Predicting soil organic carbon with different approaches and spatial resolutions for the southern Iberian Peninsula, Spain
Geoderma Regional(2024)
摘要
Quantification and monitoring of soil organic carbon (SOC) stocks across local-to-global scales are needed to assess soil resource management practices and adapt environmental policies. Multiple SOC estimates are available worldwide; however, verification and validation are required to quantify the discrepancies and provide improved estimates. Here, we evaluated four different digital soil mapping (DSM) approaches (i.e., linear models, support vector machine, random forest, and quantile regression forest) to estimate SOC concentration (SOCc) and SOC stocks (SOCs) in the Region of Murcia (11,313 km2), a complex topographic and climatic area in the southern Iberian Peninsula, at three spatial resolutions (100, 250, and 1000 m). We estimated SOC spatially using a local harmonized database of 255 soil profiles for modeling and 1100 topsoils for external validation. We found that the quantile regression forest (QRF) approach had the best data-model agreement at 100 m spatial resolution, with the highest accuracy percentage (79%), external validation (correlation coefficient of 52%), and spatial interpretability of patterns, especially for SOCc. The QRF model showed a mean SOCc of 12.18 g/kg with an overall uncertainty of 10.54 g/kg and an accuracy percentage of 79%, whereas the total SOCs was 27,572 GgC with an uncertainty of 0.016 GgC. Our results showed that using local environmental covariates and local soil information to predict SOC within this region resulted in a relative improvement in the prediction accuracy of ~40% for SOCc and ~ 65% for SOCs compared to the SOC products derived from national and global databases. Our results showed a large discrepancy between the national and global estimates for reporting SOC locally. Consequently, local-to-regional efforts are needed to describe SOC spatial variability better to reduce uncertainty and improve the assessment of soil resources. We provide the resulting SOC maps with associated spatial uncertainty on the public Environmental Data Initiative Repository at https://portal.edirepository.org/nis/mapbrowse?packageid=edi.1238.2.
更多查看译文
关键词
Digital soil mapping,SOC concentration,SOC stock,Quantile regression forest (QRF)
AI 理解论文
溯源树
样例
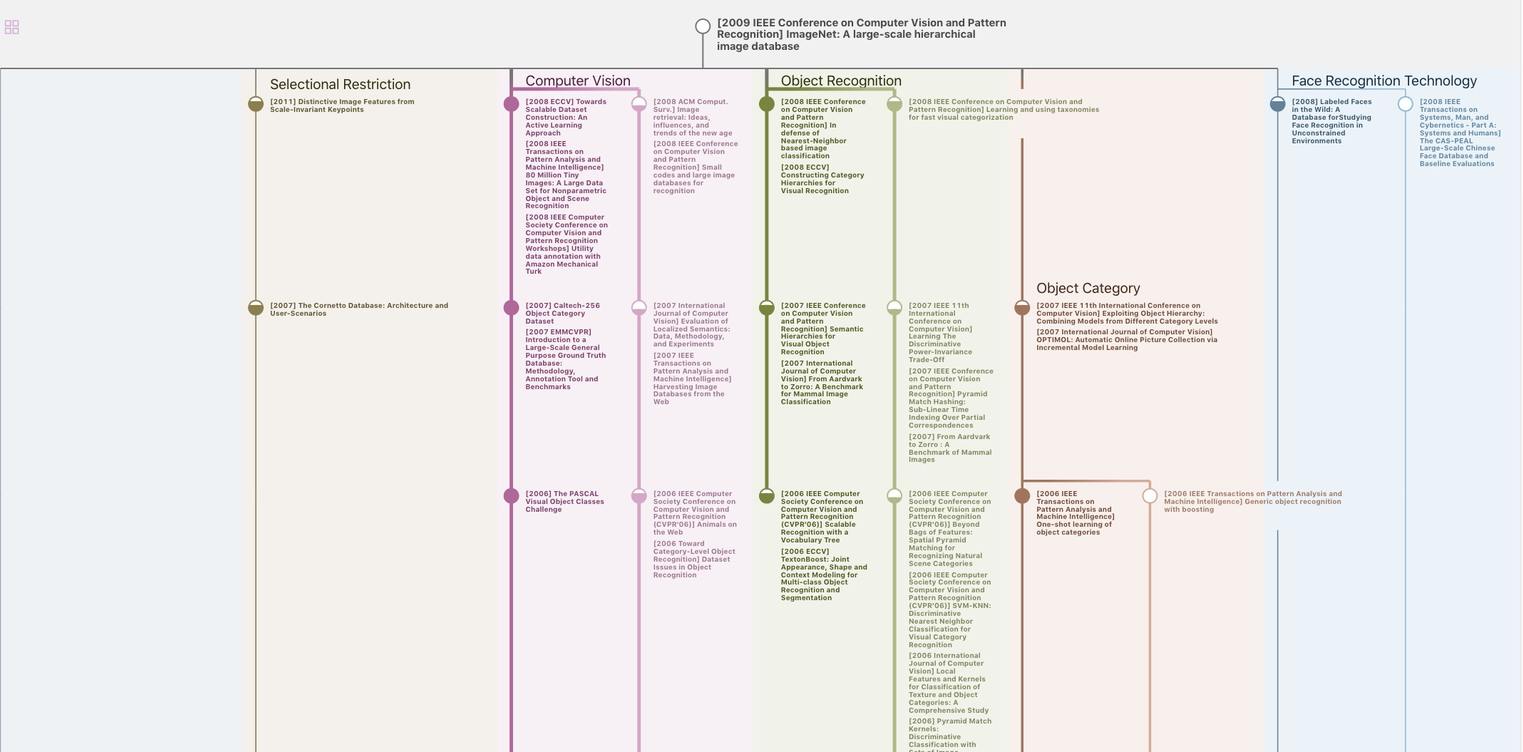
生成溯源树,研究论文发展脉络
Chat Paper
正在生成论文摘要