Addressing Long-Tail Noisy Label Learning Problems: a Two-Stage Solution with Label Refurbishment Considering Label Rarity
arxiv(2024)
摘要
Real-world datasets commonly exhibit noisy labels and class imbalance, such
as long-tailed distributions. While previous research addresses this issue by
differentiating noisy and clean samples, reliance on information from
predictions based on noisy long-tailed data introduces potential errors. To
overcome the limitations of prior works, we introduce an effective two-stage
approach by combining soft-label refurbishing with multi-expert ensemble
learning. In the first stage of robust soft label refurbishing, we acquire
unbiased features through contrastive learning, making preliminary predictions
using a classifier trained with a carefully designed BAlanced Noise-tolerant
Cross-entropy (BANC) loss. In the second stage, our label refurbishment method
is applied to obtain soft labels for multi-expert ensemble learning, providing
a principled solution to the long-tail noisy label problem. Experiments
conducted across multiple benchmarks validate the superiority of our approach,
Label Refurbishment considering Label Rarity (LR^2), achieving remarkable
accuracies of 94.19
long-tail datasets, as well as 77.74
datasets, Food-101N and Animal-10N, surpassing existing state-of-the-art
methods.
更多查看译文
AI 理解论文
溯源树
样例
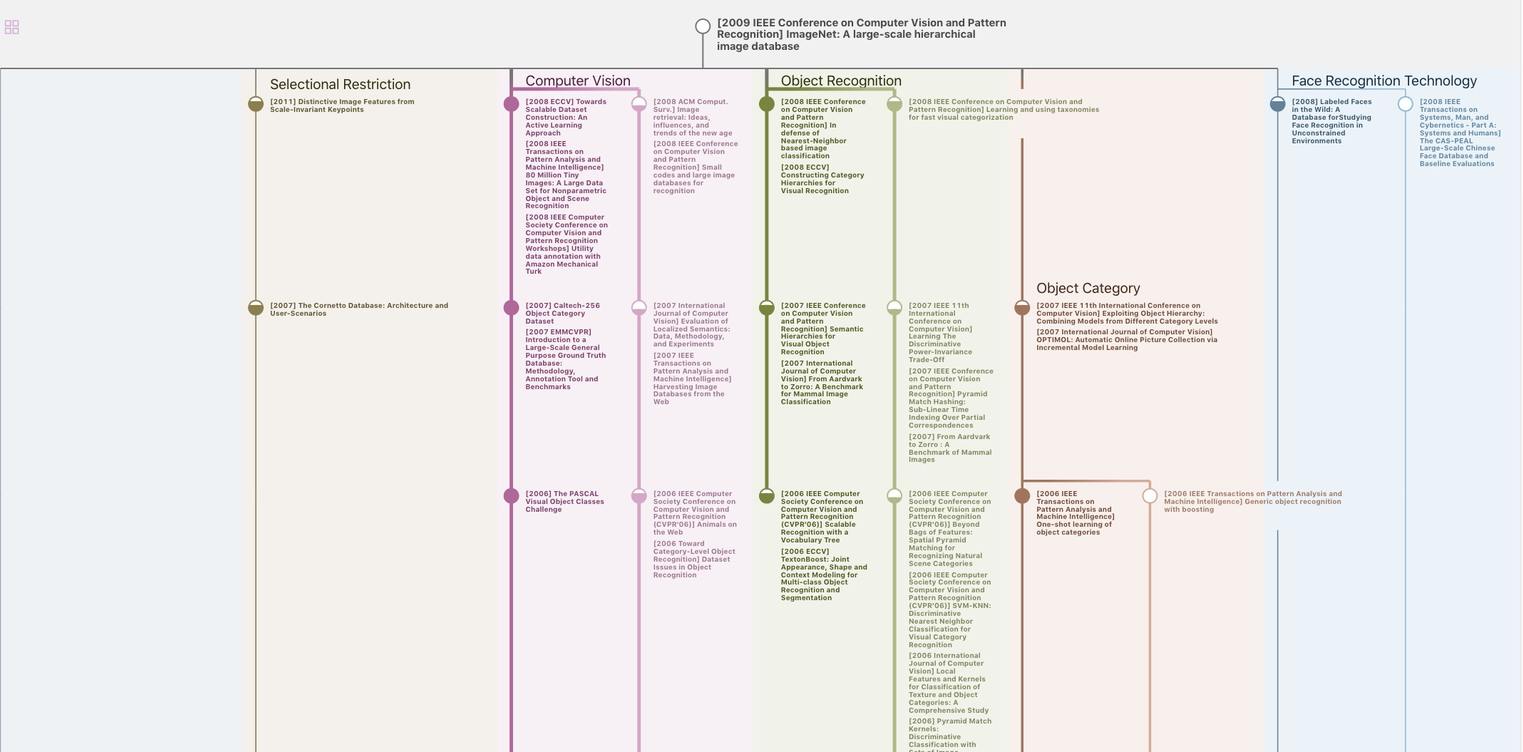
生成溯源树,研究论文发展脉络
Chat Paper
正在生成论文摘要