Energy-Based Domain-Adaptive Segmentation With Depth Guidance.
IEEE Robotics Autom. Lett.(2024)
Abstract
Recent endeavors have been made to leverage self-supervised depth estimation as guidance in unsupervised domain adaptation (UDA) for semantic segmentation. Prior arts, however, overlook the discrepancy between semantic and depth features, as well as the reliability of feature fusion, thus leading to suboptimal segmentation performance. To address this issue, we propose a novel UDA framework called
SMART
(cro
S
s do
M
ain sem
A
ntic segmentation based on ene
R
gy es
T
imation) that utilizes Energy-Based Models (EBMs) to obtain task-adaptive features and achieve reliable feature fusion for semantic segmentation with self-supervised depth estimates. Our framework incorporates two novel components: energy-based feature fusion (
EB2F
) and energy-based reliable fusion Assessment (
RFA
) modules. The EB2F module produces task-adaptive semantic and depth features by explicitly measuring and reducing their discrepancy using Hopfield energy for better feature fusion. The RFA module evaluates the reliability of the feature fusion using an energy score to improve the effectiveness of depth guidance. Extensive experiments on two datasets demonstrate that our method achieves significant performance gains over prior works, validating the effectiveness of our energy-based learning approach. The project page is available at
http://VLIS2022.github.io/SMART
.
MoreTranslated text
Key words
Unsupervised Domain Adaptation,Semantic Segmentation,Energy-based Model,Depth Estimation
AI Read Science
Must-Reading Tree
Example
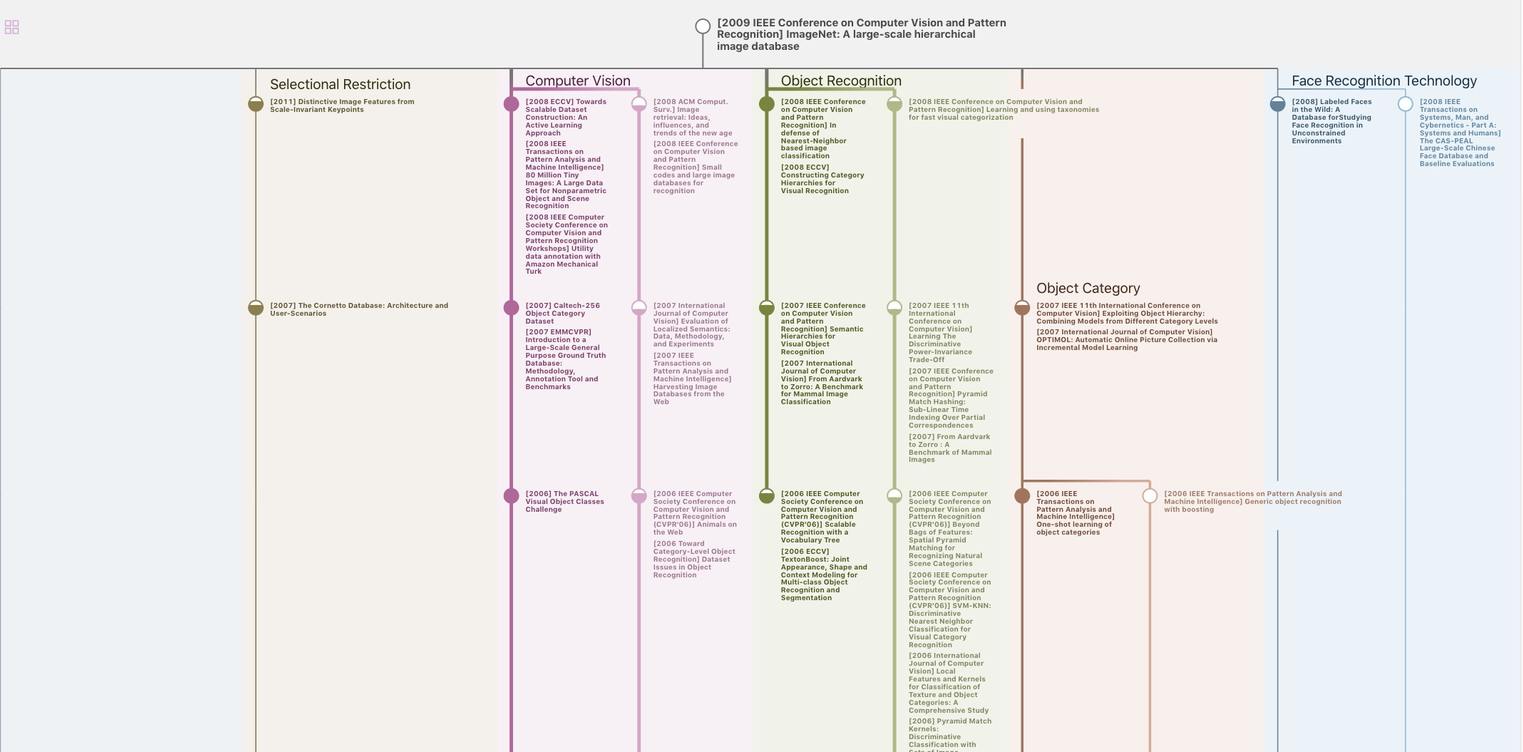
Generate MRT to find the research sequence of this paper
Chat Paper
Summary is being generated by the instructions you defined