An Interactive Knowledge-Based Multiobjective Evolutionary Algorithm Framework for Practical Optimization Problems
IEEE TRANSACTIONS ON EVOLUTIONARY COMPUTATION(2024)
Abstract
Experienced users often have useful knowledge and intuition in solving real-world optimization problems. User knowledge can be formulated as intervariable relationships to assist an optimization algorithm in finding good solutions faster. Such intervariable interactions can also be automatically learned from high-performing solutions discovered at intermediate iterations in an optimization run-a process called innovization. These relations, if vetted by the users, can be enforced among newly generated solutions to steer the optimization algorithm toward practically promising regions in the search space. Challenges arise for large-scale problems where the number of such variable relationships may be high. This article proposes an interactive knowledge-based evolutionary multiobjective optimization (IK-EMO) framework that extracts hidden variable-wise relationships as knowledge from evolving high-performing solutions, shares them with users to receive feedback, and applies them back to the optimization process to improve its effectiveness. The knowledge extraction process uses a systematic and elegant graph analysis method which scales well with the number of variables. The working of the proposed IK-EMO is demonstrated on three large-scale real-world engineering design problems. The simplicity and elegance of the proposed knowledge extraction process and the achievement of high-performing solutions quickly indicate the power of the proposed framework. The results presented should motivate further such interaction-based optimization studies for their routine use in practice.
MoreTranslated text
Key words
Optimization,Knowledge based systems,Data mining,Task analysis,Maintenance engineering,Self-organizing feature maps,Search problems,"Innovization",interactive optimization,knowedge extraction,multiobjective optimization,repair
AI Read Science
Must-Reading Tree
Example
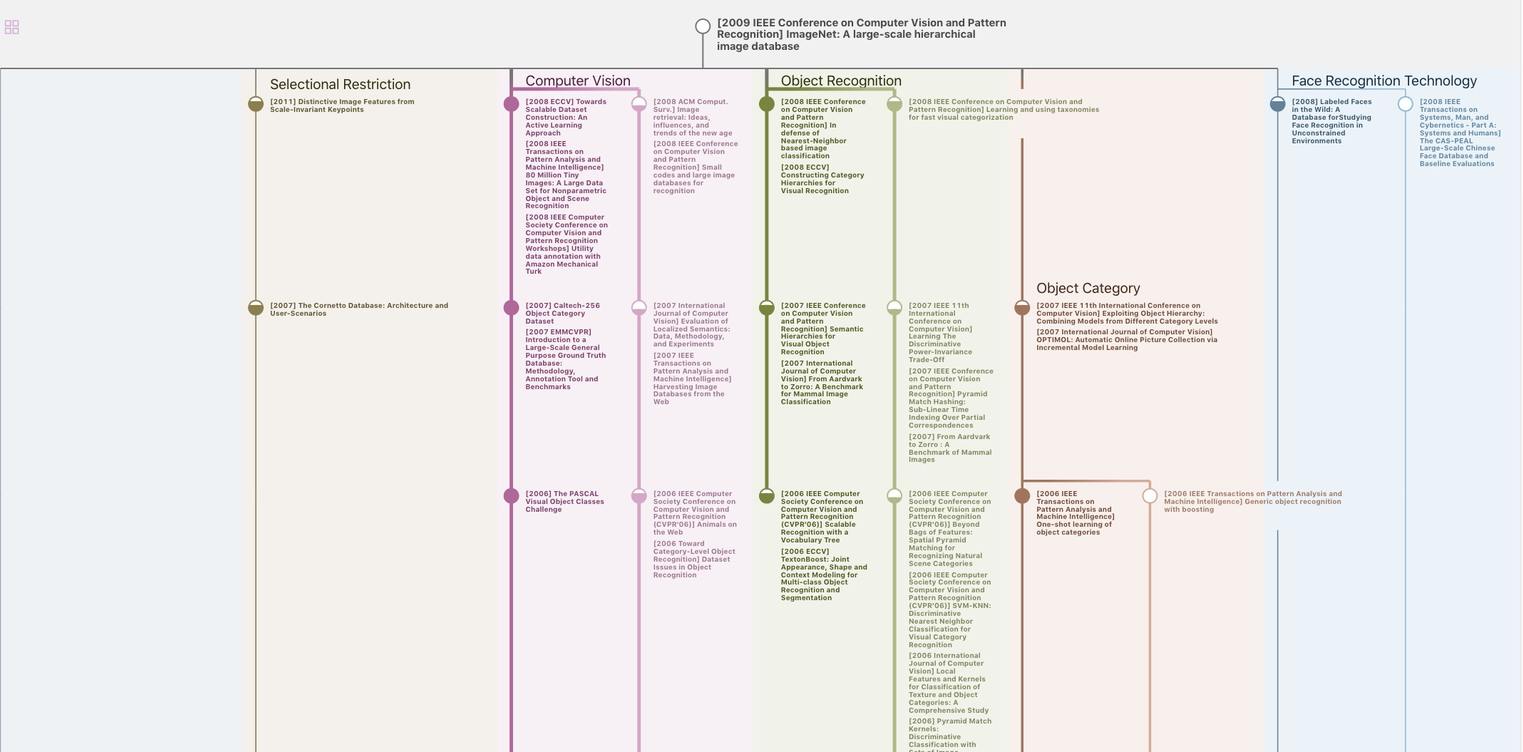
Generate MRT to find the research sequence of this paper
Chat Paper
Summary is being generated by the instructions you defined