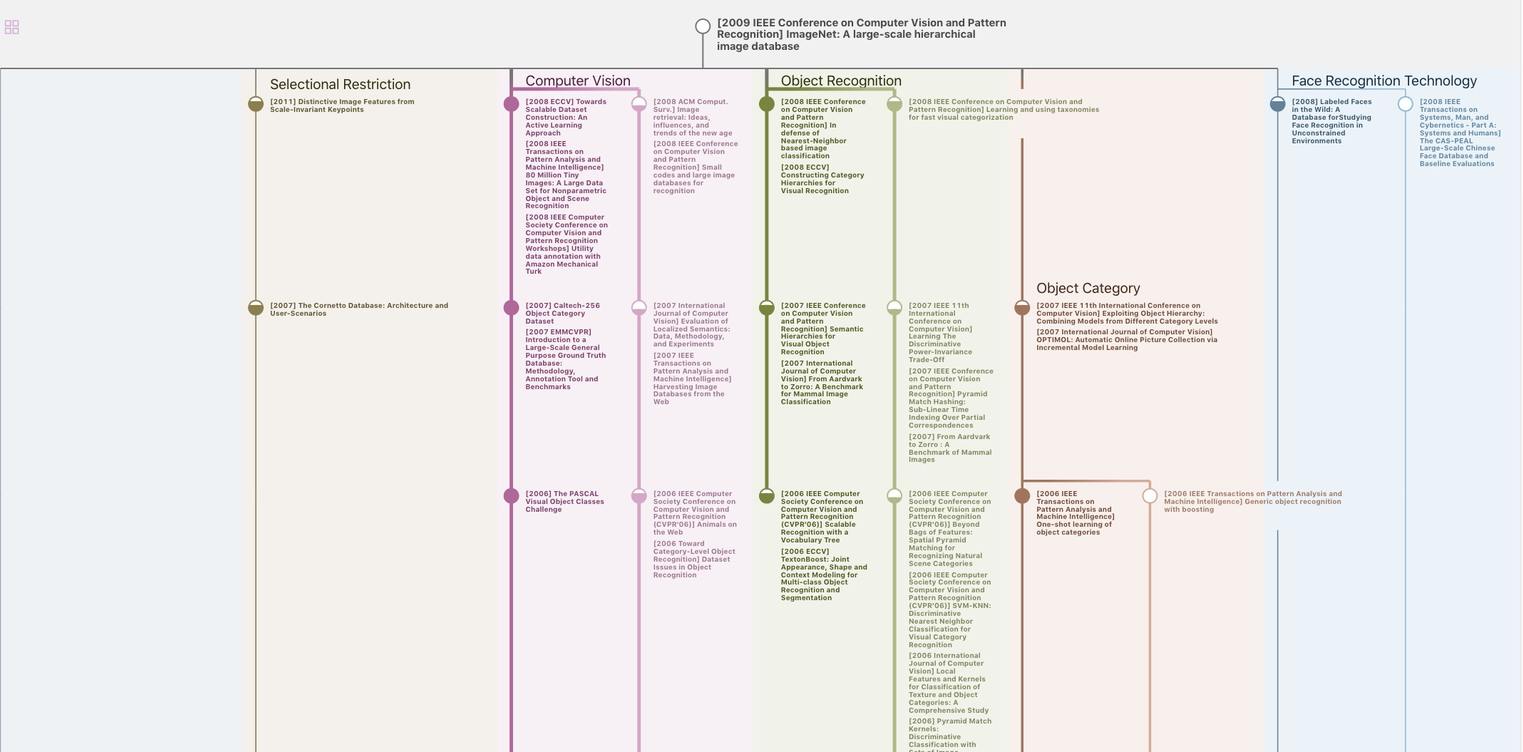
生成溯源树,研究论文发展脉络
Chat Paper
正在生成论文摘要