Federated Learning Using Coupled Tensor Train Decomposition
arxiv(2024)
摘要
Coupled tensor decomposition (CTD) can extract joint features from multimodal
data in various applications. It can be employed for federated learning
networks with data confidentiality. Federated CTD achieves data privacy
protection by sharing common features and keeping individual features. However,
traditional CTD schemes based on canonical polyadic decomposition (CPD) may
suffer from low computational efficiency and heavy communication costs.
Inspired by the efficient tensor train decomposition, we propose a coupled
tensor train (CTT) decomposition for federated learning. The distributed
coupled multi-way data are decomposed into a series of tensor trains with
shared factors. In this way, we can extract common features of coupled modes
while maintaining the different features of uncoupled modes. Thus the privacy
preservation of information across different network nodes can be ensured. The
proposed CTT approach is instantiated for two fundamental network structures,
namely master-slave and decentralized networks. Experimental results on
synthetic and real datasets demonstrate the superiority of the proposed schemes
over existing methods in terms of both computational efficiency and
communication rounds. In a classification task, experimental results show that
the CTT-based federated learning achieves almost the same accuracy performance
as that of the centralized counterpart.
更多查看译文
AI 理解论文
溯源树
样例
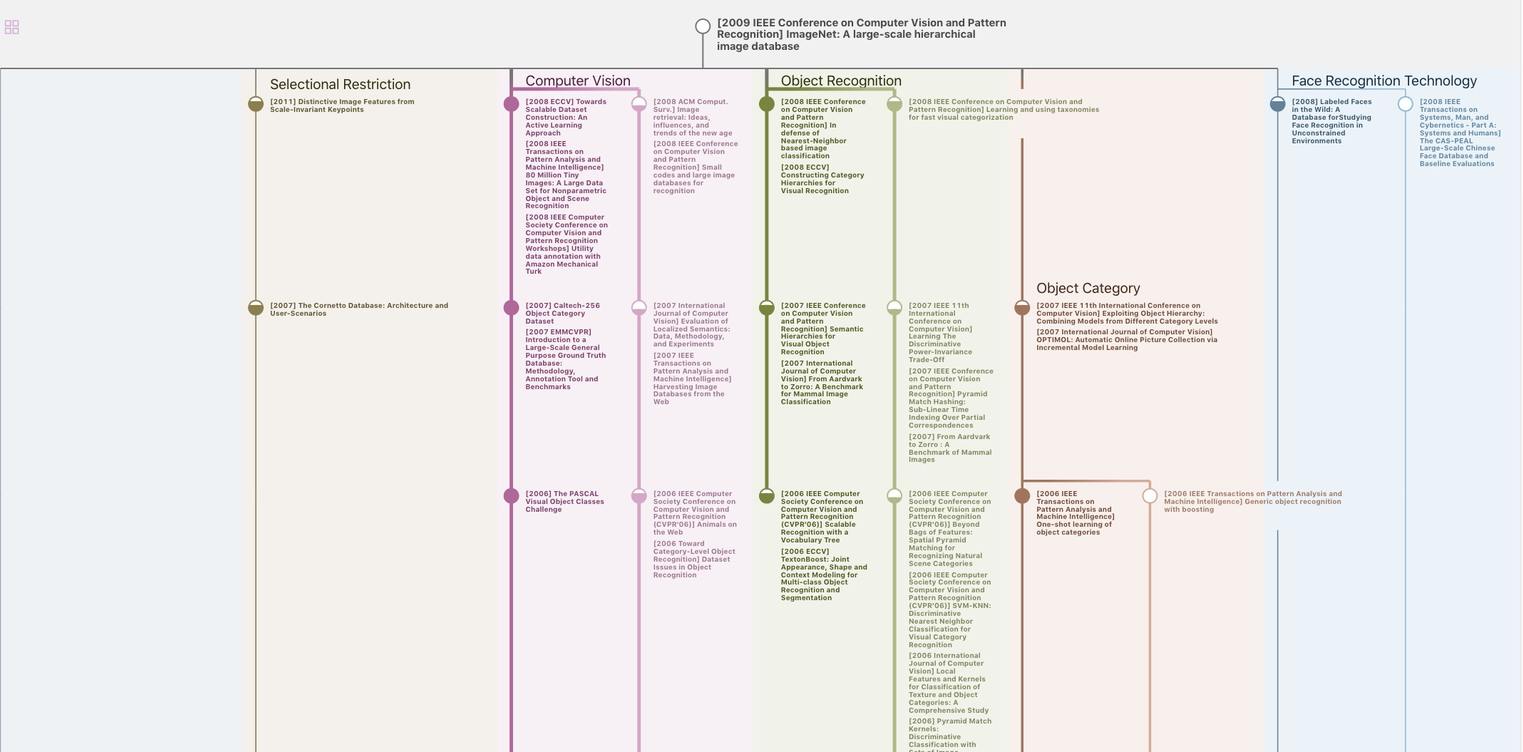
生成溯源树,研究论文发展脉络
Chat Paper
正在生成论文摘要