A Deep Learning Framework for Suppressing Prestack Seismic Random Noise Without Noise-Free Labels
Energy geoscience(2024)
摘要
Random noise attenuation is significant in seismic data processing. Supervised deep learning-based denoising methods have been widely developed and applied in recent years. In practice, it is often time-consuming and laborious to obtain noise-free data for supervised learning. Therefore, we propose a novel deep learning framework to denoise prestack seismic data without clean labels, which trains a high-resolution residual neural network (SRResnet) with noisy data for input and the same valid data with different noise for output. Since valid signals in noisy sample pairs are spatially correlated and random noise is spatially independent and unpredictable, the model can learn the features of valid data while suppressing random noise. Noisy data targets are generated by a simple conventional method without fine-tuning parameters. The initial estimates allow signal or noise leakage as the network does not require clean labels. The Monte Carlo strategy is applied to select training patches for increasing valid patches and expanding training datasets. Transfer learning is used to improve the generalization of real data processing. The synthetic and real data tests perform better than the commonly used state-of-the-art denoising methods.
更多查看译文
关键词
Data processing,Denoising,Signal processing,Seismics,Deep learning
AI 理解论文
溯源树
样例
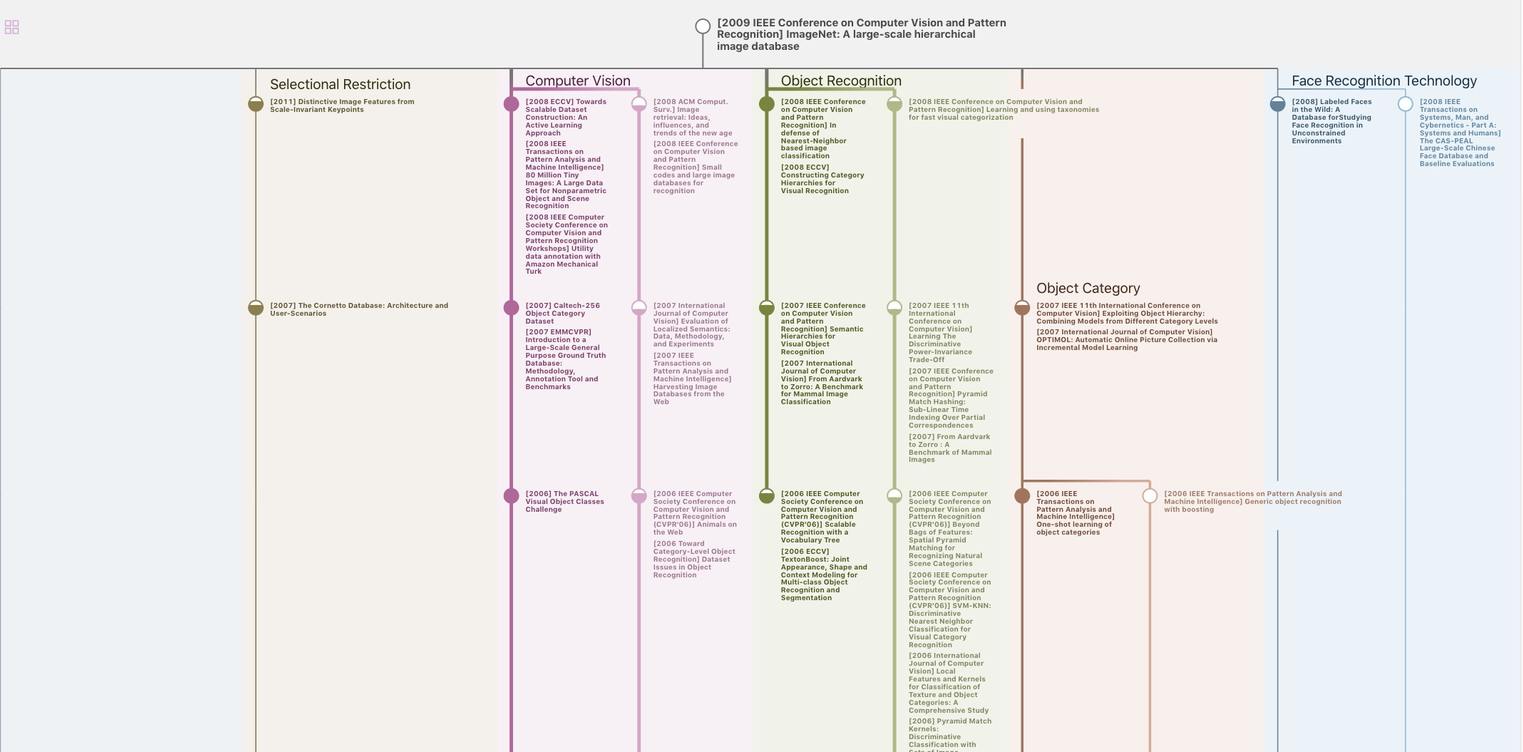
生成溯源树,研究论文发展脉络
Chat Paper
正在生成论文摘要