Towards Fair and Efficient Learning-based Congestion Control
arxiv(2024)
摘要
Recent years have witnessed a plethora of learning-based solutions for
congestion control (CC) that demonstrate better performance over traditional
TCP schemes. However, they fail to provide consistently good convergence
properties, including fairness, fast convergence and stability, due to the mismatch between their objective functions and these
properties. Despite being intuitive, integrating these properties into existing
learning-based CC is challenging, because: 1) their training environments are
designed for the performance optimization of single flow but incapable of
cooperative multi-flow optimization, and 2) there is no directly measurable
metric to represent these properties into the training objective function.
We present Astraea, a new learning-based congestion control that ensures fast
convergence to fairness with stability. At the heart of Astraea is a
multi-agent deep reinforcement learning framework that explicitly optimizes
these convergence properties during the training process by enabling the
learning of interactive policy between multiple competing flows, while
maintaining high performance. We further build a faithful multi-flow
environment that emulates the competing behaviors of concurrent flows,
explicitly expressing convergence properties to enable their optimization
during training. We have fully implemented Astraea and our comprehensive
experiments show that Astraea can quickly converge to fairness point and
exhibit better stability than its counterparts. For example, achieves
near-optimal bandwidth sharing (i.e., fairness) when multiple flows compete for
the same bottleneck, delivers up to 8.4× faster convergence speed and
2.8× smaller throughput deviation, while achieving comparable or even
better performance over prior solutions.
更多查看译文
AI 理解论文
溯源树
样例
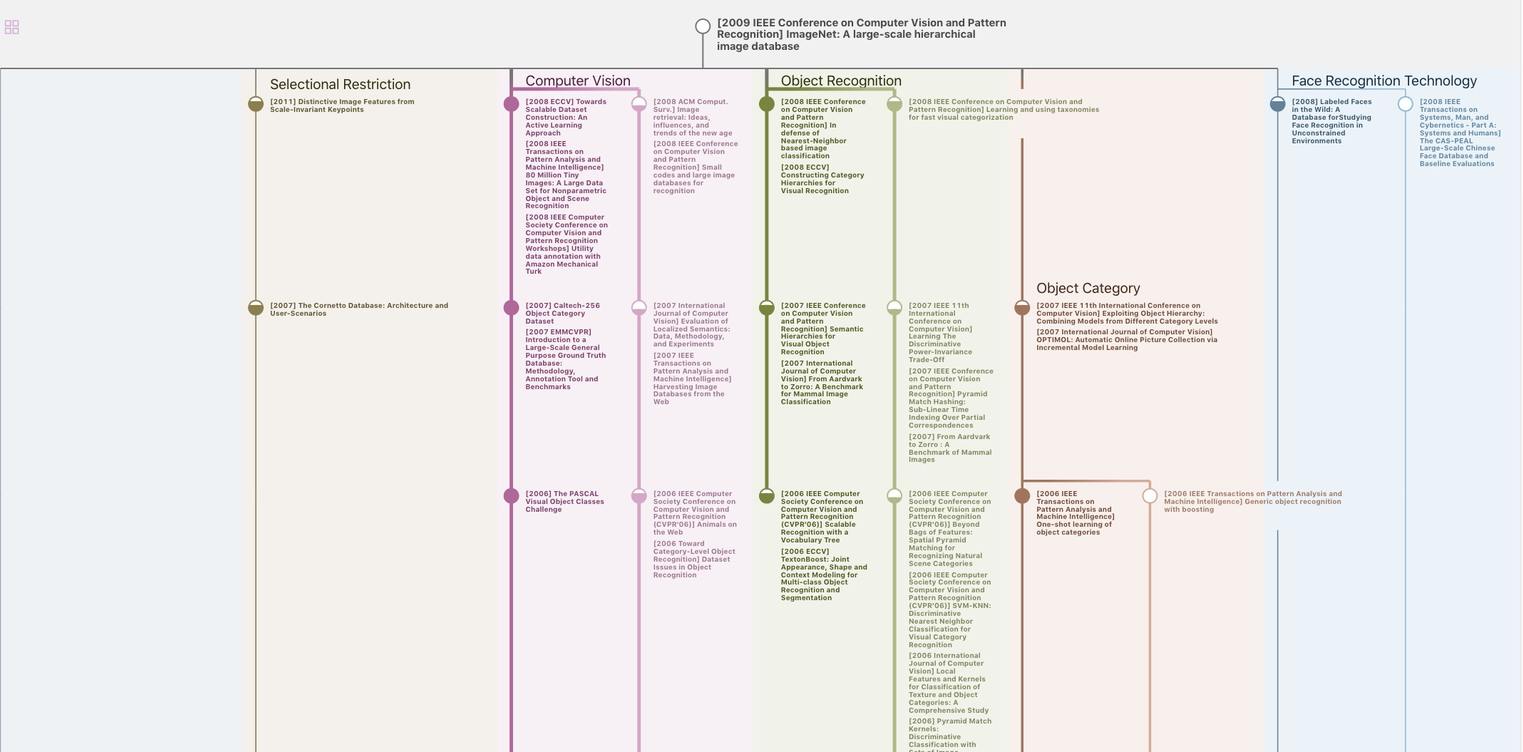
生成溯源树,研究论文发展脉络
Chat Paper
正在生成论文摘要