Linguistic-based Mild Cognitive Impairment detection using Informative Loss
Computers in Biology and Medicine(2024)
摘要
This paper presents a deep learning method using Natural Language Processing (NLP) techniques, to distinguish between Mild Cognitive Impairment (MCI) and Normal Cognitive (NC) conditions in older adults. We propose a framework that analyzes transcripts generated from video interviews collected within the I-CONECT study project, a randomized controlled trial aimed at improving cognitive functions through video chats. Our proposed NLP framework consists of two Transformer-based modules, namely Sentence Embedding (SE) and Sentence Cross Attention (SCA). First, the SE module captures contextual relationships between words within each sentence. Subsequently, the SCA module extracts temporal features from a sequence of sentences. This feature is then used by a Multi-Layer Perceptron (MLP) for the classification of subjects into MCI or NC. To build a robust model, we propose a novel loss function, called InfoLoss, that considers the reduction in entropy by observing each sequence of sentences to ultimately enhance the classification accuracy. The results of our comprehensive model evaluation using the I-CONECT dataset show that our framework can distinguish between MCI and NC with an average area under the curve of 84.75%.
更多查看译文
关键词
Mild Cognitive Impairment classification,Informative Loss function,Natural Language Processing,Transformers,Linguistic features detection,I-CONECT dataset
AI 理解论文
溯源树
样例
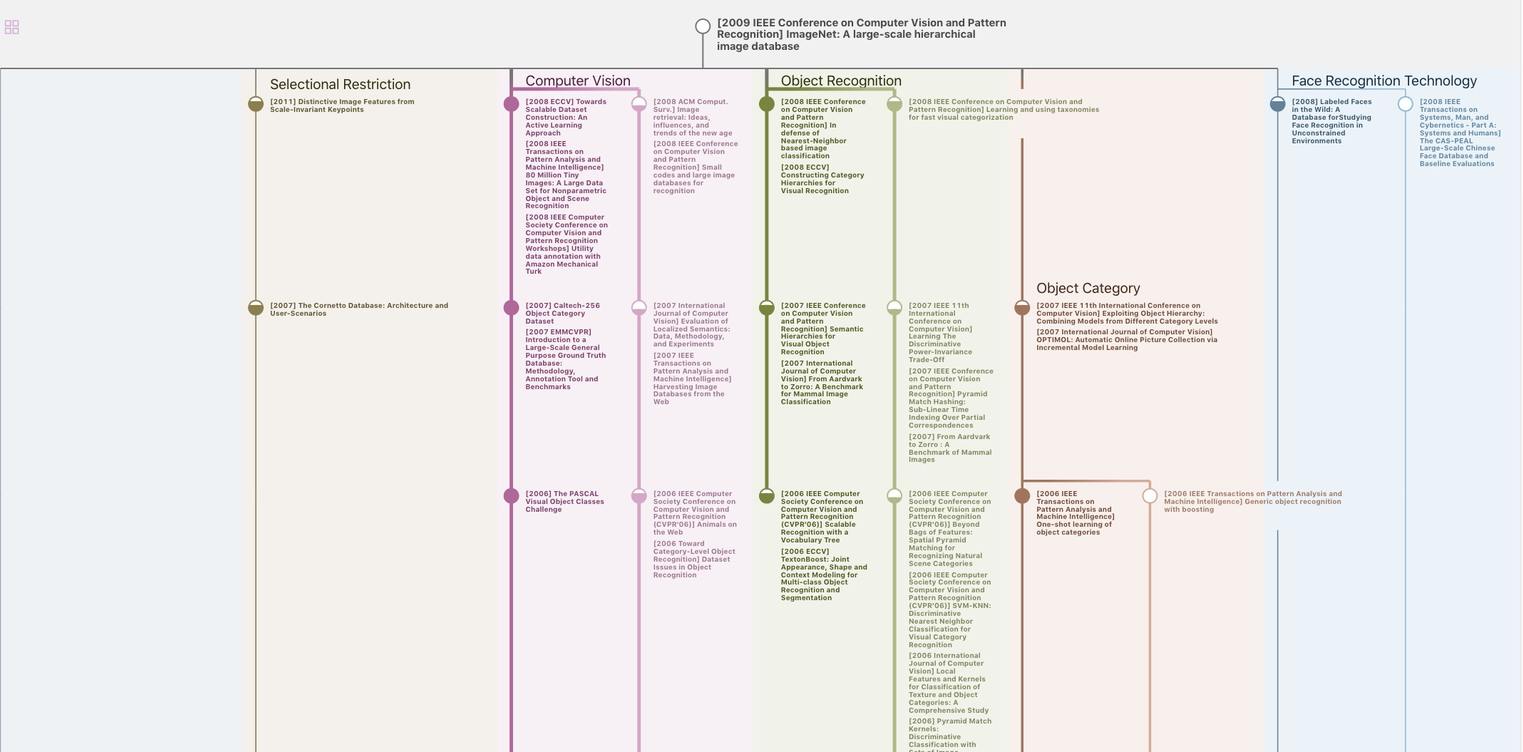
生成溯源树,研究论文发展脉络
Chat Paper
正在生成论文摘要