Assessing deep learning performance in power demand forecasting for smart grid
INTERNATIONAL JOURNAL OF SENSOR NETWORKS(2024)
摘要
This paper addresses the issue of forecasting power demands via deep learning (DL) techniques in smart grid (SG). Assessing proper DL models for power demand forecasting requires the consideration of factors (e.g., data pre-processing, computational resource usage, the complexity of learning models). We employ a two-tiered approach to carry out both short-term and long-term forecasting. Short-term forecasting emphasises model accuracy, while long-term forecasting assesses model robustness. Our evaluations utilise temporal fusion transformers (TFT) and the neural hierarchical interpolation for time series (N-HiTS)-based predictors, tested on a publicly available dataset. Our findings confirm that while TFT and N-HiTS perform similarly in short-term forecasting tasks, TFT displays superior robustness and accuracy in long-term forecasting tasks. The TFT model requires substantial computational resources, especially video RAM (VRAM), for a longer input data stream. Conversely, N-HiTS, though less confident in long-term forecasting, is shown to be more resource-efficient for handling longer input data streams.
更多查看译文
关键词
deep learning,smart grid,power demand forecasting,performance assessment,sensing and communication infrastructure
AI 理解论文
溯源树
样例
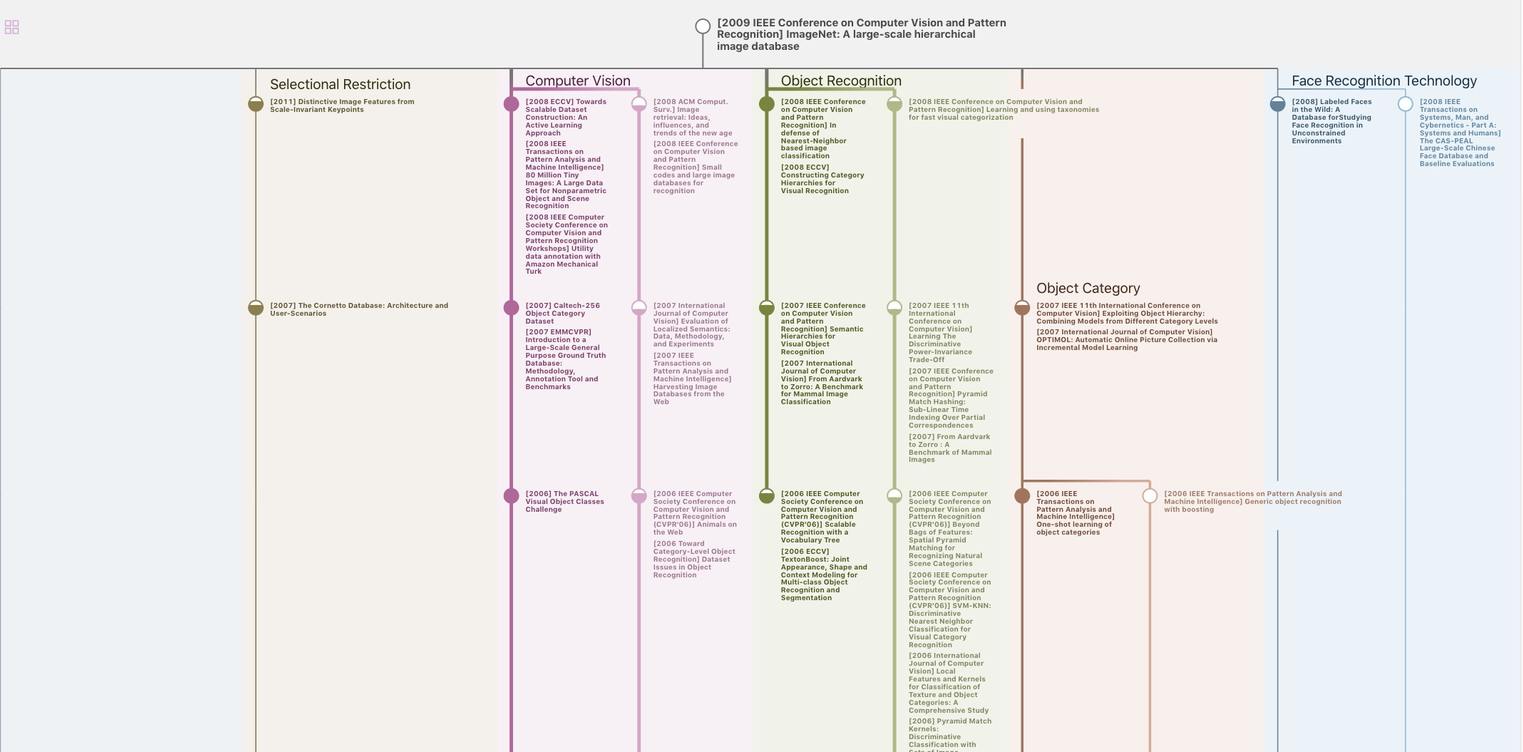
生成溯源树,研究论文发展脉络
Chat Paper
正在生成论文摘要