UNM: A Universal Approach for Noisy Multi-label Learning
IEEE Transactions on Knowledge and Data Engineering(2024)
摘要
Multi-label image classification relies on a large-scale, well-maintained dataset, which may easily be mislabeled due to various subjective reasons. Existing methods for coping with noise usually focus on improving the model robustness in the case of single-label noise. However, compared with noisy single-label learning, noisy multi-label learning is more practical and challenging. To reduce the negative impact of noisy multi-annotations, we propose a universal approach for noisy multi-label learning (UNM). In UNM, we propose the label-wise embedding network which investigates the semantic alignment between label embeddings and their corresponding output features to learn robust feature representations. Meanwhile, mining the co-occurrence of multi-labels is also added to regularize the noisy network predictions. We cyclically change the fitting status of our label-wise embedding network to distinguish the noisy samples and generate pseudo labels for them. As a result, UNM provides an effective way to exploit the label-wise features and semantic label embeddings in noisy scenarios. To verify the generalizability of our method, we also test our method on Partial Multi-label Learning (PML) and Multi-label Learning with Missing Labels (MLML). Extensive experiments on benchmark datasets including Microsoft COCO, Pascal VOC, and Visual Genome explicitly validate the proposed method.
更多查看译文
关键词
noisy labels,multi-label classification,label refinement
AI 理解论文
溯源树
样例
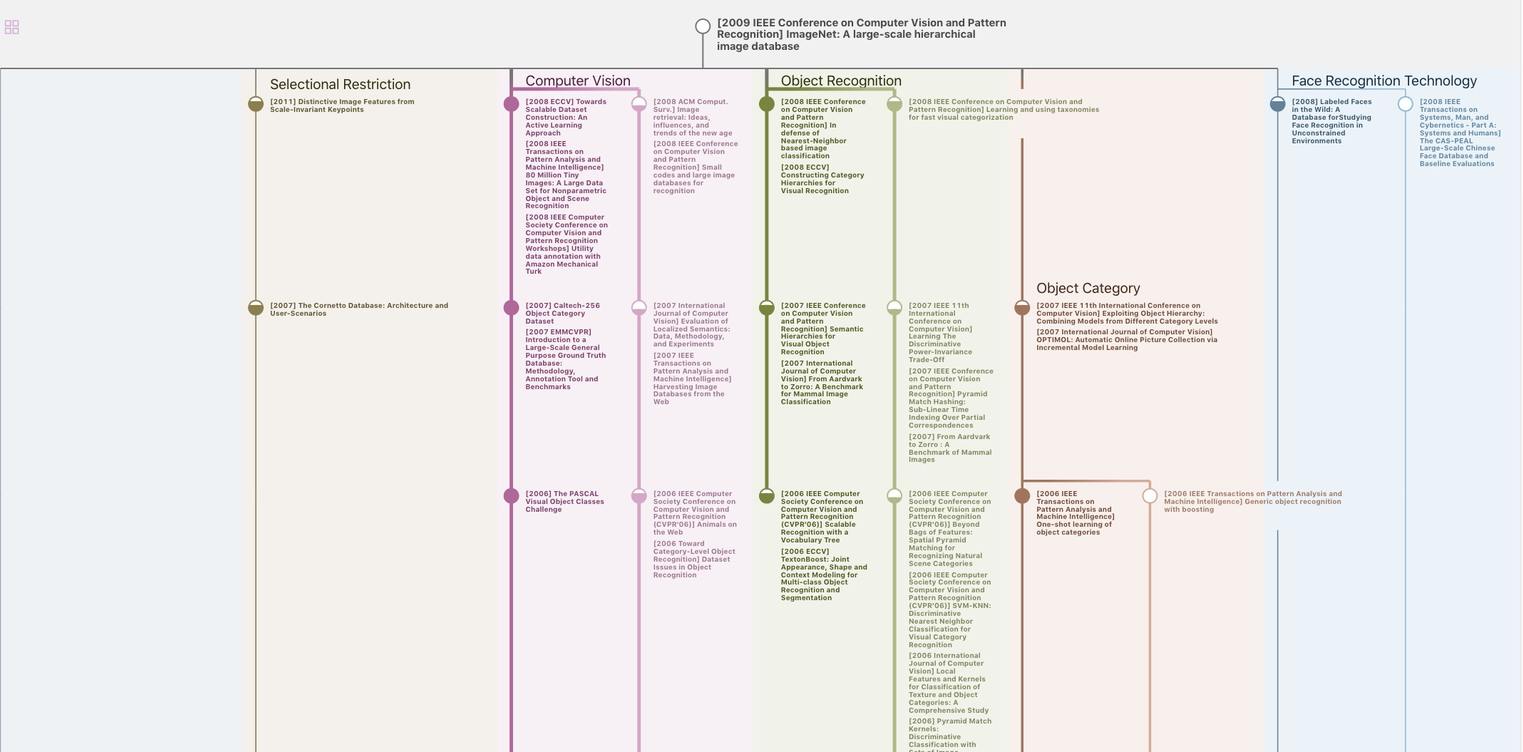
生成溯源树,研究论文发展脉络
Chat Paper
正在生成论文摘要