What Is Missing in Multilingual Visual Reasoning and How to Fix It
CoRR(2024)
Abstract
NLP models today strive for supporting multiple languages and modalities,
improving accessibility for diverse users. In this paper, we evaluate their
multilingual, multimodal capabilities by testing on a visual reasoning task. We
observe that proprietary systems like GPT-4V obtain the best performance on
this task now, but open models lag in comparison. Surprisingly, GPT-4V exhibits
similar performance between English and other languages, indicating the
potential for equitable system development across languages. Our analysis on
model failures reveals three key aspects that make this task challenging:
multilinguality, complex reasoning, and multimodality. To address these
challenges, we propose three targeted interventions including a translate-test
approach to tackle multilinguality, a visual programming approach to break down
complex reasoning, and a novel method that leverages image captioning to
address multimodality. Our interventions achieve the best open performance on
this task in a zero-shot setting, boosting open model LLaVA by 13.4
also minorly improving GPT-4V's performance.
MoreTranslated text
AI Read Science
Must-Reading Tree
Example
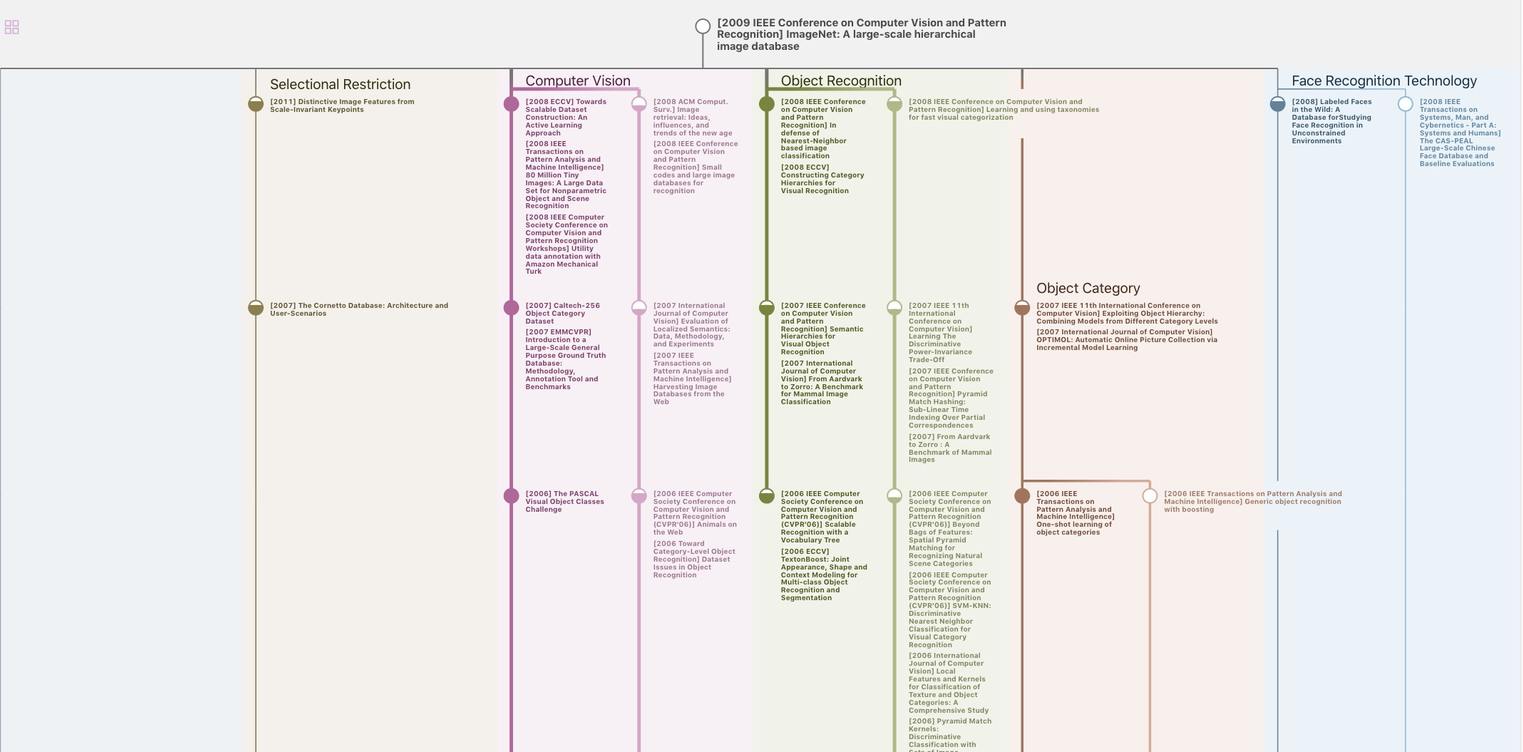
Generate MRT to find the research sequence of this paper
Chat Paper
Summary is being generated by the instructions you defined