Sparse Bayesian Learning-Based 3-D Radio Environment Map Construction-Sampling Optimization, Scenario-Dependent Dictionary Construction, and Sparse Recovery
IEEE TRANSACTIONS ON COGNITIVE COMMUNICATIONS AND NETWORKING(2024)
摘要
The radio environment map (REM), which can visualize the information of invisible electromagnetic spectrum, is vital for monitoring, management, and security of spectrum resources in cognitive radio (CR) networks. In view of a limited number of spectrum sensors and constrained sampling time, this paper presents a new three-dimensional (3D) REM construction scheme based on sparse Bayesian learning (SBL). Firstly, we construct a scenario-dependent channel dictionary matrix by considering the propagation characteristic of the interested scenario. To improve sampling efficiency, a maximum mutual information (MMI)-based optimization algorithm is developed for the layout of sampling sensors. Then, a maximum and minimum distance (MMD) clustering-based SBL algorithm is proposed to recover the spectrum data at the unsampled positions and construct the whole 3D REM. We finally use the simulation data of the campus scenario to construct the 3D REMs and compare the proposed method with the state-of-the-art. The recovery performance and the impact of different parameters on the constructed REMs are also analyzed. Numerical results show that the proposed scheme can reduce the required spectrum data and has higher accuracy under the low sampling rate.
更多查看译文
关键词
Three-dimensional displays,Sensors,Sparse matrices,Dictionaries,Transmitters,Optimization,Data models,3D radio environment map,sparse Bayesian learning,mutual information,channel propagation model,clustering algorithm
AI 理解论文
溯源树
样例
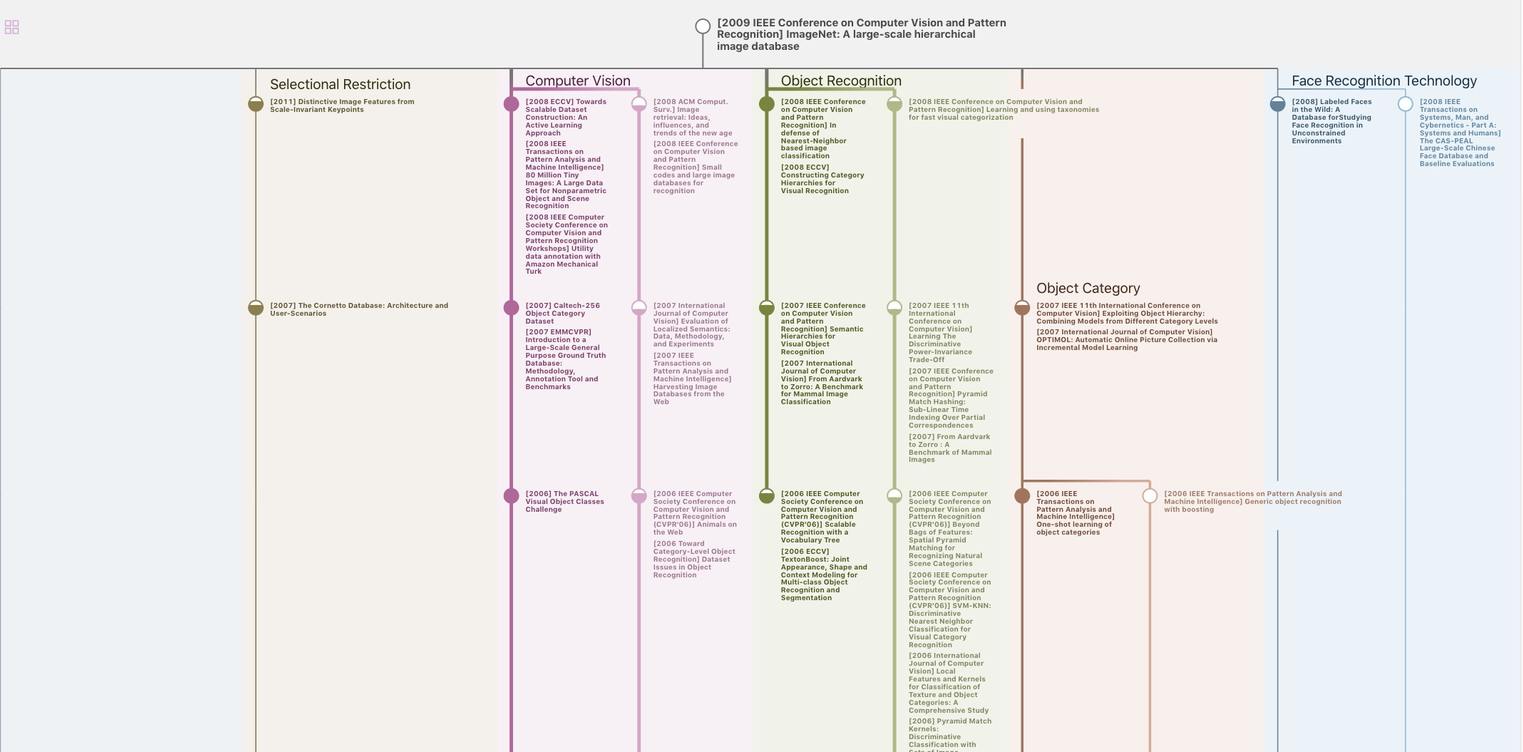
生成溯源树,研究论文发展脉络
Chat Paper
正在生成论文摘要