A Unified Inexact Stochastic ADMM for Composite Nonconvex and Nonsmooth Optimization
arxiv(2024)
摘要
In this paper, we propose a unified framework of inexact stochastic
Alternating Direction Method of Multipliers (ADMM) for solving nonconvex
problems subject to linear constraints, whose objective comprises an average of
finite-sum smooth functions and a nonsmooth but possibly nonconvex function.
The new framework is highly versatile. Firstly, it not only covers several
existing algorithms such as SADMM, SVRG-ADMM, and SPIDER-ADMM but also guides
us to design a novel accelerated hybrid stochastic ADMM algorithm, which
utilizes a new hybrid estimator to trade-off variance and bias. Second, it
enables us to exploit a more flexible dual stepsize in the convergence
analysis. Under some mild conditions, our unified framework preserves
𝒪(1/T) sublinear convergence. Additionally, we establish the linear
convergence under error bound conditions. Finally, numerical experiments
demonstrate the efficacy of the new algorithm for some nonsmooth and nonconvex
problems.
更多查看译文
AI 理解论文
溯源树
样例
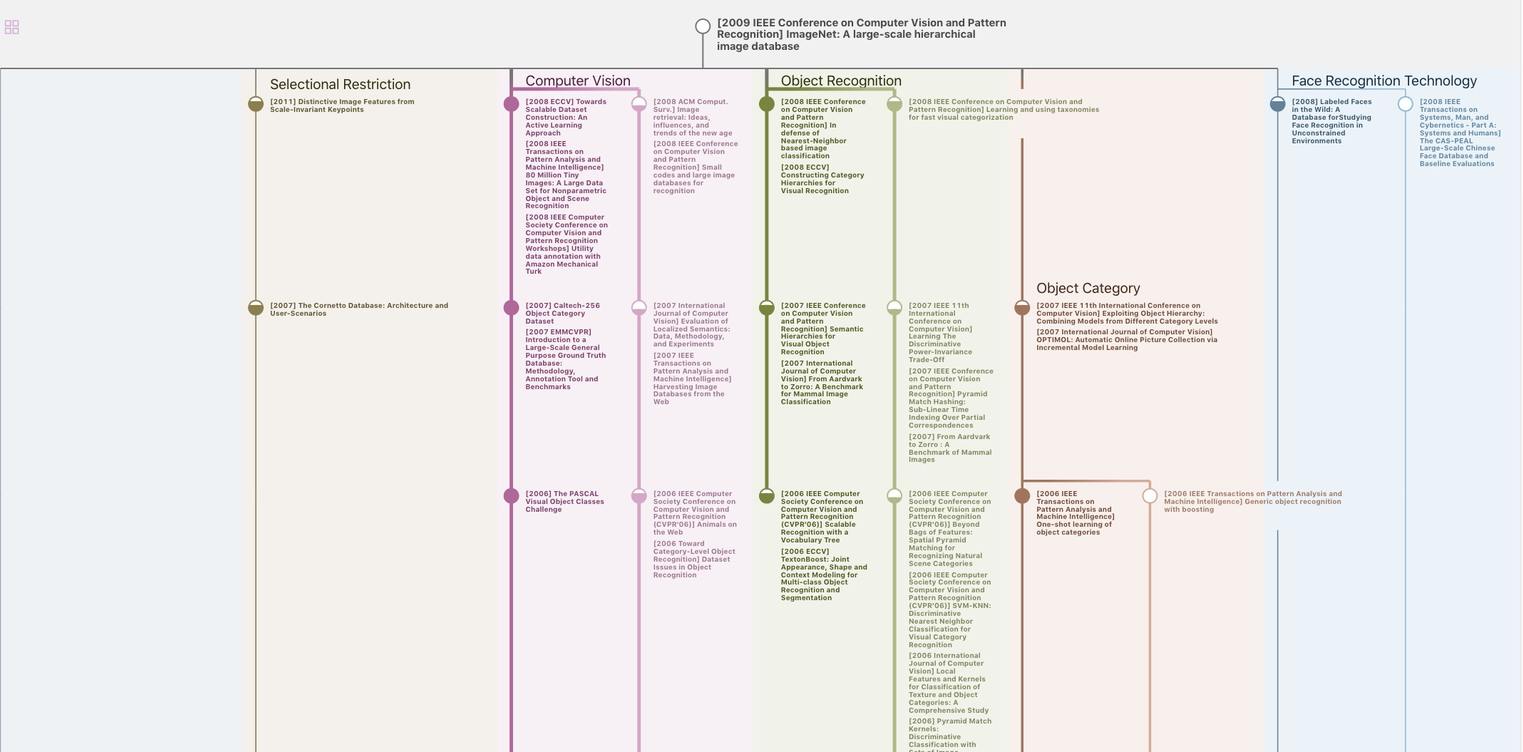
生成溯源树,研究论文发展脉络
Chat Paper
正在生成论文摘要