Machine Learning Models for Structural Crack Trend Analysis
2023 International Conference on Data Science, Agents & Artificial Intelligence (ICDSAAI)(2023)
摘要
This project aims to create an artificial intelligence (AI)-driven system for crack pattern analysis of structural elements. Cracks in structural elements can cause severe damage and even lead to failure, so early detection and monitoring of cracks is essential to ensure structural safety. However, manual crack detection and monitoring can be time-consuming and prone to human error. To address this issue, a deep learning model ResNet50V2 using the Keras framework, was trained on a dataset of images of cracked and non-cracked structural elements. 81.6% of the test set’s data were accurately predicted by the model, indicating that it can effectively distinguish between images with and without cracks. The model was deployed on a set of 109 images, and the model correctly identified 89 images with cracks, but incorrectly classified 20 images as not having cracks. This indicates a false negative rate and suggests that there is still room for improvement in the model’s performance. In conclusion, the developed machine learning-based crack pattern analysis system has shown promising results in detecting and distinguishing between images with and without cracks. By detecting and tracking cracks in structural elements early on, this technology may prove to be an invaluable instrument in mitigating the likelihood of structural failure or damage. By gathering new data, improving the model architecture, and investigating different methods like data augmentation or fine-tuning the pre-trained model, more advancement can be achieved
更多查看译文
关键词
structural health monitoring,structural crack detection,convolutional neural networks,high-resolution images,real-time,accuracy,infrastructure safety
AI 理解论文
溯源树
样例
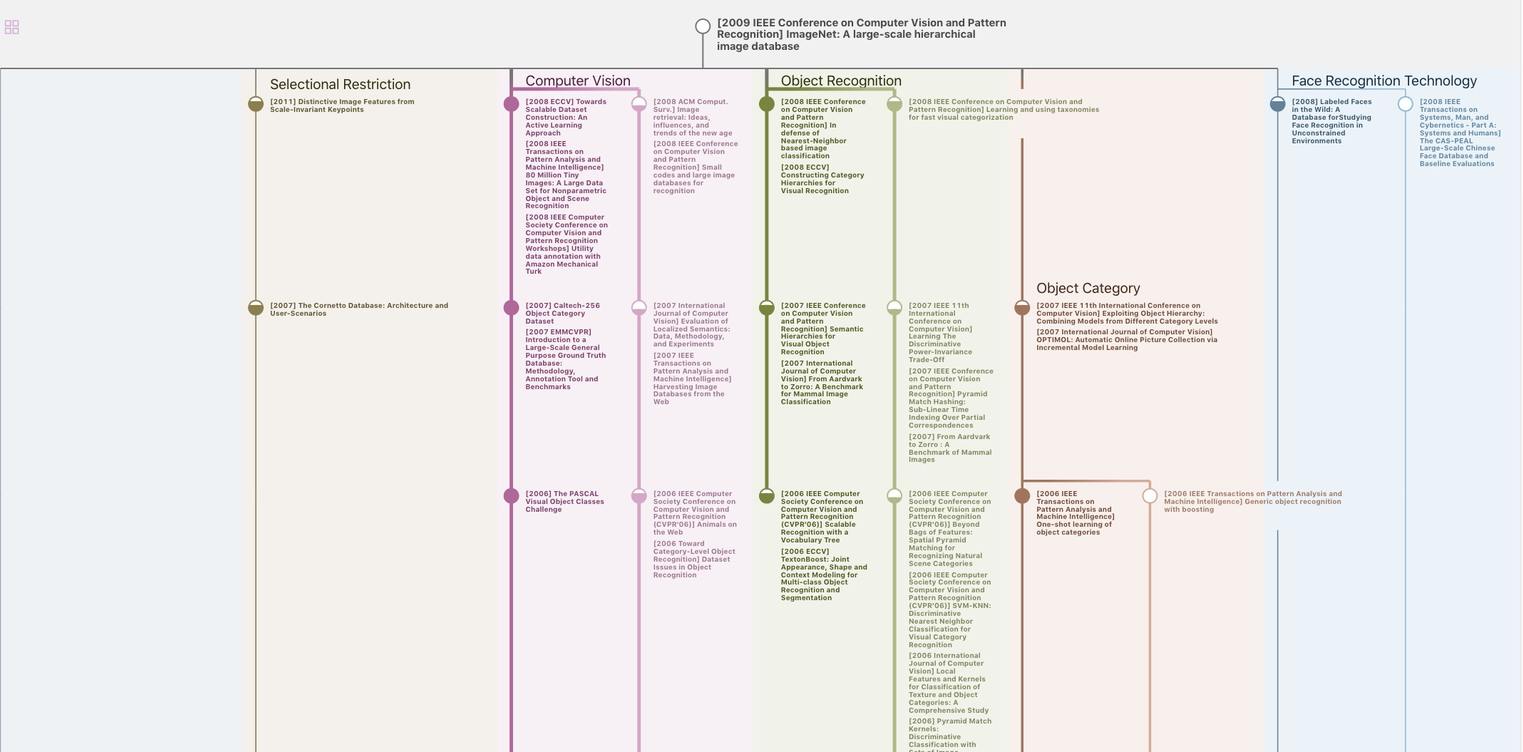
生成溯源树,研究论文发展脉络
Chat Paper
正在生成论文摘要