Predicting Silicate Glass Geochemistry Using Raman Spectroscopy and Supervised Machine Learning: Partial Least Square Applications to Amorphous Raman Spectra
Applied spectroscopy(2024)
Abstract
Here, Raman spectroscopy is used to develop a univariate partial least squares (PLS) calibration capable of quantifying geochemistry in synthetic and natural silicate glass samples. The calibration yields eight oxide-specific models that allow predictions of silicon dioxide (SiO2), sodium oxide (Na2O), potassium oxide (K2O), calcium oxide (CaO), titanium dioxide (TiO2), aluminum oxide (Al2O3), ferrous oxide (FeOT), and magnesium oxide (MgO) (wt%) in glasses spanning a wide range of compositions, while also providing correlation-coefficient matrices that highlight the importance of specific Raman channels in the regression of a particular oxide. The PLS suite is trained on 48 of the 69 total glasses, and tested against 21 validation samples (i.e., held out of training). Trends in root mean square error of calibration (RMSEC), root mean square error of cross-validation (RMSECV), and root mean square error of prediction (RMSEP) model accuracy metrics are investigated to uncover the efficacy of utilizing multivariate analysis for such Raman data and are contextualized against recently produced strategies. The technique yields an average root mean of calibration (similar to 2.4 wt%), cross-validation (similar to 2.9 wt%), prediction (similar to 2.6 wt%), and normalized variance (similar to 28%). Raman band positional shifts are also mapped against underlying chemical variations; with major influences arising primarily as a function of overall oxidation state and silica concentration: via ferric cation (Fe3+)/ferrous cation (Fe2+) ratios and SiO2 (wt%). The algorithm is further validated preliminarily against a separate external set of 11 natural basaltic glasses to unravel the limitations of the synthetic models on natural samples, and to determine the suitability of "universal" Raman-model applications in scenarios where prior chemical contextualization of the target sample is possible. This study represents the first time Raman spectra of amorphous silicates have been paired with PLS, offering a foundation for future improvements utilizing these systems.
MoreTranslated text
Key words
Raman spectroscopy,partial least squares,PLS,supervised machine learning,multivariate analysis,silicate glass,geochemistry,geoscience
AI Read Science
Must-Reading Tree
Example
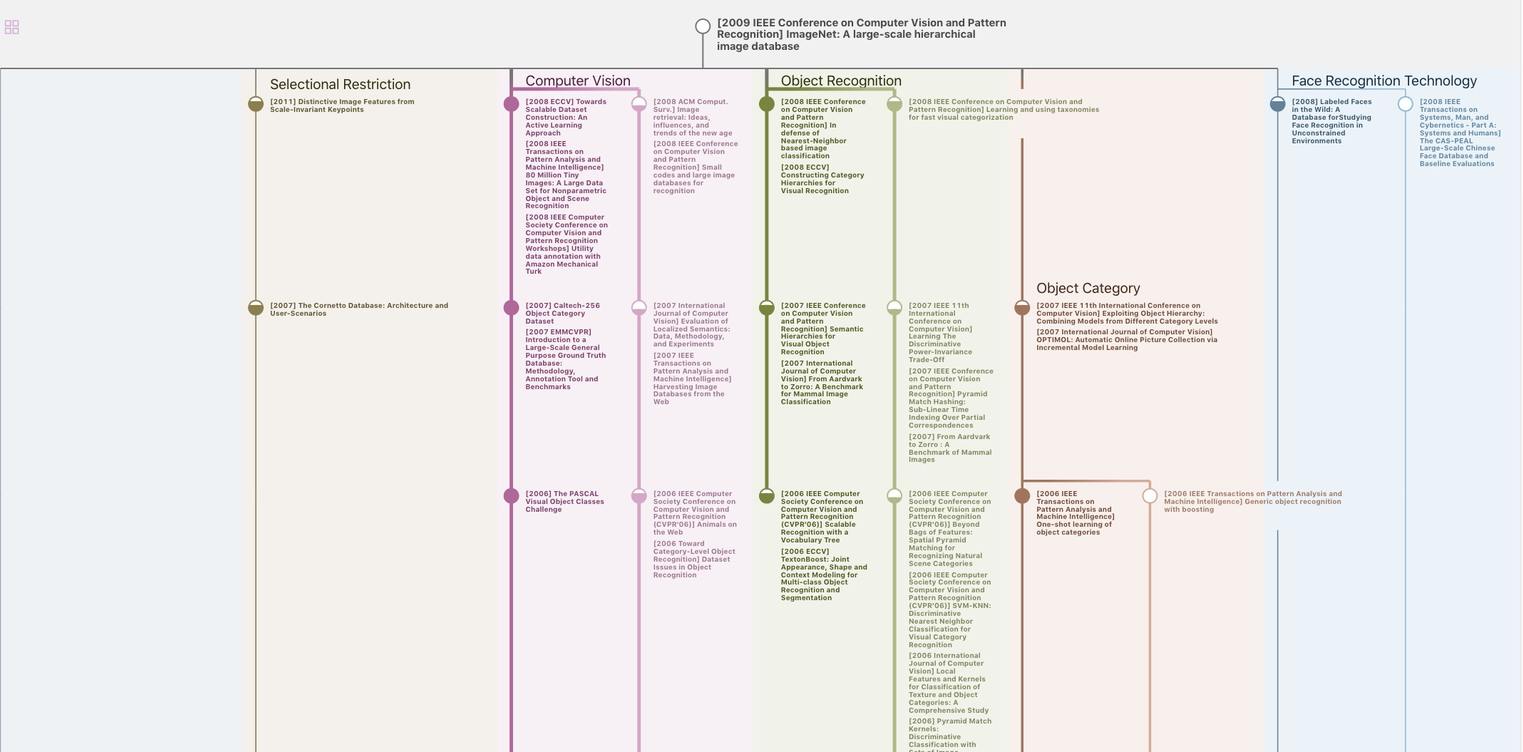
Generate MRT to find the research sequence of this paper
Chat Paper
Summary is being generated by the instructions you defined