Constraining primordial non-Gaussianity using neural networks
MONTHLY NOTICES OF THE ROYAL ASTRONOMICAL SOCIETY(2024)
摘要
We present a novel approach to estimate the value of primordial non-Gaussianity (fNL) parameter directly from the cosmic microwave background (CMB) maps using a convolutional neural network (CNN). While traditional methods rely on complex statistical techniques, this study proposes a simpler approach that employs a neural network to estimate fNL. The neural network model is trained on simulated CMB maps with known fNL in range of [-50, 50], and its performance is evaluated using various metrics. The results indicate that the proposed approach can accurately estimate fNL values from CMB maps with a significant reduction in complexity compared to traditional methods. With 500 validation data, the $f<^>{\rm output}_{\rm NL}$ against $f<^>{\rm input}_{\rm NL}$ graph can be fitted as y = ax + b, where $a=0.980<^>{+0.098}_{-0.102}$ and $b=0.277<^>{+0.098}_{-0.101}$, indicating the unbiasedness of the primordial non-Gaussianity estimation. The results suggest that the CNN technique can be widely applied to other cosmological parameter estimation directly from CMB images.
更多查看译文
关键词
cosmic background radiation,cosmological parameters
AI 理解论文
溯源树
样例
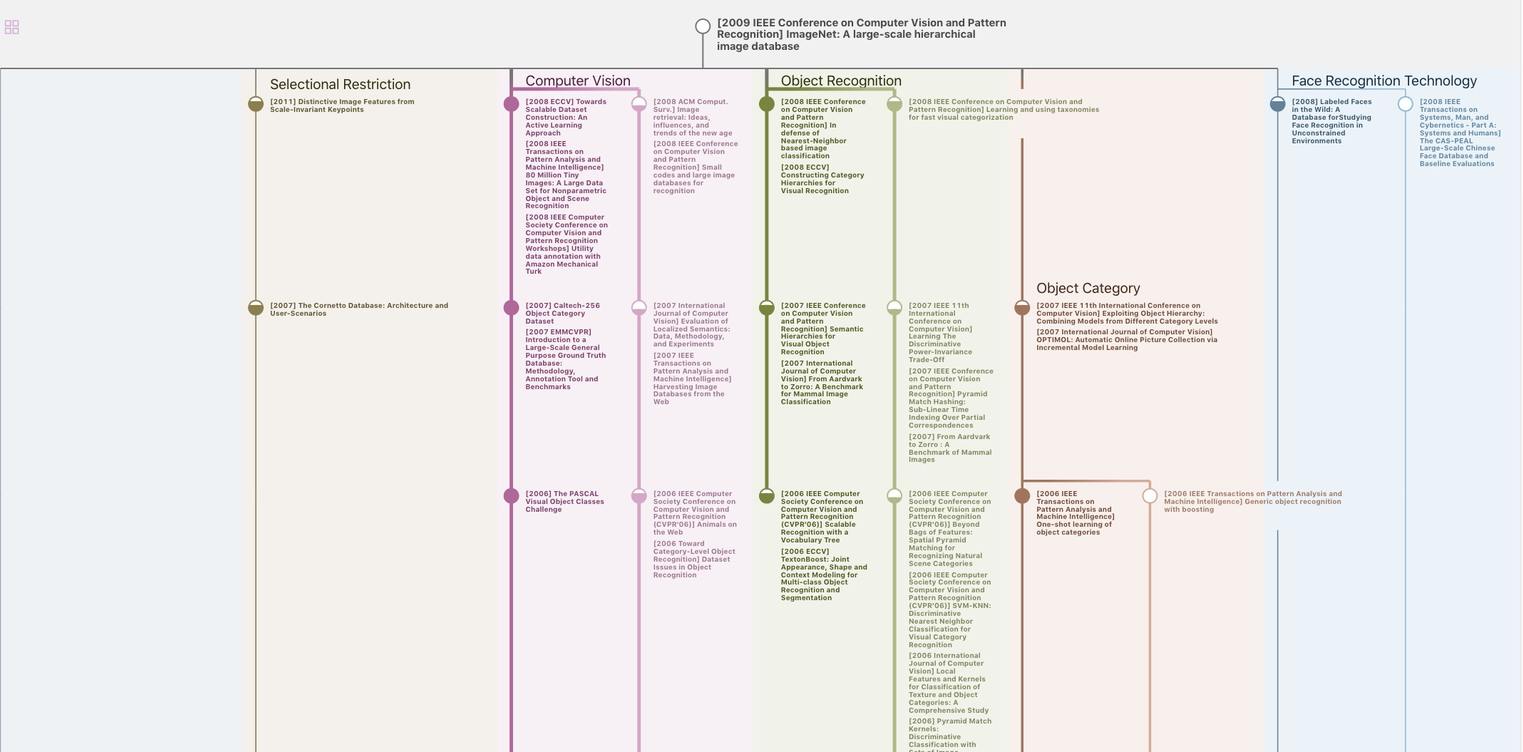
生成溯源树,研究论文发展脉络
Chat Paper
正在生成论文摘要