Capturing High-Level Semantic Correlations via Graph for Multimodal Sentiment Analysis
IEEE SIGNAL PROCESSING LETTERS(2024)
摘要
Modeling intra-modal and cross-modal interactions poses significant challenges in multimodal sentiment analysis. Currently, graph-based methods like HGraph-CL achieve promising performance, which rely on two different levels of graph contrastive learning within and between modalities to explore sentiment correlations. However, HGraph-CL still faces the following drawbacks in graph construction: 1) nodes of the graph are represented at the frame level, only containing low-level information, neglecting the correlations among high-level semantics; 2) edges of the graph are based on the fixed dependency relations between words in the text sequence and the adjacent relations between frame-level nodes in the non-verbal sequences, failing to effectively capture implicit and long-distance correlations. To this end, this letter introduces capsule networks to construct high-level semantic nodes in a graph, uncovering deep sentimental structures. Furthermore, the learnable adjacency matrices are employed to construct edges of graph, thus adaptively learning the relations between nodes. Experimental results on several benchmark datasets for multimodal sentiment analysis demonstrate the effectiveness of the proposed method.
更多查看译文
关键词
Semantics,Routing,Correlation,Feature extraction,Visualization,Self-supervised learning,Videos,Multimodal sentiment analysis,cross-modal interactions,capsule networks,graph networks,high-level semantic correlations
AI 理解论文
溯源树
样例
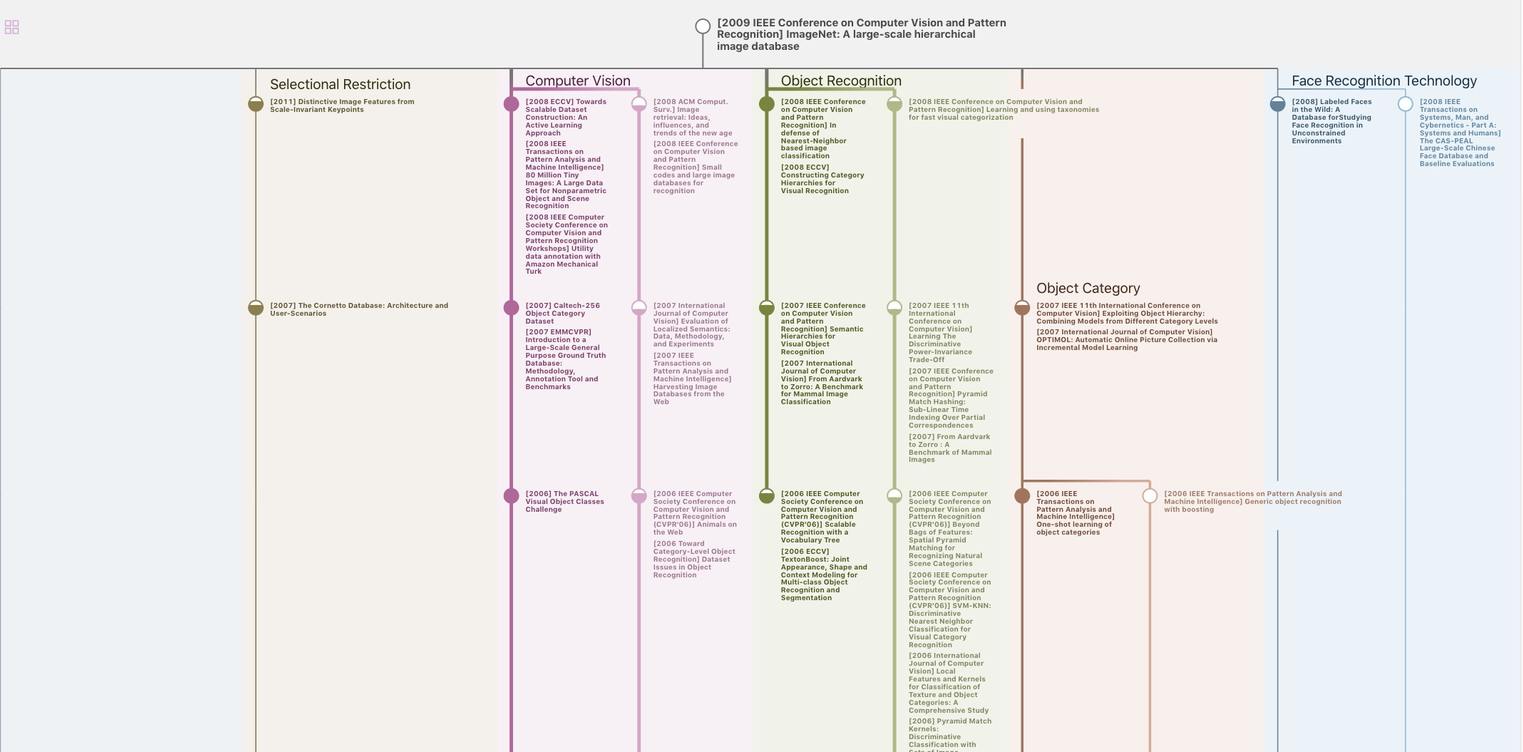
生成溯源树,研究论文发展脉络
Chat Paper
正在生成论文摘要