Scattering Attribute Embedded Network for Few-Shot SAR ATR
IEEE Transactions on Aerospace and Electronic Systems(2024)
摘要
Restricted by the deficient training samples, deep learning-based automatic target recognition (ATR) methods for synthetic aperture radar (SAR) are prone to performance degradation of target recognition with extremely few samples, making them difficult to apply in practical scenarios. To address this issue, we propose a scattering attribute embedded network (SANet) for few-shot SAR ATR. SANet embeds inherent physical attributes provided by attribute scattering centers of SAR targets to enable effective generalization to novel-class targets unseen in the training set with only an extremely small number of samples. The method employs a two-stream architecture, comprising attribute induced global semantic extraction and graph-based structure representation learning, which are optimized to enhance intra-class compactness and inter-class discriminability to achieve physics embedded few-shot SAR target recognition. Several few-shot recognition experiments are conducted using the MSTAR dataset of different depression angles. The experimental results demonstrate that the proposed SANet significantly outperforms state-of-the-art few-shot learning methods in terms of SAR target recognition accuracy. Additionally, SANet exhibits better generalization and robustness across different depression angles and occlusions in SAR images, which shows a great potential for practical applications.
更多查看译文
关键词
Scattering attribute embedding,automatic target recognition (ATR),few shot learning (FSL),synthetic aperture radar (SAR)
AI 理解论文
溯源树
样例
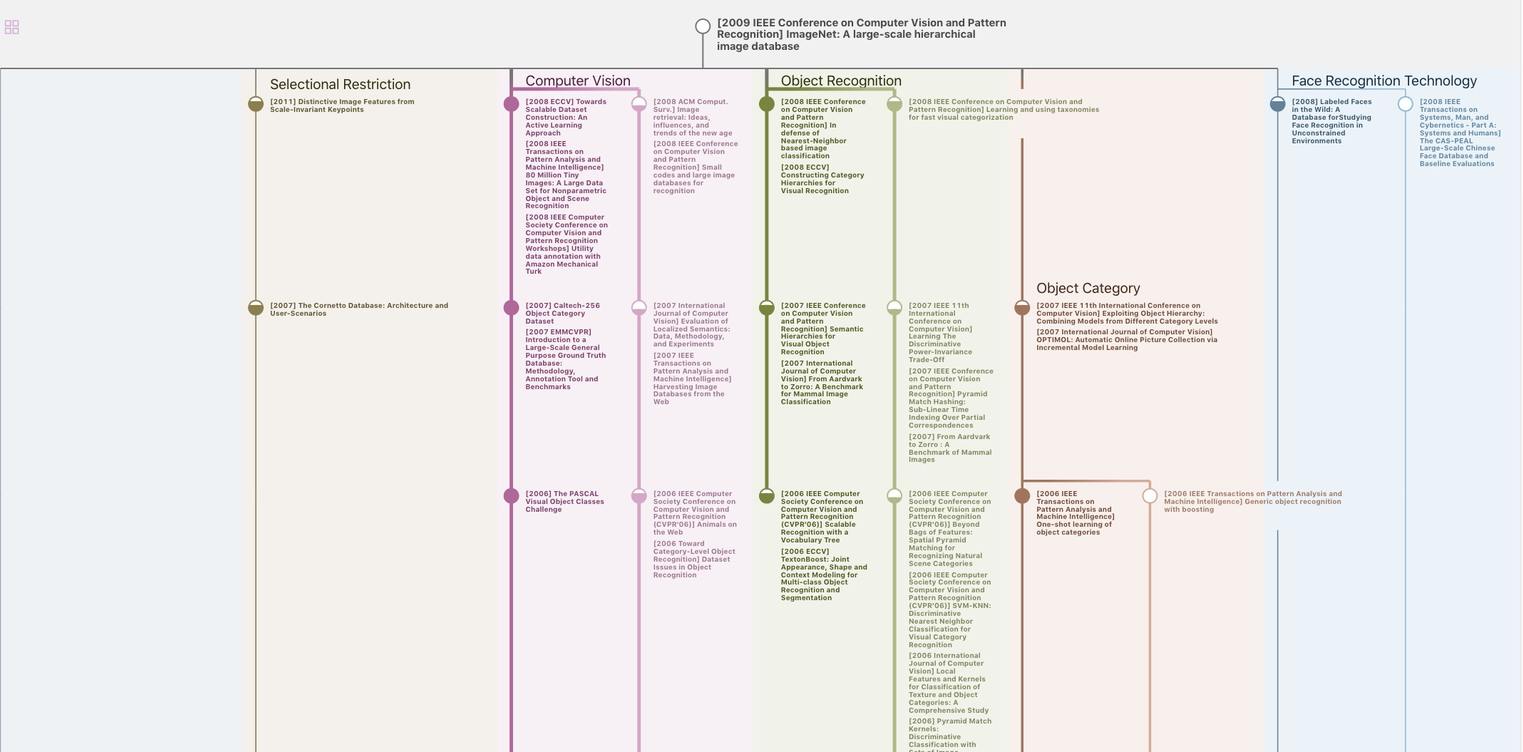
生成溯源树,研究论文发展脉络
Chat Paper
正在生成论文摘要