MCD-Net: Toward RGB-D Video Inpainting in Real-World Scenes
IEEE TRANSACTIONS ON IMAGE PROCESSING(2024)
摘要
Video inpainting gains an increasing amount of attention ascribed to its wide applications in intelligent video editing. However, despite tremendous progress made in RGB video inpainting, the existing RGB-D video inpainting models are still incompetent to inpaint real-world RGB-D videos, as they simply fuse color and depth via explicit feature concatenation, neglecting the natural modality gap. Moreover, current RGB-D video inpainting datasets are synthesized with homogeneous and delusive RGB-D data, which is far from real-world application and cannot provide comprehensive evaluation. To alleviate these problems and achieve real-world RGB-D video inpainting, on one hand, we propose a Mutually-guided Color and Depth Inpainting Network (MCD-Net), where color and depth are reciprocally leveraged to inpaint each other implicitly, mitigating the modality gap and fully exploiting cross-modal association for inpainting. On the other hand, we build a Video Inpainting with Depth (VID) dataset to supply diverse and authentic RGB-D video data with various object annotation masks to enable comprehensive evaluation for RGB-D video inpainting under real-world scenes. Experimental results on the DynaFill benchmark and our collected VID dataset demonstrate our MCD-Net not only yields the state-of-the-art quantitative performance but successfully achieves high-quality RGB-D video inpainting under real-world scenes. All resources are available at https://github.com/JCATCV/MCD-Net.
更多查看译文
关键词
Video inpainting,RGB-D inpainting,multi-modal learning,RGB-D object removal,RGB-D video dataset
AI 理解论文
溯源树
样例
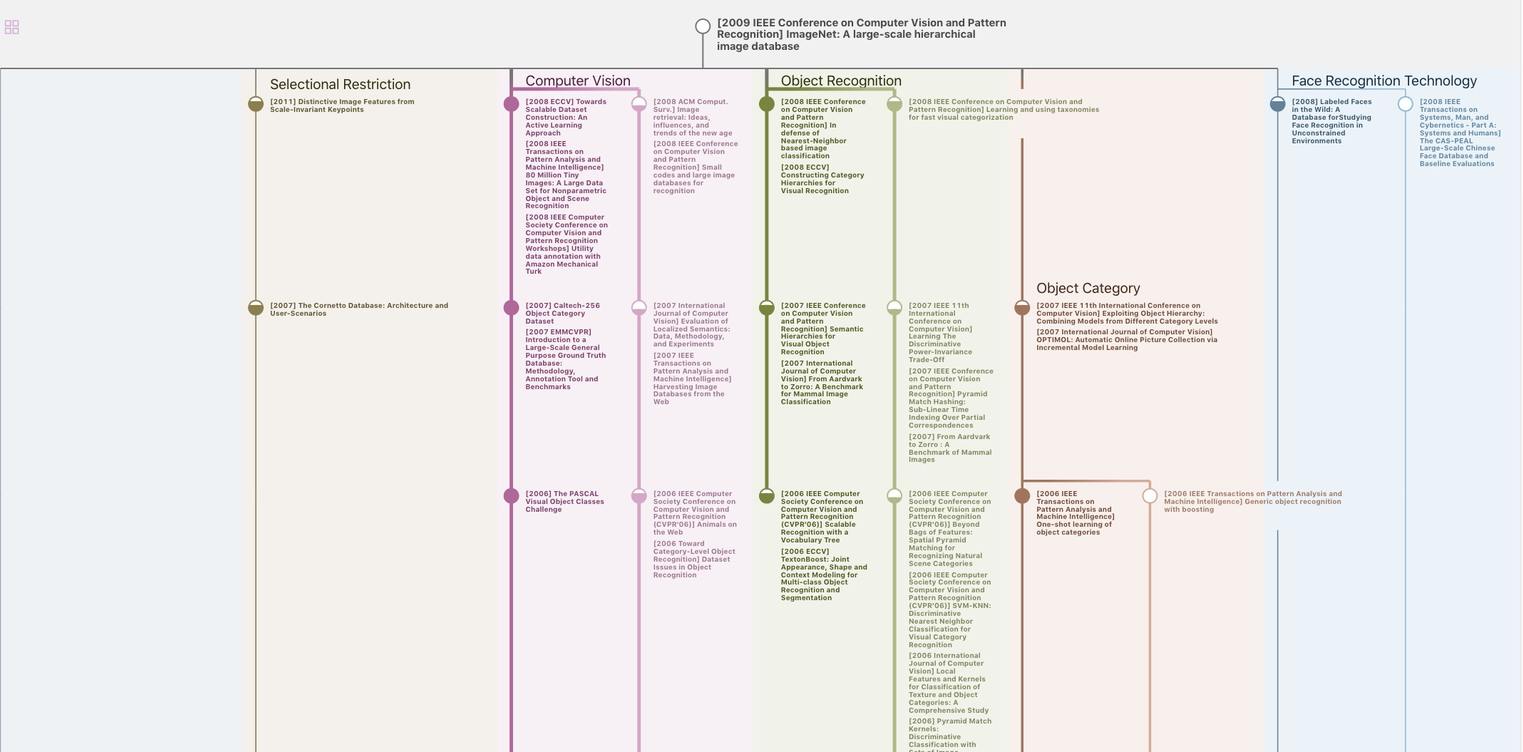
生成溯源树,研究论文发展脉络
Chat Paper
正在生成论文摘要