SeisCLIP: A Seismology Foundation Model Pre-Trained by Multimodal Data for Multipurpose Seismic Feature Extraction
IEEE TRANSACTIONS ON GEOSCIENCE AND REMOTE SENSING(2024)
摘要
In seismology, while training a specific deep learning model for each task is common, it often faces challenges such as the scarcity of labeled data and limited regional generalization. Addressing these issues, we introduce SeisCLIP: a foundation model for seismology, leveraging contrastive learning during pretraining on multimodal data of seismic waveform spectra and the corresponding local and global event information. SeisCLIP consists of a transformer-based spectrum encoder and an multilayer perceptron (MLP)-based information encoder that are jointly pre-trained on massive data. During pretraining, contrastive learning aims to enhance representations by training two encoders to bring the corresponding waveform spectra and event information closer in the feature space, while distancing uncorrelated pairs. Remarkably, the pre-trained spectrum encoder offers versatile features, enabling its application across diverse tasks and regions. Thus, it requires only modest datasets for fine-tuning to specific downstream tasks. Our evaluations demonstrate SeisCLIP's superior performance over baseline methods in tasks like event classification, localization, and focal mechanism analysis, even when using distinct datasets from various regions. In essence, SeisCLIP emerges as a promising foundational model for seismology, potentially revolutionizing foundation-model-based research in the domain.
更多查看译文
关键词
Task analysis,Data models,Training,Seismology,Earthquakes,Transformers,Self-supervised learning,Contrastive learning,event classification,focal mechanism analysis,location,seismology foundation model
AI 理解论文
溯源树
样例
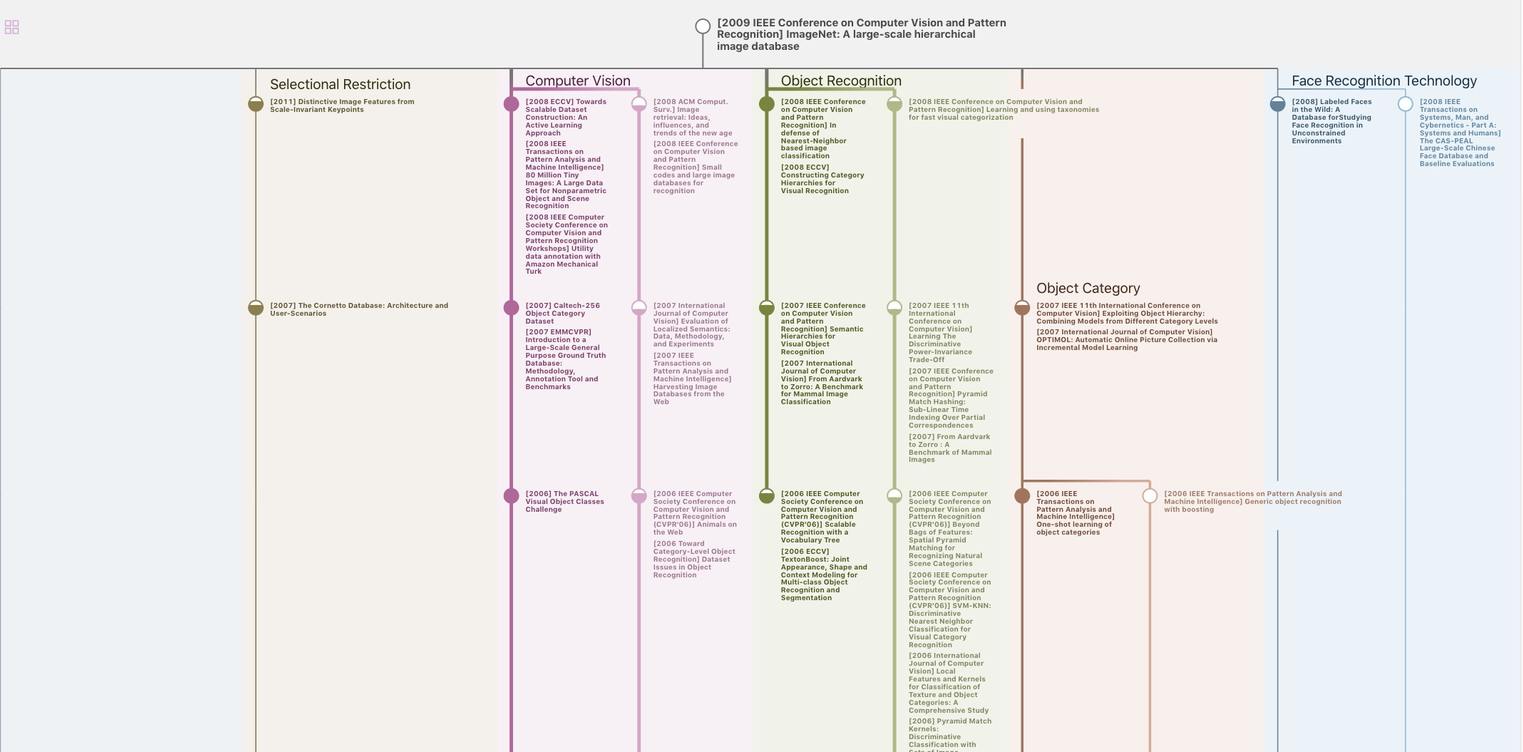
生成溯源树,研究论文发展脉络
Chat Paper
正在生成论文摘要