MUSIC: Accelerated Convergence for Distributed Optimization With Inexact and Exact Methods.
IEEE transactions on neural networks and learning systems(2024)
摘要
Gradient-type distributed optimization methods have blossomed into one of the most important tools for solving a minimization learning task over a networked agent system. However, only one gradient update per iteration makes it difficult to achieve a substantive acceleration of convergence. In this article, we propose an accelerated framework named multiupdates single-combination (MUSIC) allowing each agent to perform multiple local updates and a single combination in each iteration. More importantly, we equip inexact and exact distributed optimization methods into this framework, thereby developing two new algorithms that exhibit accelerated linear convergence and high communication efficiency. Our rigorous convergence analysis reveals the sources of steady-state errors arising from inexact policies and offers effective solutions. Numerical results based on synthetic and real datasets demonstrate both our theoretical motivations and analysis, as well as performance advantages.
更多查看译文
关键词
Convergence acceleration,distributed optimization,gradient descent,machine learning,multiple updates
AI 理解论文
溯源树
样例
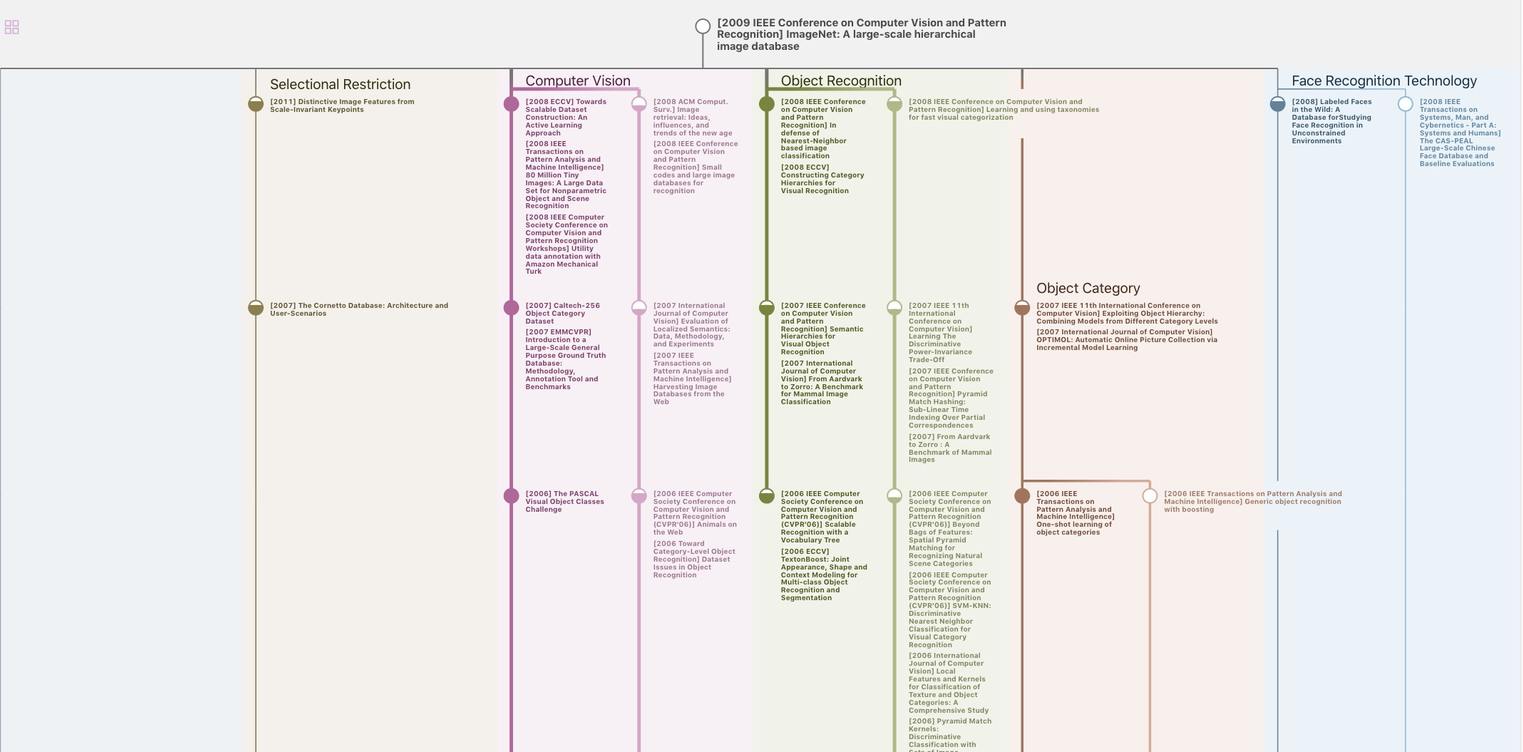
生成溯源树,研究论文发展脉络
Chat Paper
正在生成论文摘要